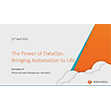
Can Big Data Deliver on the Huge Expectations of Precision Medicine?
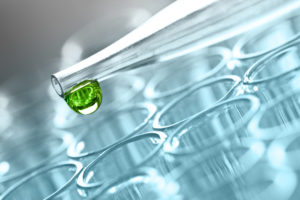
Big data can do a lot of things. But can it allow doctors to create drugs that are tailor-made to your genes, reduce the cost of healthcare for a billion people, and let us all live to 150? Only time will tell, but there are certainly those in the industry, like Cloudera co-founder Mike Olson, who are bullish on big data’s potential to create a healthcare renaissance through precision medicine.
There are many ways big data technologies are creeping into the healthcare space. Genomic sequencing would not have become as popular without a way to store all those data sets, which weigh in at 200GB per person. You also have the rise of Fitbits and other wearables that are part of the fitness-data craze, and even AIs that read MRI scans.
But there’s something else going on behind the scenes, something that Olson, Cloudera‘s chairman and chief strategy officer, believes will result in big changes to the practice of healthcare.
“The bald fact is we’ve never been able to collect this much data and this much variety before,” Olson says. “And even if we had, we would not have been able to build the analytic systems to combine it and work with it. And we know how to do that now.”
A Reset on Health
The Federal Government is spending $215 million this year on President Barack Obama’s Precision Medicine Initiative (PMI), which was announced in early 2015 and promises to help create much more targeted medical treatments than science has been able to deliver up to this point.
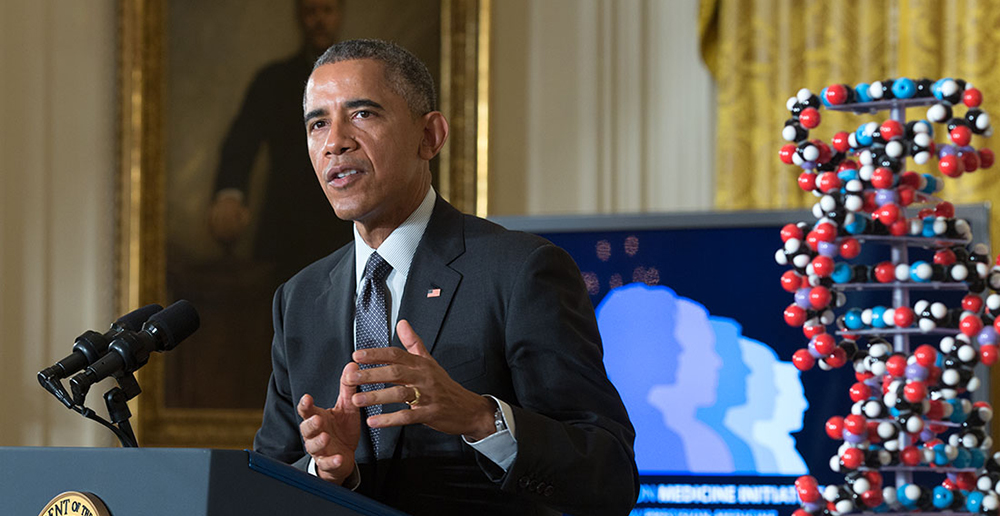
President Obama unveiled the Precision Medicine Initiative in early 2015
One of the focuses of PMI–which Fast Company called “the ultimate big-data project”— is cancer. Historically, cancer treatments have been mostly generalized, and oncologists have few options when coming up with a treatment plan. You would get largely the same treatment that the 30,000 people who got a certain type of cancer before you. It may increase your odds of survival by 30 percent, and that was better than nothing.
But we’re on the cusp of new treatments that could dramatically improve our odds. Instead of creating drugs that target at an average patient, researchers can now create drug therapies that are designed for individual’s specific DNA, potentially boosting the drug’s efficacy by a wide margin.
The approach has already been proven for certain types of breast and colorectal cancers, and now the race is on to scale it out to the masses. That’s where big data platforms and tools come in, Olson says.
“We want to use not just wet-lab chemistry, not just designing molecules and testing them on populations–we want to use informatics,” he tells Datanami. “We want to collect not just genomic data, but also information about their phenotype… stuff like resting heart rate or height or weight…..We’re using informatics and wet lab science together to do that work.”
A Playground of Exploration
The advent of new platforms like Apache Hadoop and new frameworks like Apache Spark are giving scientists powerful new tool to crunch through huge reams of data and find the patterns that lead to new cures.
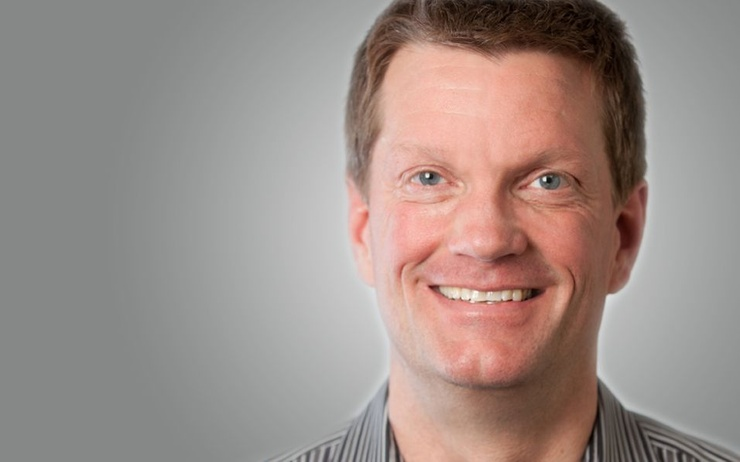
Cloudera Chief Strategy Officer Mike Olson is bullish on the long-term possibility for big data analytics to drive precision medicine
“The data volumes—the petabyte-scale volumes–were simply impossible to store affordably until this new generation of scale-out systems, and big data built on Apache Hadoop came along,” Olson continues. “It’s only now that we can build a database of genomic sequence that has everything we want and that we can keep in in spinning storage at a cost we can afford to pay.”
The twin phenomenon of big data storage and big data analytics are set to play a much bigger role in this new era of personalized medicine, says Olson, who described the cutting edge as a veritable playground of technology.
“Places where we simply didn’t have the data or the tools before, we can suddenly bring a whole new regiment to the research,” he says. “The question is, How do we do that in a cost effective and affordable way? If we have a patient population of 100 people worldwide who have a particular condition, can we design, can we produce that molecule in a cost-effective way? I’m long-term bullish on our ability to figure out how to do that.”
As a member of Obama’s PMI, Cloudera is donating tens of millions of dollar’s worth of software licenses and training to help accelerate research in personalized healthcare. “We’re super pleased to be participating in the PMI,” he says. “We need the best minds in the industry taking advantage of these tools and learning new lessons.”
Industrywide Challenges
While the healthcare industry has powerful new tools for crunching more data than it’s ever seen, there are big obstacles that must be overcome.
For starters, the digitization of data in the healthcare business is in its infancy. Before Congress made electronic medical records (EMR) the law with the Health Information Technology for Economic and Clinical Health (HITECH) Act in 2009, less than 10 percent of hospitals kept records in electronic format.
Since then, digitization has skyrocketed and EMRs have been adopted by roughly 80 percent of hospitals. That has greatly expanded the potential for sharing the types of clinical data that researchers increasingly would like to get their hands on—particularly for big longitudinal studies, such as the Million Veteran Program, a Department of Veterans Affairs plan to share anonymized medical data for a million current and retired service members, and other similar efforts.
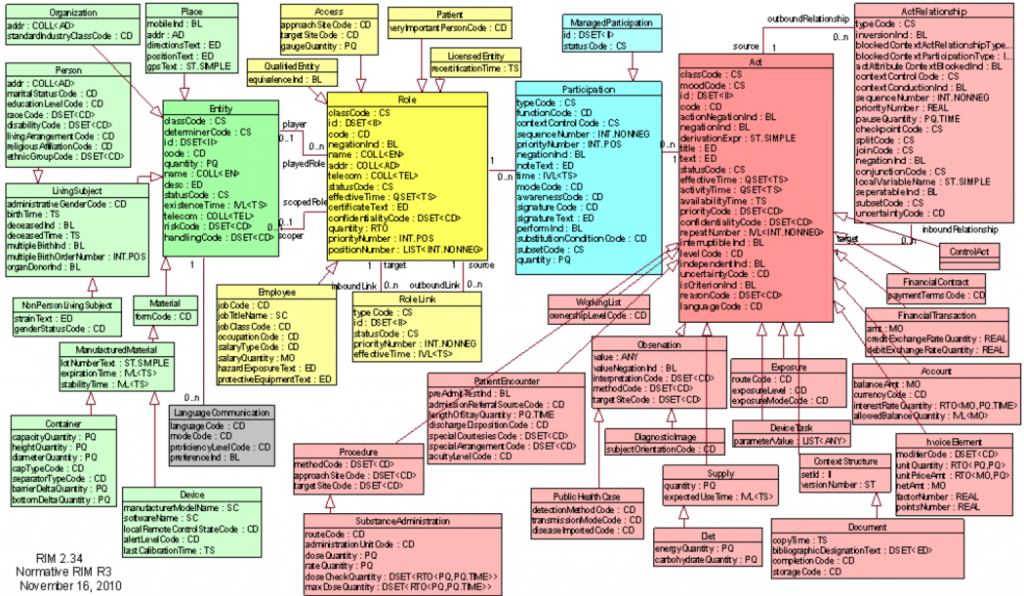
The HL7 v3 Reference Information Model, per healthstandards.com, really is that intimidating
The problem is, there’s no standard for EMRs. “We have 14,000 interfaces that we deal with,” says Gary Palgon, an executive with Liaison Technologies, which runs a cloud-based service that companies in the healthcare field and other industries use to store, transform, and deliver data to customers and partners.
It takes years to create standards, such as the electronic data interchange (EDI) format that automates the consumer processed goods (CPG) supply chain. HL7, an EDI-like standard used in healthcare, is a good start, but it’s often implemented in a haphazard fashion.
“Healthcare is 30 years behind [CPG], if you will,” Palgon says. “Where I see the ERP vendors in the late 90s and early 2000s saying ‘Give us access to data that you have in the system, then we can do something with it,’ healthcare is just like that.”
While Liaison doesn’t do analytics, it does do a lot of the grunt work of tapping data sources and standardizing formats that’s required before the data scientist can get to it. The company, which relies on a Hadoop cluster from MapR Technologies, serves data to and from about 1,500 customers in the healthcare and life sciences companies, including 170 laboratories in the United States.
“What interesting about this is you have all these high-paid PhDs, especially in the pharma and healthcare world, and they spend 80 percent of their time being a data janitor,” Palgon says. “So what we’re trying to do is flip that upside down, where they can spend 80 percent of the time actually interpreting the data, whether it’s clinical trials or genomics.”
Big Data’s Clinical Setting
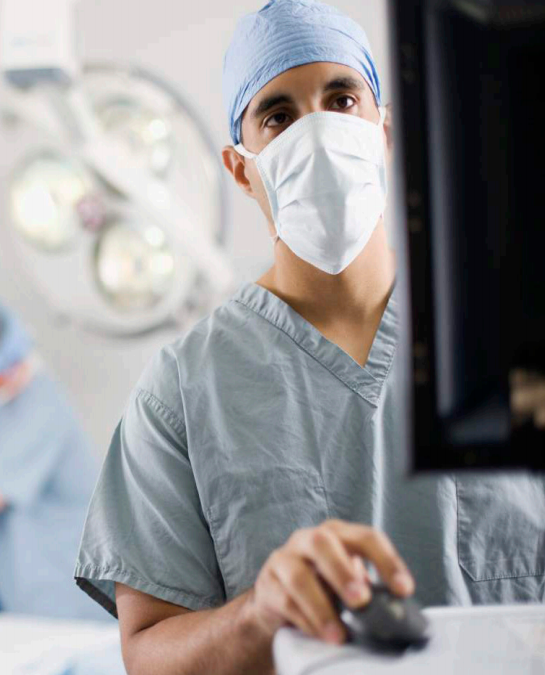
The University of Iowa Hospitals and Clinics reduced incidence of surgical site infection by 58 percent after implementing a predictive analytics application based on Dell’s Statistica software
But big data’s bite won’t only be felt in large, multi-year initiatives, and in fact it’s playing out on a daily basis in operating rooms around the country.
The University of Iowa Hospitals and Clinics, for example, is using analytics from Dell’s Statistica group to predict the likelihood of surgical site infections. By merging data from EMRs with data collected from the operating room, the hospital was able to reduce the occurrence of surgical site infections by 58 percent.
The predictions from the Statistica models enhance “the precision in the decision-making that we use for surgery,” said Dr. John Cromwell, the director of the hospital group’s division of gastrointestinal, minimally invasive and bariatric surgery. The impact on patient care is “really incredible,” he says in a case study.
Surgical site infections represent an annual cost of $10 billion to the $3-trillion US healthcare system. By more closely monitoring patients, analytic models such as the one built by the University of Iowa Hospitals and Clinics can reduce those costs and save lives at the same time.
Persistence of Optimism
While there’s no shortcutting data science’s dirty little secret, there’s also no telling where big data will take us in the healthcare space. As Cloudera’s Olson noted, this is all new. We haven’t been down this road before, and the possibilities are enticing. It’s worth thinking big.
“It’s absolutely true that we didn’t have these tools a few years ago,” he says. “It’s exciting that we do today. I wouldn’t say that we’ve got what we need, and as a result, there’s going to be huge innovation just on top of the platform. We’re going to learn a lot in the next couple years that we spend in the lab and in places like the Broad and elsewhere.”
The Broad Institute is a joint collaboration by MIT and Harvard University that aims to push the state of the art in biomedical research forward. The institute currently uses a 20PB cluster based on Cloudera’s Distribution of Hadoop (CDH) that it uses to store and analyze genomic data.
“We’ve been working with the Broad for some time,” Olson says. “They’re using a whole bunch of new parts of the platform. They’re a big adapter of Spark. They’re working with hospitalist, with pharmaceutical companies and researcher institutes around the world to drive analysis of that genomic data and to tie the genome to disease.”
Olson notes that we sequenced the first human genome a decade ago for $300 million, and today it costs about $300, or 100,000 times less. Those are the types of numbers that spark the hope that detectable patterns hidden in the data will point us to new approaches to solving old illnesses.
“Diseases that had previously been death sentences are going to be long-term managed diseases, or even curable disease, because we understand the genomic sequence,” Olson says. “What that means is that even very stupid ideas in this space will pay off. We’ve never been able to test even rudimentary new approaches before. We’re going to find huge value relatively quickly from relatively simple analytics. And as our sophistication increases, we’ll build better and more sophisticated analytic. We’ll learn a lot more. Advances may get tougher as we knock off the first few wins. But I have no reservations at all about the opportunity to advance and about the speed at which we’re going to see improvements.”
Related Items:
The Bright Future of Semantic Graphs and Big Connected Data
Big Data and the White House’s Cancer Moonshot