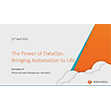
Room for Improvement in Data Quality, Report Says
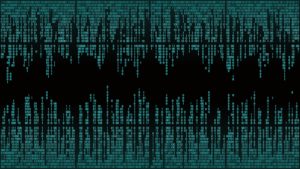
(Valery-Brozhinsky/Shutterstock)
A new study commissioned by Trifacta is shining the light on the costs of poor data quality, particularly for organizations implementing AI initiatives. The study found that dirty and disorganized data are linked to AI projects that take longer, are more expensive, and do not deliver the anticipated results. As more firms ramp up AI initiatives, the consequences of poor data quality are expected to grow.
The relatively sorry state of data quality is not a new phenomenon. Ever since humans started recording events, we’ve had to deal with errors. But when you couple today’s super-charged data generation and collection mechanisms with the desire of companies to become “data-driven” through advanced analytics and AI, the ramifications of automating decisions based on dirty or disorganized data cannot be ignored.
Trifacta did its best to describe the causes and effects of poor data quality, as well as the most common data sources and data wrangling activities, in its new report, titled “Obstacles to AI & Analytics Adoption in the Cloud.” The study, officially released today, is based largely on a survey of 600 high-ranking, U.S.-based business and technology executives conducted on the Internet by Researchscape International last August.
The survey explored various aspects of data quality. For starters, it found that just 26% of respondents reported that their data is “completely accurate,” while 42% said their data was “very accurate.” That would leave around one-third of survey respondents with somewhat or very inaccurate data (although those figures were not included in the report).
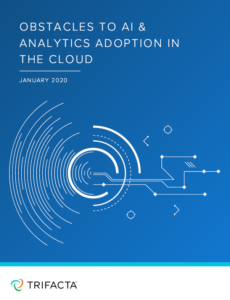
Trifacta’s report can be accessed online here.
That number jibes with another part of the survey, which found more than 33% to 38% of survey respondents reported that poor data quality had negatively impacted their AI and machine learning initiatives.
Drilling down, the survey found that poor data quality has various impacts on their AI and ML projects, with 38% saying their projects took longer, 36% saying their projects were more expensive, and 33% saying they did not achieve anticipated results.
These figures show that data quality has the attention of enterprise decision-makers, says Will Davis, Trifacta’s senior director of marketing.
“One of the biggest takeaways for me is how the C-Suite has finally noticed the ramifications of poor data quality,” Davis tells Datanami via email. “I think the critical importance of analytics initiatives to the overall success of businesses has brought this to the forefront.”
These data points suggest that roughly one-third of organizations have identified data quality as a major problem. Is that to say that roughly two-thirds of organizations are not struggling with data quality at this point? Davis says that could be the case, but added a caveat.
“That’s a fair estimate to say,” Davis says. “And the reason this statistic could be on the higher end is due to the number of organizations that are able to deploy advanced analytics and AI initiatives. AI remains an aspirational effort and often times leveraged in very small tests that are easier to control. As organizations begin to use AI for larger initiatives and more AI/ML projects are moved to the cloud, we anticipate more organizations will feel the impact of poor data quality.”
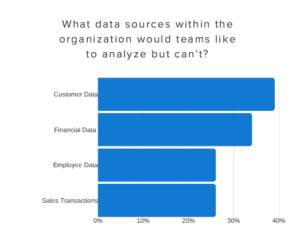
Access to first-party data is an issue for AI teams, Trifacta’s study found (image courtesy Trifacta)
Lest one believe that data quality is not a problem, here’s another takeaway from the report: three-quarters of survey respondents lack confidence in the quality of their data. That data point clearly shows that there is concern at the highest levels of the organization.
“Yes, 75% of C-Suite executives aren’t confident in the quality of their data and 74% of total respondents feel their data isn’t completely accurate before data prep processes,” Davis explains. “It shows that even at the highest level of the organization (the C-Suite) the quality of the data is in doubt.”
Only 14% of survey respondents say they’re getting access to the data they need. First-party data sources (i.e. data from inside the company) are among the biggest contributors to AI and ML initiatives, but survey-takers say they’re not getting enough access to customer data (cited by 39% of survey respondents), financial data (34%), employee data (26%), and sales transactions (26%). Second- and third-party data is also used; about half of survey respondents say they spend time moving, blending, and cleaning second- and third-party data, the report says.
When it comes to common data preparation tasks, the survey found that 28% reported accessing data from different systems took up their time, while 27% reported the need to merge disparate data sources together as a big consumer of time. One-quarter of respondents cited data reformatting as a common need.
Trifacta also asked how much time respondents take to prep their data for AI and ML initiatives. The results were split into four segments of equal size, including: one to four hours; five to nine hours; 10 to 19 hours; and more than 20 hours. Trifacta says these numbers support Forrester’s claim that data preparation consumes about 80% of data analysts’ time.
This was the first data quality survey of this type from Trifacta. The company says it plans to conduct this survey annually to provide a benchmark for data quality in the enterprise.
You can download Trifacta’s report at www.trifacta.com/gated-form/cloud-ai-adoption-survey.
Related Items:
A Bottom-Up Approach to Data Quality
As Data Quality Declines, Costs Soar
Data Quality Trending Down? C’est La Vie