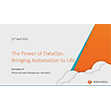
MapR Takes Aim at DataOps with Platform 6.0
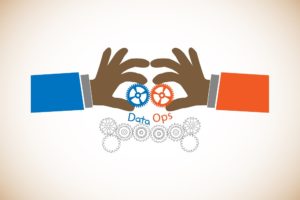
MapR today unveiled a new release of its converged data platform that’s aimed at helping companies use emerging DataOps concepts to go from data science insight to positive business result in a speedy, reliable, and secure manner.
The term DataOps has recently been used to refer to the overall operationalization of data science, and it’s one that MapR has found to its liking, particularly in light of the new features the company just delivered with Version 6.0 of the MapR Platform.
There’s something for everybody involved in the DataOps cycle — including the data scientists, data engineers, systems administrators, and cluster operators — in version 6.0.
- Operations folks will appreciate the new MapR Control System (MCS), which gives them a single pane of glass to monitor the health of the cluster and manage all the data stored in the MapR platform, including files in the MapR File System, JSON-like tables stored in MapR-DB, and streaming data stored in MapR Streams.
- Admins will appreciate the new security features in MapR Platform 6.0, including the capability to implement encryption and to enforce strong authentication on the cluster with just a few clicks of the mouse.
- Data scientists will like the new Data Science Refinery, which gives them secured access to all the data stored in the cluster (including files, tables, and streams) as well as a built-in Apache Zeppelin data science notebook environment for experimenting with machine learning models. Hooks to more powerful data science systems from Data Science.com, H2O, and C3 IoT are also provided.
- Data engineers will appreciate the new changed data capture (CDC) capabilities built into the platform that automatically propagates data persisted in MapR-DB out to MapR Streams via a microservices API so that the freshest data can be consumed by data scientists who are iterating with their machine learning models, eliminating the need for batch data transfers.
These features help to grease the wheels to achieving success with data science work, according to MapR Senior Technologist Mitesh Shah.
‘Everybody wants to turn data into value, ideally greater value and ideally in a shorter time,” he says. “But there’s lots of sources of friction along the way that impede that progress, impede that data-to-dollar cycle, if you will.”
Some of the sources of friction are technology oriented, while others are process and people oriented. Having security configurations properly set up is important, because data science can’t occur if the cluster is susceptible to being breached, he says. Just getting the data from its source to a place where it can be experimented upon by data scientists is another source of friction that MapR.
Connecting everybody involved in the DataOps process together can also help to eliminate barriers to productivity. That speaks to the speed and agility that’s required to achieve success with data science in the real world, Shah tells Datanami.
“From a process perspective, organizations are still a little bit in that waterfall model,” he says. “As they work with their IT department, they’re taking months, and in some cases years, to plan ahead and build out their system and tap into their data. But by then perhaps the market moved, perhaps the data is stale and you’re out of luck.”
Just as the broader IT world has embraced the concept of DevOps, which uses new technologies and processes to brings application developers and operations folks together in a cohesive and mutually beneficial manner, the data world today is moving toward DataOps.
“The analogy is DevOps is to agile application development and release what DataOps is to the agile process of turning data into value,” Shah says. “We’re now extending that [DevOps] team to include data engineer and data scientists, where instead of just releasing production grade applications, they’re now tasked with turning data into value.”
Related Items:
MapR-DB Gets Secondary Indexes to Drive Operational Analytics
MapR Rebrands Around Converged Data Fabric
MapR Extends Its Platform to the Edge