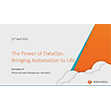
Ethical Data Science Is Good Data Science
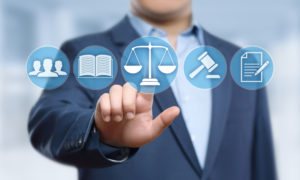
(Alexander Supertramp/Shutterstock)
There’s no doubt about it: The future will be machine driven, and central to this future are the advanced algorithms, which are fueled by the data they’re trained on. Every ad you see, every car driving itself, every medical diagnosis provided by a machine will be based on your data – and lots of it.
Without your data, we inherit a world without machine learning, and most would argue that companies without machine learning will fail. At least that’s where we’re heading; it sounds like a big problem, and it is.
Concepts around “big data” are completely incompatible with how people expect their data to be protected and how laws are shaping those protections. In fact, the GDPR, a data privacy regulation enacted in the EU, treats your data as if it’s an extension of your body. And more regulations like GDPR are coming.
One of the key tenets of the GDPR, and of the new wave of data regulations, is limiting data usage to specific purposes. The GDPR does not simply restrict how or what data is collected, it restricts how the collected data is being used.
The GDPR requirements show us a pathway that can actually translate into better data privacy protections, and ultimately, better data science. Put simply, GDPR is a manifestation of the data governance initiatives all organizations should have been doing all along. Building data governance across machine learning activities will accelerate innovation – not stifle it.
So where does this leave organizations building products based on user data – and organizations running their businesses with algorithms powered by user data? Is the Facebook fiasco the beginning of the end of data-driven initiatives? What are the lessons and steps we can take in reaction to this?
The utility of the show on the Hill is that it could start a real conversation on how to protect both the innovations driven by algorithms and consumer’s privacy when it comes to their data. Here are three steps that businesses – and the technology companies supporting them – need to take:
End the Data Usage Free-for-All
Just because you’re able to collect massive amounts of data does not mean that every user in an organization should be able to use and touch all aspects of that data. GDPR terms this “privacy by design”, but I term it common sense.
Should all your data scientists see all your data subjects’ Social Security number when they’re building a fraud analytic? No.
When you work with 3rd parties, where your data is “better together,” should you share it all? No.
This means enforcing fine-grained controls on your data. Not just coarse-grained role-based access control (RBAC), but down to the column and row level of your data, based on user attributes and purpose (more on that below). You need to employ techniques such as column masking, row redaction, limiting to an appropriate percentage of the data, and even better, differential privacy to ensure data anonymization.
In almost all cases, your data scientists will thank you for it. It provides accelerated, compliant access to data and with that a great deal of comfort, freedom, and collaboration that comes when everyone knows they are compliant in what they are doing and can share work more freely. This freedom to access and share data comes when data controls are enforced at the data layer consistently and dynamically across all users. It provides the strong foundation needed to enable a high performing data science team.
Purpose Based Restrictions Gives Accountability
All use of data should have a purpose – and data usage should be restricted to those purposes. This is critical to modernizing the way data is governed. While seemingly incompatible with the promise of big data, purpose-based restrictions represent the future of privacy.
This also creates a new level of accountability across an organization – providing a broader understanding of how – and why – data is being used.
Monitoring Your Models
Last is the importance of “bookkeeping” for machine learning. This sounds simple, but in practice is more difficult than it seems. Keep track of all the data that is going into all your models. Models need constant monitoring, but this should not be limited to the output. It also includes the inputs and understanding the risk and regulations associated with that data.
Imagine if you could monitor, similar to a infrastructure dashboard, the risk associated with all your production models based on the data that went into training them. Or understand data value across your organization based on how often it’s being leveraged for predictions. Sounds useful – and it is! Equally important, this type of approach lets you manage change more easily. If a policy on how you can use data changes, this lets you understand what models it impacts and react appropriately.
The future of data privacy is about more than letting consumers know how and where there data is collected. Data-driven companies and the developers of tomorrow’s technology need to think beyond privacy checkboxes and build technology that allows us to manage how our data is used.
As the Facebook circus shows, there may not be a penalty now for how you’re using data, but the oncoming GDPR regulations will change everything. It’s simply a matter of time before the U.S. enacts similar regulations. Getting ahead of it now can save a lot of heartache tomorrow. You might literally not be able to afford to wait.
About the author: Steve Touw is co-founder and CTO of Immuta. Steve has a long history of designing large-scale geotemporal analytics across the US intelligence community, including some of the very first Hadoop analytics as well as frameworks to manage complex multitenant data policy controls. He and his co-founders at Immuta drew on this real-world experience to build a software product to make data experimentation easier. Previously, Steve was the CTO of 42Six Solutions (acquired by Computer Sciences Corporation), where he led a large big data services engineering team. Steve holds a BS in geography from the University of Maryland
Related Items:
Immuta Cashes In on Data Privacy Scramble
Keeping Your Models on the Straight and Narrow
Opening Up Black Boxes with Explainable AI