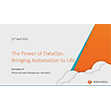
Data Science Platforms Seen as Difference-Makers
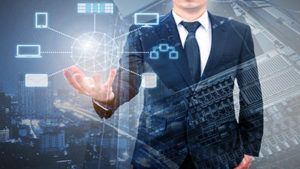
(Joe Techapanupreeda/Shutterstock)
How will data scientists work in the future? Based on today’s trends and a new survey by Forrester, it seems likely that much of the work that data scientists do will revolve around centralized platforms that help to organize not just the data and the tools, but data scientists themselves.
The idea of a data science platform is not exactly new in the big data community, but neither is it widespread. A handful of companies in the space are beginning to advertise their wares as “data science platforms” that can centralize the tools and processes needed to integrate and explore data, develop and deploy advanced analytic models, and to streamline communication and collaboration among principals—all while adhering to security and governance principles.
One of these vendors, the aptly named Los Angeles-based firm DataScience, recently teamed up with Forrester to explore the use of big data analytics and data science platforms in greater depth. In particular, Forrester sought to quantify the impact that data science platforms have on the organizations that use them, and whether the use of more advanced and centralized platforms translates into better business results.
The survey found a strong correlation between investments in data science and better business results, which should come as no surprise. That is, after all, the whole point. While 99% of the 208 people who participated in the study agreed that data science is important to their companies, not all of the companies were equal in their capability to squeeze business advantages out of their data (which also should come as no surprise).
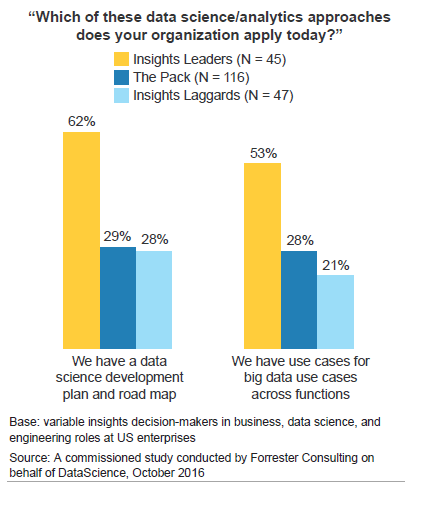
Source: Forrester Consulting report: “Data Science Platforms Help Companies Turn Data Into Business Value.”
Based on the answers they gave and analysis of the results, Forrester categorized its survey-takers into one of three buckets. The top 22% of the companies were termed “Insights Leaders,” while the bottom 23% were the “Insights Laggards.” In the middle is The Pack.
Forrester found Insights Leaders have data science budgets that are twice as big as Insights Laggards, and 1.5 times as big as The Pack. Leaders are also more likely to have a well-thought-out data science development plan and roadmap compared to the others, and to use big data across different functions. They also have more automated management and deployment of algorithms and APIs than their brethren, the report found.
In terms of business results, it found Leaders are four times more likely than Laggards to have revenue growth that exceeds the expectations of shareholders. Leaders are also twice as likely to see profit exceed expectations than Laggards. Interestingly, the Laggards tended to be smaller firms, while Laggards tended to be bigger firms.
While only 26% of firms have invested in a single data science platform to manage their data science work, the Forrester study found that Leaders were nearly twice as likely to have already implemented a data science platform or to be planning to deploy one in the next two years (85% for Leaders, 47% for Laggards).
Connective Tissue
When you look at the results as a whole, it shows a need for “connective tissue” to fill in operational gaps in data science projects, says Ian Swanson, founder and CEO of DataScience, which commissioned Forrester to perform the survey.
“A lot of them have been spending massive amounts of time and money and resources building data infrastructure, but they’re not yet doing data science work and actually applying models from that data infrastructure,” Swanson tells Datanami. “When we step back, our view is that the last wave was investment in Hadoop and big data infrastructure. And this next wave is about hot to value out of all that infrastructure and data that’s now available, and we think that data science platforms are an important piece of customers being able to do that.”
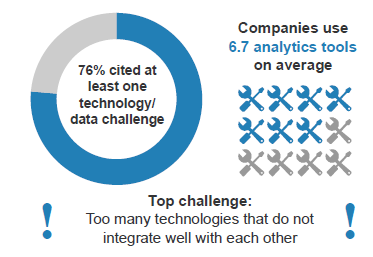
Source: Forrester Consulting report: “Data Science Platforms Help Companies Turn Data Into Business Value.”
To be sure, Data Science isn’t the only vendor talking about this thing called a data science platform. Other data science firms using that phrase to refer to all-inclusive platforms for data science work include Continuum Analytics, H2O, Domino Data Lab, and Wolfram. And we’d be remiss if we didn’t include analytics giant SAS in that vein, as well as IBM (with SPSS) and Matlab developer Mathworks. Rapid Miner, KNIME, and Alteryx also come to mind, and surely there are others.
In Swanson’s view, the there’s an important distinction between advanced analytic and statistical tools on the one hand, and a data science platform in the other. An enterprise data science platform helps data scientists get the most value out of their data by managing the big data analytics lifecycle and standardizing routine processes while enforcing security and governance.
“It’s reducing the sprawl of tools that they have internally,” Swanson says. “It’s allowing them to do things like reuse work that teams are doing, to share knowledge across teams, and to accelerate what they’re doing in terms of applying the models they develop in their actual business. It’s closing that gap between developing interesting models and insights and actually applying it to the business.”
Managing the Workflow
In the Wild West world of big data, data scientists were free to use the tools they want in whatever manner works for them. But that anything-goes mentality is changing now that the well-dressed men and women in the C-suite are giving big data projects more notice and more money.
While we’re not likely to see a repeat of the rigid software change management (SCM) environments that enterprise IT have been laboring under since the passage of the Sarbanes-Oxley Act so many years ago, there is a definite trend to rein in some of the more wild and wily aspects of one-off data science projects, and toward more management and reproducibility.
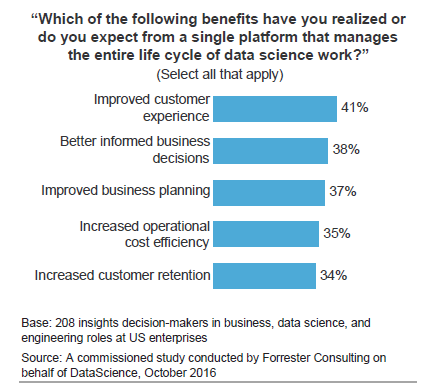
Source: Forrester Consulting report: “Data Science Platforms Help Companies Turn Data Into Business Value.”
A critical step to gaining reproducibility in data science is defining all the different steps involved, from ingesting the first piece of data to deploying a model in production. A data science platform will help to create and manage the workflows involving those steps, says DataScience Chief Strategy Officer William Merchan.
“Think of all those steps that go into developing a recommendation engine or a targeting algorithm or a lifetime-value model,” Merchan says. “Those all need to go through that process, and you need to have a process that’s consistent across the team that people can collaborate with, that has the infrastructure behind it that can scale. Those are all the pieces that need to come together.”
DataScience, which has attracted more than $37 million in funding, is primarily a services firm. The company developed its data science platform primarily to help its data scientists in customer engagements, and only later decided to productize it.
Some of the common challenges DataScience employees have faced is how to integrate models developed in R or Python with business applications written in Java. In that respect, data science platforms go beyond the math and statistics, and touch on aspects of enterprise application architectures, as well.
Swanson sees one other important aspect of data science platforms. Many of the data science tools that are popular today are free and open source, and Swanson says that’s translating into customer demand for data science tools that are open and flexible.
“We’re seeing this shift away from closed, legacy systems to leveraging the power of open source, but still making sure it’s secure, making sure there’s data governance, making sure there’s best of class engineering practices like code reusability and reproducibility,” he says. “Open source is a pretty big freaking space. There are dozens of dozens of technologies and libraries that you have to orchestrate and make work together. It’s not an easy task…..[but] that’s where the field is going. They want to leverage the best that open source provides and not be stuck in a rigid platform.”
Clearly, a data science platform won’t be the only tool in your big data tool set. We’ve seen demand for big data fabrics gaining momentum, too, and easy-to-use data science notebooks are also en vogue. But as the big data analytics market matures and new categories of software tools are established, it’s clear that enterprise capabilities will be near the top in terms of importance, and to that end, the rise of data science platforms makes sense.
Related Items:
Big Data Fabrics Emerge to Ease Hadoop Pain
Why You Need a Data Science Platform
The Rise of Data Science Notebooks