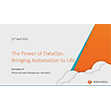
The ‘Big Bang’ of Data Science and ML Tools
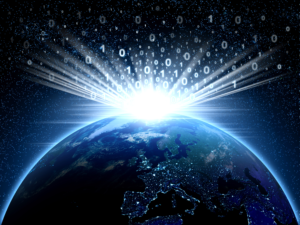
The tools used for data science are rapidly changing at the moment, according to Gartner, which said we’re in the midst of a “big bang” in its latest report on data science and machine learning platforms.
“The data science and ML market is healthy and vibrant, with a broad mix of vendors offering a range of capabilities,” Gartner says in its Magic Quadrant for Data Science and Machine Learning Platforms published January 28. “The market is experiencing a ‘big bang’ that is redefining not only who does data science and ML, but how it is done.”
The analyst group defines a data science platform as an integrated place where data scientists, citizen data scientists, and developers can get all of the core capabilities that they need to not only build data science application, but to embed them into existing business processes and manage and maintain them over time.
Data science and ML platforms must meet minimum requirements, and include tools for
- ingesting and preparing data;
- interactively exploring and visualizing data;
- engineering data features and building predictive models;
- testing and deploying those models in an integrated fashion with surrounding infrastructure.
Integration and cohesion are keys, in Gartner’s view, and applications that simply bundle various packages and libraries – especially open source offerings — are not considered true platforms.
While these core requirements set the stage for data science and ML platforms, there are big differences in how the various suppliers get there. Gartner notes that expert data scientists may prefer writing code in Python or R, while others like the ease of use of data science notebooks, such as Jupyter. Still other less technical folks prefer more intuitive point and click interfaces.
Leader’s Quadrant
Gartner placed four vendors in the Leader’s Quadrant, including KNIME, RapidMiner, TIBCO Software, and SAS.
KNIME ranked highly in Gartner’s assessment as a result of strong support from customers, a broad product set, and having “one of the most balanced” visions in the market. The Zurich company’s product lineup – which consists of the open source KNIME Analytics offering and the commercial KNIME Server product — were lauded as the “Swiss Army Knife” of analytics. Support for advanced features like deep learning, ease of use by intermediate users, and integration with other packages were lauded. However, performance and scalability were seen as weaknesses, as well as limited traction in IoT.
Rapid Miner also ranked highly in the leader’s quadrant thanks to its balance between ease of use and supporting sophisticated data science capabilities. The software supports deep learning technology and deploys to GPUs, and Gartner seemed to like how Rapid Miner’s delivers more transparency for machine learning deployments. Its integration with open source tools will be beneficial to data scientists, it says. The main concerns are around data prep and visualization; licensing and pricing; and model operationalization.
TIBCO made a big move up from the Challenger’s Quadrant by purchasing a range of analytics properties, including Jaspersoft, Spotfire, Statistica, and Alpine Data, and integrating them into a single cohesive platform. Gartner liked the end-to-end workflow integration that TIBCO delivers, and its IoT capabilities – particularly with the integration of streaming analytics. Potential concerns include performance and stability, data management, and questions around operationalization.
SAS is a perennial contender on this list, and in fact has multiple platforms that were assessed. Its Enterprise Miner offering delivers strong, reliable performance across a range of metrics, while Visual Data Mining and Machine Learning (VDMML) had high scores for data prep and augmentation. High customer satisfaction levels and strong market presence bolster SAS’s position as a leader. But Gartner also listed some downsides of SAS’s approach, particularly around pricing and product coherence. The SAS EM user experience hasn’t kept up with expectations, and SAS’ approach to open source is a question mark for Gartner.
Challenger’s Quadrant
The Challenger’s Quadrant was fairly empty, with just Alteryx and Dataiku occupying that space.
Alteryx dropped from the Leader’s Quadrant by maintaining its “ability to execute” (the Y axis) but losing some of its “completeness of vision” (the X axis). Gartner heralded the Irvin, California company’s citizen data science capabilities within an end-to-end pipeline. Despite its capabilities, the market perceives Alteryx as just a data preparation tool, which obscures its value, the analyst group says.
Dataiku‘s Data Science Studio (DSS) offering received high marks for the way it fosters collaboration among different stakeholders, from data engineers to scientists. Gartner also liked the automation it brings to the machine learning workflow, as well as the management and monitoring of models once they’re in production. Some concerns include scalability, pricing, and support for streaming analytics and IoT use cases, it says.
Visionaries Quadrant
The Visionaries Quadrant was crowded, with new fewer than seven vendors jockeying for position.
Databricks, which inked $250 million in venture funding this week, impressed Gartner with its support for the full analytics life cycle, its support for hybrid cloud strategies, and its capability to support a variety of users. Users spoke highly of the Spark-based cloud offering, and documentation was a plus, per Gartner. Pricing and contract negotiations were potential weak spots for Databricks, along with monitoring, management, and troubleshooting and debugging potential problems.
DataRobot debuted on the quadrant in the Visionaries, thanks to the fact that it “sets the standard for augmented data science and ML,” Gartner says. Customers enjoy a “strong experience,” which is helping the company to gain traction with an already solid installed base. Sales execution, pricing, scalability concerns, and the possible commoditization of the “augmented analytics” space are cocerns.
H2O.ai, which held its H2O World conference this week, dropped from the Leader’s Quadrant in 2019 into the Visionaries Quadrant as a result of strong competition, and some concerns from customers about capabilities. The performance of its core open source machine learning components remain a strength for H2O.ai, and Gartner was impressed with its GPU-based deep learning and the automated ML capabilities of Driverless AI. But a steep learning curve for non-developers, a lack of management capabilities, and a lack of data access and data prep features were concerns.
MathWorks made a huge lateral move, from the Challengers to the Visionaries Quadrant, thanks to “a remarkable strength” in serving the demands of its customers in asset-centric industries, according to Gartner (the company has a long heritage among manufacturers and engineering organizations). Its MATLAB offering was hailed for its “citizen engineer” capabilities, and integrated data prep and support for real-time streaming, deep learning, and simulation impressed the G man. Dings were difficulty of use by non-engineers, no support for Google Cloud Platform, and a lack of automated machine learning capabilities were downsides.
Microsoft scored well with its cloud-based offerings, which include Azure Machine Learning, Azure Data Factory, Azure HDInsight, Azure Databricks, and Power BI. Gartner liked how Microsoft works with third-parties, in particular Databricks’ Spark offering. Support for diverse data personas, including entry-level ML enthusiasts, was also a plus. Automation in the ML process was a concern, as was the coherence of all the different tools. A lack of on-prem capabilities also limits its applicability.
IBM stays in the Visionaries Quadrant for 2019, but it has lost ground. Gartner praised the comprehensive nature of IBM’s Watson Studio offering, which serves expert and citizen data scientists. Integration of the SPSS modeler into Watson Studio was also praised. But the frequency that IBM rebrands products and shifts strategy is a concern to Gartner, as is the need to license multiple products to get complete end-to-end capabilities.
Google did pretty well in the data science and ML platform ranking, thanks largely to the wide breadth of tools available on its cloud. Its core data science platform consists of Cloud ML Engine, Cloud AutoML, TensorFlow, and BigQuery ML. But Google also offers unique hardware, with the Tensor Processing Unit (TPU), crowdsourcing with Kaggle, and a range of other offerings. Scalability and speed are strengths. But a lack of end-to-end cohesion among the tools was a concern, as well as a lack of reusability. The lack of an on-prem offering was also a concern.
Niche Players Quadrant
Four vendors found themselves in the Niche Players Quadrant.
SAP’s Predictive Analytics (PA) offering is tightly integrated with HANA, which makes it suitable for SAP HANA customers. The capability to process large HANA datasets and deploy models to SAP applications are strengths. So is SAP’s vision of a unified ML fabric, which is tied to its Leonardo Machine Learning Foundation. However, product coherence, a changing AI strategy, and the customer experience were marks against the German giant.
Domino Data Lab was downgraded from the Visionaries Quadrant, which reflected mostly a drop in its perceived ability to execute. Gartner likes Domino’s product strategy, in particular its focus on collaboration and building an end-to-end solution. Its ability to integrate with open source and proprietary products was a bonus, as was its scalability. But Domino’s focus on expert data scientists leaves citizen data scientists wanting, according to Gartner, and it also lacks some data prep, automation, and augmentation capabilities.
Anaconda remained in the Niche Players category. Key strength of the Anaconda product is its reach into the open source Python community, which continues to churn out data science innovation. Its capability to scale open source Python is also a plus. But the expertise needed to successfully wield the Anaconda platform is a caution, per Gartner, and the complexity of the Python “jungle” is also a concern. Reliance on the open source community also puts customers at a disadvantage when they need something specific (Gartner uses the example of model operationalization), and the overall level of coherence is a downside.
Datawatch is a newcomer to this Magic Quadrant by way of its January 2018 acquisition of Angoss, which has more than 20 years of experience in the field. Gartner praised the coherence and ease of use of the Datawatch products, and marked the text analytics and optimization engine components as above average. Customer support was also a plus. A lack of data preparation capabilities dragged Datawatch’s score down, while the overall vision of the product and uncertainties raised by the acquisition were also mentioned.
Related Items:
What Gartner Sees In Analytic Hubs
Winners and Losers from Gartner’s Data Science and ML Platform Report