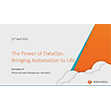
We’re In the Moneyball 3.0 Era. Here’s What It Means for Live Sports
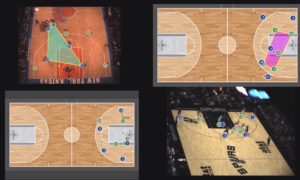
(Image courtesy Stats Perform)
When Oakland A’s General Manager Billy Beane adopted Sabermetrics with his 2002 and 2003 teams, he popularized what became known as the Moneyball 1.0 era. Eighteen years later, we’re firmly on the cusp of Moneyball 3.0, which will be marked by insights gleaned from deep learning models running against video data in real time, according to Stats Perform Chief Scientist Patrick Lucey.
Datanami caught up with Lucey, one of the foremost experts in sports analytics, recently to get an update on the state of the art. The last time we chatted, in 2017, Lucey’s thesis was that we were on the cusp of a major revolution thanks to deep learning (See “Deep Learning Is About to Revolutionize Sports Analytics. Here’s How.”)
Naturally, the first question Datanami posed to Lucey was whether that prediction was true. Has deep learning revolutionized sports analytics? His short answer: “I think it has.”
His longer answer is more interesting.
Moneyball 1.0
In Lucey’s telling of the history of sports analytics, the Moneyball era didn’t actually start with Beane in the MLB in the early 2000s, but with Daryl Morey nearly a decade earlier.
“Daryl Morey was part of the company [STATS] in the mid-90s,” Lucey said. “[He] started his basketball analytics career and obviously that changed how basketball is being played.”
Morey would go on to a historical run as the general manager of the Houston Rockets from 2007 to 2020, a period in which the James Harden-led Rockets had one of the best records in the NBA. Morey, who left the Rockets nearly a year ago to become the president of sports operations at the Washington Wizards, also is the co-chair of the MIT Sloan Sports Analytics Conference, which is basically the Consumer Electronics Show for sports data nerds. (He also was also the subject of a 2016 Michael Lewis book titled “The Undoing Project.”)
Moneyball 1.0 was a revolution in thinking, Lucey said. “You think about this story about Moneyball. It’s all about the value of data and using data to make better decisions,” Lucey said. “So instead of using your gut, let’s have some metrics.”
Moneyball 2.0
Moneyball 2.0 commenced around the year 2010, when STATS rolled out its SportVU program in the NBA, Lucey said. SportVU is a camera system that uses computer vision algorithms to track the movement of players on the screen. The technology was originally based on a missile tracking system developed by Israeli scientists, and STATS adapted it to track basketball and soccer play and players.
Through SportVU, Stats Perform (the company dropped the all-caps when it merged with Perform in 2019 to create a 1,600-person strong sports analytics powerhouse) is the official provider of player-tracking data to the NBA. This data largely is about how individual players move across the court or field, and for identifying specific plays, such as the pick-and-roll in basketball, or spotting formations in soccer (although the latter is something that the technology still struggles with).
“At the frame roll, 25 frames per second, it’s very precise,” Lucey said. “That’s important because [it shows] how quick [they are] and how they recover. At every frame, I know how far you’re away, the pressure–all those type of things. So we can start measuring things that you couldn’t measure before.”
Unfortunately, the need to invest in high-priced cameras has limited the adoption of the SportVU program, and prevented teams from benefiting from the detailed computer-generated version of what happened during the game.
“The last 10 years we’ve been really using in-venue solutions, really relying on cameras and these systems to kind of track play. But it’s limited in terms of scale because we haven’t been able to do it for every game that’s ever been played,” Lucey said. “We haven’t had that data exist in college basketball because in-venue solutions haven’t been able to scale, meaning that, if I’m making a decision in the NBA of a future player, I’m only going on a box score. I’m only going on play-by-play data.”
When it comes to advanced analytics, college basketball teams are trailing their NBA brethren, although some of the bigger programs are starting to look into. According to Lucey, we aren’t far from having a solution to this dilemma. It turns out, specialty camera equipment may not be needed much longer.
“We’ve been pioneers in the first success story for computer vision,” Lucey said. “Ten years ago, computer vision systems were based on color and edges and histograms. They were human generated features. Now the Big Bang has happened.”
Moneyball 3.0
The Big Bang, of course, is the explosion of deep learning models, which has mostly been in the realm of computer vision and natural language processing (NLP). Convolutional neural networks have proven to be very good at identifying and classifying objects in images–better than humans, in some cases.
Advanced player tracking data can now be gleaned from the TV broadcasts on CBS Sports, ESPN, or any other channel. Stats Perform makes this data available through its AutoStats program, which it launched in 2019.
“Really, the heart of the improvement to computer vision has been deep learning. And this scale has enabled us to collect tracking data from broadcast,” Lucey said. “We just rely on what you see from home. The nice thing about doing it from broadcast…is that the broadcast is generated by intelligent humans. The director and producer and the camera operators are very, very smart. They’re telling us what’s important.”
This is the Moneyball 3.0 era, and it’s marked by detecting things that couldn’t previously be detected, Lucey said. But the most important aspect of this new era of sports analytics is the capability to get these insights as they happen, he said.
“We spent a lot of time getting this done and we’re looking to scale that to other sports, like doing this live in soccer,” Lucey said. “The full value of data and decision-making is having that live. So having, first of all, the ecosystem to have that live data streaming. And then having the AI ecosystem to be able to produce predictions live.”
Live Insight and Simulation
Having live deep learning-based insights opens up a number of new possibilities, including the ability to conduct counterfactual analysis, Lucey said. In other words, this could enable a coach, commentator, or even a wagerer to ask whether the outcome of the game would have been different had a player done something different.
“Having that data coming in live and having the predictions live, having differentiated markets, team and player props, being able to generate insights, textual insight, which is personalized….there’s infinite things you could describe during the game,” Lucey said. “But really the focus that we’ve had is doing it at scale, which is what we talked about in computer vision. But also doing this live, whether it’s computer vision live or just generating live predictions and insights.”
Lucey stopped himself. “I’m being redundant in using live,” he said. “But that’s really the value. It doesn’t matter if it happens after the game. It doesn’t matter. It has to be live.”
Of course, that doesn’t mean Stats Perform isn’t interested in after-the-fact processing. The company is the official keeper of sports data for the NBA and is heavily involved in many other sports leagues, so the historical record is critically important. But what moves the needle for the company and its customers these days is the ability to enhance that real-time experience.
Where things get really interesting is how teams use these models built on public data to build simulations, so-called “ghosting” systems, that also use the teams’ private data. “That’s their secret sauce,” Lucey said. “A lot of them want to use it. But I think being able to leverage all the data and having these pretrained networks for a recruitment model and things like that–that’s definitely where things are trending.”
Each successive achievement in sports analytics – from tracking players in video to understanding actions to understanding the actual gameplay and then simulating it in a computer – gets us one step closer to achieving something remarkable, Lucey’s Stats Perform colleague, AI scientist Sujoy Ganguly, said in a video posted to the company’s website.
“The way I know that we will have understood or solved sports is when we can get a computer to simulate games of flow,” Ganguly said. “If we can do that reliably, then I feel like we will have understood completely all the aspects of the game. And therefore, we would have turned the computer into an Oracle. It’s not that the computer will tell you how to win. It will answer a question that you have. The real goal of machine learning and artificial intelligence is to free people up to be creative.”
Related Items:
Five Real-World Applications for Sports Analytics
When Citizen Data Science Meets Basketball Analytics
Deep Learning Is About to Revolutionize Sports Analytics. Here’s How