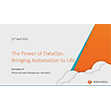
Big Data Predictions: What 2020 Will Bring
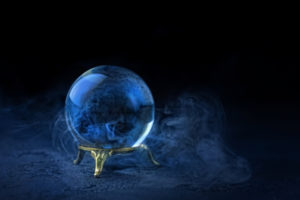
(ju_see/Shutterstock)
With just over a week left on the 2019 calendar, it’s now time for predictions. We’ll run several stories featuring the 2020 predictions of industry experts and observers in the field. It all starts today with what is arguably the most critical aspect of the big data question: The data itself.
There’s no denying that Hadoop had a rough year in 2019. But is it completely dead? Haoyuan “HY” Li, the founder and CTO of Alluxio, says that Hadoop storage, in the form of the Hadoop Distributed File System (HDFS) is dead, but Hadoop compute, in the form of Apache Spark, lives strong.
“There is a lot of talk about Hadoop being dead,” Li says. “But the Hadoop ecosystem has rising stars. Compute frameworks like Spark and Presto extract more value from data and have been adopted into the broader compute ecosystem. Hadoop storage (HDFS) is dead because of its complexity and cost and because compute fundamentally cannot scale elastically if it stays tied to HDFS. For real-time insights, users need immediate and elastic compute capacity that’s available in the cloud. Data in HDFS will move to the most optimal and cost-efficient system, be it cloud storage or on-prem object storage. HDFS will die but Hadoop compute will live on and live strong.”
As HDFS data lake deployments slow, Cloudian is ready to swoop in and capture the data into its object store, says Jon Toor, CMO of Cloudian.
“In 2020, we will see a growing number of organizations capitalizing on object storage to create structured/tagged data from unstructured data, allowing metadata to be used to make sense of the tsunami of data generated by AI and ML workloads,” Toor writes.
The end of one thing, like Hadoop, will give rise the beginning of another, according to ThoughtSpot CEO Sudheesh Nair.
“Over the last 10 years or so, we’ve seen the rise, plateau, and the beginning of the end for Hadoop,” Nair says. “This isn’t because Big Data is dead. It’s exactly the opposite. Every organization in the world is becoming a Big Data company. It’s a requirement to operate in today’s business landscape. Data has become so voluminous, and the need for agility with this data so great, however, that organizations are either building their own data lakes or warehouses, or going directly to the cloud. As that trend accelerates in 2020, we’ll see Hadoop continue to decline.”
When data gets big enough, it exerts a gravitational-like force, which makes it difficult to move, while also serving to attract even more data. Understanding data gravity will help organizations overcome barriers to digital transformation, says Chris Sharp, CTO of Digital Realty.
“Data is being generated at a rate that many enterprises can’t keep up with,” Sharp says. “Adding to this complexity, enterprises are dealing with data – both useful and not useful – from multiple locations that is hard to move and utilize effectively. This presents enterprises with a ‘data gravity’ problem that will prevent digital transformation initiatives from moving forward. In 2020, we’ll see enterprises tackle data gravity by bringing their applications closer to data sources rather than transporting resources to a central location. By localizing data traffic, analytics and management, enterprises will more effectively control their data and scale digital business.”
All things being equal, it’s better to have more data than less of it. But companies can move the needle just by using available technology to make better use of the data they already have, argues Beaumont Vance, the director of AI, data science, and emerging technology at TD Ameritrade.
“As companies are creating new data pools and are discovering better techniques to understand findings, we will see the true value of AI delivered like never before,” Vance says. “At this point, companies are using less than 20% of all internal data, but through new AI capabilities, the remaining 80% of untapped data will be usable and easier to understand. Previous questions which were unanswerable will have obvious findings to help drive massive change across industries and societies.”
Big data is tough to manage. What if you could do AI with small data? You can, according to Arka Dhar, the CEO of Zinier.
“Going forward, we’ll no longer require massive big data sets to train AI algorithms,” Dhar says. “In the past, data scientists have always needed large amounts of data to perform accurate inferences with AI models. Advances in AI are allowing us to achieve similar results with far less data.”
How you store your data dictates what you can do with it. You can do more with data stored in memory than on disk, and in 2020, we’ll see organizations storing more data on memory-based systems, says Abe Kleinfeld, the CEO of GridGain.
“In 2020, the adoption of in-memory technologies will continue to soar as digital transformation drives companies toward real-time data analysis and decision-making at massive scale,” Kleinfeld says. “Let’s say you’re collecting real-time data from sensors on a fleet of airplanes to monitor performance and you want to develop a predictive maintenance capability for individual engines. Now you must compare anomalous readings in the real-time data stream with the historical data for a particular engine stored in the data lake. Currently, the only cost-effective way to do this is with an in-memory ‘data integration hub, based on an in-memory computing platform like Apache Ignite that integrates Apache Spark, Apache Kafka, and data lake stores like Hadoop….2020 promises to be a pivotal year in the adoption of in-memory computing as data integration hubs continue to expand in enterprises.”
Big data can make your wildest business dreams come true. Or it can turn into a total nightmare. The choice is yours, say Eric Raab and Kabir Choudry, vice presidents at Information Builders.
“Those that have invested in the solutions to manage, analyze, and properly action their data will have a clearer view of their business and the path to success than has ever been available to them,” Raab and Choudry write. “Those that have not will be left with a mountain of information that they cannot truly understand or responsibly act upon, leaving them to make ill-informed decisions or deal with data paralysis.”
Let’s face it: Managing big data is hard. That doesn’t change in 2020, which will bring a renewed focus on data orchestration, data discovery, data preparation, and model management, says Todd Wright, head of data management and data privacy solutions at SAS.
“According to the World Economic Forum, it is predicted by 2020 that the amount of data we produce will reach a staggering 44 zettabytes,” Wright says. “The promise of big data never came from simply having more data – and from more sources – but by being able to develop analytical models to gain better insights on this data. With all the work being done to advance the work of analytics, AI and ML, it is all for not if organizations do not have a data management program in place that can access, integrate, cleanse and govern all this data.”
Organizations are filling up NVMe drives as fast as they can to help accelerate the storage and analysis of data, particularly involving IoT. But doing this alone is not enough to ensure success, says Nader Salessi, the CEO and founder of NGD Systems.
“NVMe has provided a measure of relief and proven to remove existing storage protocol bottlenecks for platforms churning out terabytes and petabytes of data on a regular basis,” Salessi writes. “Even though NVMe is substantially faster, it is not fast enough by itself when petabytes of data are required to be analyzed and processed in real time. This is where computational storage comes in and solves the problem of data management and movement.”
Data integration has never been easy. With the ongoing data explosion and expansion of AI and ML use cases, it gets even harder. One architectural concept showing promise is the data fabric, according to Ravi Shankar, senior vice president and chief marketing officer at Denodo.
“Through real-time access to fresh data from structured, semi-structured and unstructured data sets, data fabric will enable organization to focus more on ML and AI in the coming year,” Shankar says. “With the advancement in smart technologies and IoT devices, a dynamic data fabric provides quick, secure and reliable access to vast data through logical data warehouse architecture. Thus, facilitating AI-driven technologies and revolutionizing businesses.”
Seeing how disparate data sets are connected using semantic AI and enterprise knowledge graphs (EKG) provide other approaches for tackling the data silo problem, says Saurav Chakravorty, the principal data scientist at Brillio.
“An organization’s valuable information and knowledge is often spread across multiple documents and data silos, creating big headaches for a business,” Chakravorty says. “EKG will allow organizations to do away with semantic incoherency in fragmented knowledge landscape. Semantic AI with EKG complement each other and can bring great value overall to enterprise investments in data lake and big data.”
2020 holds the potential to be a breakout year for storage-class memory, argues Charles Fan, the CEO and co-founder of MemVerge.
“With an increasing demand from data center applications, paired with the increased speed of processing, there will be a huge push towards a memory-centric data center,” Fan says. “Computing innovations are happening at a rapid pace, with more and more computation tech–from x86 to GPUs to ARM. This will continue to open up new topology between CPU and memory units. While architecture currently tends to be more disaggregated between the computing layer and the storage layer, I believe we are headed towards a memory-centric data center very soon.”
We are rapidly moving toward a converged storage and processing architecture for edge deployments, says Bob Moul, CEO of machine data intelligence platform Circonus.
“Gartner predicts there will be approximately 20 billion IoT-connected devices by 2020,” Moul says. “As IoT networks swell and become more advanced, the resources and tools that managed them must do the same. Companies will need to adopt scalable storage solutions to accommodate the explosion of data that promises to outpace current technology’s ability to contain, process and provide valuable insights.
Dark data will finally see the light of day in 2020, according to Rob Perry, the vice president of product marketing at ASG Technologies.
“Every organization has islands of data, collected but no longer (or perhaps never) used for business purposes,” Perry says. “While the cost of storing data has decreased dramatically, the risk premium of storing it has increased dramatically. This dark data could contain personal information that must be disclosed and protected. It could include information subject to Data Subject Access Requests and possible required deletion, but if you don’t know it’s there, you can’t meet the requirements of the law. Though, this data could also hold the insight that opens up new opportunities that drive business growth. Keeping it in the dark increases risk and possibly masks opportunity. Organizations will put a new focus on shining the light on their dark data.”
Open source databases will have a good year in 2020, predicts Karthik Ranganathan, founder and CTO at Yugabyte.
“Open source databases that claimed zero percent of the market ten years ago, now make up more than 7%,” Ranganathan says. “It’s clear that the market is shifting and in 2020, there will be an increase in commitment to true open source. This goes against the recent trend of database and data infrastructure companies abandoning open source licenses for some or all of their core projects. However, as technology rapidly advances it will be in the best interest of database providers to switch to a 100% open source model, since freemium models take a significantly longer period of time for the software to mature to the same level as a true open source offering.”
However, 2019 saw a pull back away from pure open source business models from companies like Confluent, Redis, and MongoDB. Instead of open source software, the market will be responsive to open services, says Dhruba Borthakur, the co-founder and CTO of Rockset.
“Since the public cloud has completely changed the way software is delivered and monetized, I predict that the time for open sourcing new, disruptive data technologies will be over as of 2020,” Borthakur says. “Existing open-source software will continue to run its course, but there is no incentive for builders or users to choose open source over open services for new data offerings…..Ironically, it was ease of adoption that drove the open-source wave, and it is ease of adoption of open services that will precipitate the demise of open source particularly in areas like data management. Just as the last decade was the era of open-source infrastructure, the next decade belongs to open services in the cloud.”
Related Items:
2019: A Big Data Year in Review – Part One