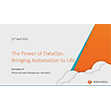
Most Data Science Projects Fail, But Yours Doesn’t Have To
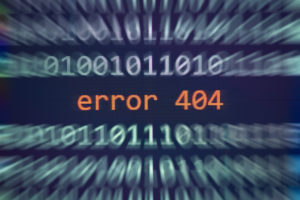
(Poring Studio/Shutterstock)
In an effort to remain competitive in today’s increasingly challenging economic times, companies are moving forward with digital transformations — powered by data science and machine learning — at an unprecedented rate. According to PwC ‘s global study, AI will provide up to 26% boost in GDP for local economies by 2030. Yet, for many companies, implementing data science into various aspects of their businesses can prove difficult if not daunting.
According to Gartner analyst Nick Heudecker, over 85% of data science projects fail. A report from Dimensional Research indicated that only 4% of companies have succeeded in deploying ML models to production environment.
Even more critical, the economic downturn caused by the COVID-19 pandemic has placed increased pressure on data science and BI teams to deliver more with less. In this down market, organizations are reassessing which AI/ML models they should develop, how to optimize resources and how to best use valuable budget dollars for maximum impact. In this type of environment, AI/ML project failure is simply not acceptable.
So, what causes data science projects to fail? There are a number of factors that contribute, with the top four being inappropriate or siloed data, skill/resource shortage, poor transparency and difficulties with model deployment and operationalization.
The Top Factors Impacting the Success of Data Science Projects
Data science project failure can have a huge impact on a company’s bottom line, there are a number of factors that drive failure:
Siloed Data: Data is spread across multiple databases in multiple formats not suitable for analytics. Many companies lack data infrastructure or do not have enough volume or quality data. Data quality and data management issues are critical given the high reliance on good quality data by AI and ML projects. However, traditionally, the approach companies take in order to solve any data issues requires months of effort. This time and the initial efforts associated with this approach often cause projects to fail after only a few months of investment and work.
Shortage of Skills: For nearly two years, there has been a widespread talent shortage in the data science space. LinkedIn reported in 2018 that there was a shortage of more than 150,000 individuals with data science skills. While the complex interdisciplinary approach of data science projects involves various subject matter experts such as mathematicians, data engineers, and many others, data scientists are often the most critical – and most difficult to recruit. This means companies are having a difficult time implementing and scaling their projects, which in turn, is slowing time to production. Additionally, many companies cannot afford the large teams required to run multiple projects simultaneously.
Poor Transparency: Within companies, there can often be disjointed expectations between technical and business teams. For instance, the data science teams typically put their focus on model accuracy, which is the simplest metric to measure, and the business teams place high importance on metrics such as business insights, financial benefit, and the interpretability of the models produced. This unclear alignment between the teams results in data science project failures as they are trying to measure completely different metrics. Also, traditional data science initiatives tend to use blackbox models which are hard to interpret, lack accountability and hence difficult to scale.
Deployment and Operationalization: In order for a data science to create real value for companies, there must be a complete understanding and view of how their projects will actually be used in production. However, more often than not, appropriate consideration is not given to this aspect. This can happen because data science teams do not have an architectural view into how their projects will be integrated within the production pipeline since these are typically managed by IT teams. The IT teams, in turn, have finite insight into the actual data science development process and how a soon-to-be-developed project will fit into their environments. This misalignment can often result in one-off data science projects that don’t deliver business value.
Avoiding the Pitfalls of Data Science Projects
Although, historically, the failure rate of data science projects has been high, it doesn’t mean that your organization’s projects should meet the same fate.
In order to help mitigate the factors that cause data science projects to fail, the industry has seen an increased interest among enterprises adopting end-to-end automation of the full data science process.
Through data science automation, companies are not only able to fail faster (which is a good thing in the case of data science), but to improve their transparency efforts, deliver minimum value pipelines (MVPs), and continuously improve through iteration.
Why is failing fast a positive? While perhaps counterintuitive, failing fast can provide a significant benefit. Data science automation allows technical and business teams to test hypotheses and carry out the entire data science workflow in days. Traditionally, this process is quite lengthy — typically taking months — and is extremely costly. Automation allows failing hypotheses to be tested and eliminated faster. Rapid failure of poor projects provides savings both financially as well as in increased productivity. This rapid try-fail-repeat process also allows businesses to discover useful hypotheses in a more timely manner.
Why is white box modelling important? White-box models (WBMs) provide clear explanations of how they behave, how they produce predictions, and what variables influenced the model. WBMs are preferred in many enterprise use cases because of their transparent ‘inner-working’ modeling process and easily interpretable behavior. For example, linear models and decision/regression tree models are fairly transparent, one can easily explain how these models generate predictions. WBMs render not only prediction results but also influencing variables, delivering greater impact to a wider range of participants in enterprise AI projects.
How does automation help organizations with continuous improvement? One of the critical challenges of organizations is the sheer time needed to complete AI and ML projects, usually in the order of months, and the incredible lack of qualified talent available to handle such projects. AutoML platforms address this by automating manual and iterative steps and enabling data science teams to rapidly test new features and validate models.
End-to-end data science automation enables teams to move faster, build and deploy rapidly and make adjustments easily as well as rebuild features and machine learning models with the latest data. Additionally, it also enables companies to explore new features from other data sources.
Following the Path to Data Science Success
To succeed in today’s business climate, companies must leverage data science automation to gain greater agility and faster, more accurate decision-making. The emergence of AutoML platforms allows enterprises to be more nimble by allowing them to tap into current teams and resources without having to recruit and additional talent. AutoML 2.0 empowers BI developers, data engineers and business analytics professionals to leverage AI/ML and add predictive analytics to their BI stack quickly and easily. By providing automated data preprocessing, model generation and deployment with a transparent workflow, AutoML 2.0 is bringing AI to masses thereby accelerating data science adoption.
About the author: Ryohei , Ph.D. is the Founder & CEO of dotData, a leader in full-cycle data science automation and operationalization for the enterprise. Prior to founding dotData, he was the youngest research fellow ever in NEC Corporation’s 119-year history, the title was honored for only six individuals among 1000+ researchers. During his tenure at NEC, Ryohei was heavily involved in developing many cutting-edge data science solutions with NEC’s global business clients and was instrumental in the successful delivery of several high-profile analytical solutions that are now widely used in the industry. Ryohei received his Ph.D. degree from the University of Tokyo in the field of machine learning and artificial intelligence.
Related Items:
5 Reasons Data Science Initiatives Fail
What is Feature Engineering and Why Does It Need To Be Automated?
On the Origin of Business Insight in a Data-Rich World