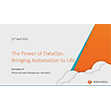
2019: A Big Data Year in Review – Part Two
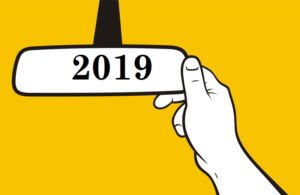
(Jamesbin/Shutterstock)
In part one of this series, we covered some of the biggest news events of the year to cross Datanami’s pages. We pick up where we left off and bring you up to date with the year’s biggest stories through the end of 2019.
Hadoop’s troubles came to a head in the spring, when MapR Technologies warned that it may have to shut down operations as a result of slow sales and Cloudera’s CEO resigned following poor first-quarter results. MapR would go on to be acquired in a fire sale by HPE in August, while Cloudera would soldier on. Cloudera’s stock (NYSE: CLDR) has recovered from the drop this spring, but it’s still 50% below its all-time high.
Last winter was unusually cold in the Bay Area, and the surrounding hills were dusted with snow on at least two occasions. The cold streak continued into June, when Snowflake’s new CEO, Industry veteran Frank Slootman, made it snow several times on stage at Snowflake’s inaugural user conference. The long-term forecast for big data: snowy.
Down in San Diego, data warehouse giant Teradata was itching for a fight during a boisterous 40th birthday celebration at company headquarters. New CEO Oliver Ratzesberger declared that the company was not going to take punches anymore and “go on the offensive.” But by November, Ratzesberger would be replaced in the CEO’s seat by Vic Lund, whom he had replaced just 11 months earlier.
Despite great progress made during human’s reign on this planet, our understanding of the world is limited. But thanks to the power of machine learning, we’re making progress in understanding how complex chemicals interact with our bodies and how we can stop the climate from changing.
We’re firmly into the new AI era, with talking toasters and cars with supercomputers in them. Why, then, are we spending so much time on remedial data management tasks like it’s the 1980s? The answer may surprise: big data is still hard. And guess what? It’s not going to get any easier, particularly with new regulations, privacy, and governance requirements coming down the pike. Time to take the red pill and deal with data’s unfortunate reality.
There are several hurdles separating us from our glorious AI future, and one of them is ethics. Unfortunately, we have barely begun to deal with the ethical concerns of data, let alone the ethical implications of AI. We talked to industry experts and came up with the start of a game plan for beginning to address this topic.
Cloud-based object stores that look like they’re on-prem. On-prem data stores that behave like they’re in the cloud. What in the world is going on? Welcome to 2019 – and it’s just going to get more fun.
Python had another breakout year in 2019, with some rankings saying it’s the number one programming language in the world. But is Python’s undeniable popularity coming at the expense of R? There’s some evidence that says it is, while other experts say reports of R’s demise are greatly exaggerated.
Hadoop was knocked down a peg or three in 2019, and public cloud platforms were there to scoop up disaffected Hadoop users. To understand what just happened, we convened with industry experts to explain how Hadoop fell so hard, what was wrong with the Hadoop view of the data world, and where big data management goes from here.
It’s 2019. The drought in the Western United States is over. War deaths around the world are at a 500 year low. The Chicago Cubs and Boston Red Sox have vanquished their World Series curses. Why, then, are we still doing ETL with big data projects? The facts will probably dismay you.
Among cloud platforms, Google Cloud Platform is the smallest. But the folks at Google are standing tall when it comes to making meaningful contributions to open source big data projects, including integrating Kubernetes into various components of the big data stack, including Hadoop.
Cloudera began its new “cloud era” at the Strata Data Conference in New York City, where it launched a new flagship platform, the CDP, which is a cloud-native version of Hadoop that runs Kubernetes instead of YARN and relies on S3-compliant data stores instead of HDFS. Next up: Introducing an on-prem version, planned for 2020.
Since its creation, Apache Kafka has been the un-database. It’s been the thing you need to move and act upon large amounts of event data, which databases have historically been bad at. But managing state in Kafka has been a tricky thing, and so at the Kafka Summit in October, Jay Kreps revealed plans to turn KSQL into an “event streaming database,” which was released as kqlDB the following month. Some things will never change – including, apparently, our reliance upon the database construct.
The vectorization of data enables neural network methods to glean insights from vast amounts of data. However, not every type of data lends itself to vectorization, which is why images and words have been the leading targets for deep learning. But now researchers are pushing the limits in creating “thought vectors,” or higher order collections of words that can be processed with neural networks. According to Geoff Hinton, the father of deep learning, it’s just a matter of bringing enough hardware to bear on the problem.
Storage-class memory promises to dramatically speed up data processing tasks, but its delivery has been relatively slow via Intel. In October, Intel’s one-time partner in storage-class memory, Micron, delivered its first 3D XPoint technology product, the X100, which it claims is the fastest SSD drive in the world.
Deep learning is the engine upon which our AI hopes are built. All the self-driving cars and “smart” devices that we are counting on to make our lives better in the future are dependent upon this technology. But there’s a big problem: Deep learning has hit a wall, according to Naveen Rao, the general manager of Intel’s AI division.
Taxes. A slowing metabolism. The influence of money in college football. Some things just aren’t worth fighting. Here’s another one to add to the list: The victory of the data nerds. If you think you can control the data exploration activities of all your employees and confine them to traditional BI workflows, Looker’s chief product officer Nick Caldwell has a piece of advice for you: Don’t.
Data regulations are coming, whether you like them or not. But how will the California Consumer Protection Act (CCPA) impact your business? The folks at Immuta helped us sort fact from fiction when it comes to conducting analytics in the CCPA era.
If history is any guide, private industry settles upon two to three standards for every product category. When it comes to building AI applications, the race is on to develop the first enterprise AI platform. Will Databricks be one of them? CEO Ali Ghodsi certainly hopes so.
AWS gave customers 28 more reasons to use its cloud to store and process data at its re:Invent conference in December. Among the new AWS offerings is a new Cassandra database service, various improvements to Redshift, and enhancements to SageMakger, it’s automated ML offering.
Deep learning is the future of machine learning. But explaining how complex neural networks work is exceptionally difficult. Now Google and a firm called ZestAI say they’ve made real progress in the explainable AI problem. The key to the solution, apparently, is a single algorithm from collaborate game theory developed decades ago called integrated gradients.
One of the qualities that separates “big data” from regular IT is the need for distributed processing. However, developing applications that run in a distributed manner is still too hard. That’s the challenge being tackled by Ray, a new computing framework for Python coming out of the RISELab. And in December, the company Anyscale launched with nearly $21 million in funding to scale Ray.
Related Items:
2019: A Big Data Year in Review – Part One
What’s Up with 2019? Big Data Predictions
Predictions for Application Development in 2019