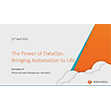
Big Data’s Cold Feet Syndrome
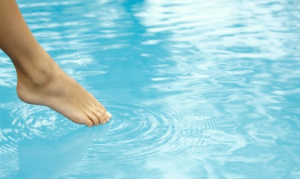
With all the hype around big data, it sometimes seems that everybody and her grandmother are doing it. But the truth is that implementing big data analytics can be almost as difficult as it is potentially profitable. And while the technical complexity makes it challenging, just getting employees to climb aboard the big data bandwagon, change their daily work patterns, and believe in the new analytic-powered systems is a challenge in itself.
The secret about big data is out, and the race to become a data-driven company is on. Management consultant companies are in overdrive to get their clients working on data analytic projects to improve margins, boost revenue, cut costs, and make customers happier. Just as the introduction of the database in the ’70s helped to revolutionize back-office processes, the rise of big data tech in the teens is remaking how companies across all industries interact with customers and each other.
There’s no doubt that big data is a big deal, but there are some hitches in big data’s giddy-up that threaten to derail the wagon train before it really gets going. According to one practitioner whose company has implemented big data projects at companies like JP Chase Morgan, T-Mobile, and United Airlines, managing the human side of the equation should not be lost amid the bits and bytes.
“Change management is a big headache,” says Arnab Gupta, founder, CEO, and chairman of data analytic product and services company Opera Solutions. “How do you get people to utilize a different model of execution? They’re all used to doing things in a certain way and getting them to do it a different way takes work. You put in these solutions, the question is, Will they use it? That’s the issue. The real problem is adoption.”
The big data movement is still in its infancy, and the models of execution are not yet set in stone. While Hadoop heavyweights like Cloudera, Hortonworks, and MapR Technologies are giving us plenty of sandboxes to get started with analytics and even lightweight application frameworks to jumpstart specific use cases like fraud detection and making personalized recommendations, getting a big data analytic application built and deployed requires a lot of handholding today.
Gupta, who founded Opera Solutions in 2004, recommends that organizations getting into the business of extracting business signals from big data learn to exercise patience with the human element.
“People are people. It just takes a little time,” he tells Datanami. “It does happen, but it doesn’t happen overnight. Regardless of the merits of your solution…if you don’t pay attention or have people thinking about it or a management team oriented toward change management or acceptance, no matter how smart you are, you won’t get that far.”
Warming Up to Big Data
This dynamic will change as big data analytics becomes more commonplace and people who are familiar with the technology move into leadership roles. This would appear inevitable, especially with millennials who grew up interacting with Apple‘s digital assistant Siri, who puts a pretty face on a morass of complex and sophisticated algorithms working on huge clusters behind the scenes to gather and dispel whatever data is requested of her.
And as the big data software gets better and easier to use, people will naturally warm to it and become more accepting of the big-data way. (Resistance, as The Borg once said, is futile.) This is the dynamic that Gupta is banking on as he transitions his big data firm from a services-first strategy to focus more on product development, specifically Signal Hub.
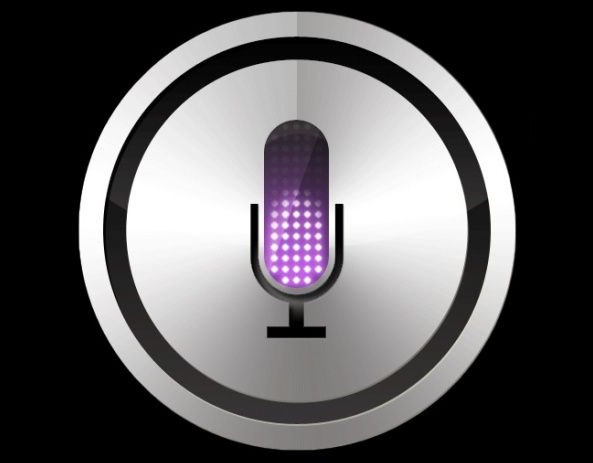
Siri has an answer for everything
Gupta has observed an 80/20 rule when it comes to Opera Solutions clients. That is, 80 percent of the time goes into prepping the data, and 20 percent of the time goes into actually using it for analytics. With Signal Hub, Gupta hopes to turn those numbers around, and let customers spend much more time doing actual analytics instead of wrangling and cleansing the data.
Whether the customer is in retail or telecom, airlines or healthcare, Gupta says the same basic principles apply to gathering data, manipulating machine learning models, and deriving actionable business insights from them. Signal Hub, which can run atop Hadoop or massively parallel data warehouses, aims to help customers re-use those assets to boost productivity.
“The same underlying approach applies, even though the industries themselves have different data models and classes of problems,” Gupta says. “Right now the basic problem we’re solving for big data is a productivity problem. The second problem we solve is about knowledge re-use…So if somebody builds something that’s useful for one use case or domain, it can be adapted and reused for something else.”
Next Problem: Addressing Value
With more than 100 engineers and more than 150 data scientists working from half-a-dozen locations around the world, Opera Solutions has the heft to help its clients overcome resistance to big-data change and embrace the analytic-driven way of doing things. But for smaller firms, it may require a different approach to get them to change and overcome the inertia of the familiar. We may be years away from having shrink-wrapped solutions that can fully deliver this, Gupta says.
If we want to move the ball faster away from big data’s cold feet syndrome, focusing on education and the words we use would be a good start, Gupta says. “When you say ‘Hadoop,’ 90 percent of them don’t know what the hell what you’re talking about,” he says. “There’s a huge communication issue that we have to resolve here.”
As software vendors like Opera Solutions hammer away at the productivity problem and make big data technology more shrink-wrapped and easier to use, it gives businesses a little bit of time to prep themselves for changes that are coming. Besides the adoption problem, finding out how to create value from big data is another stumbling block with no easy answers.
“What very few of them understand is the way in which value is created. That’s where the trick is, you know?” he says. “It’s no longer the operationalization of information. It’s about the operationalization of intelligence. What form does the data take when it transforms from information to intelligence and how do you operationalize intelligence. That’s the real issue for the IT organizations and the business users in these large companies.”
Related Items:
Big Data’s Dirty Little Secret
Alex, thanks for a great article. The “plumbing” task of integrating data across platforms can be a painful part of the analytics preparation process. Enterprises can alleviate the pain by automating the ETL coding process and using “change data capture” software to efficiently send updates to their target repositories. This frees up time and resources to focus on actual Data Science.
– Kevin Petrie, http://www.attunity.com