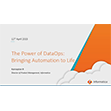
Big Data’s Dirty Little Secret
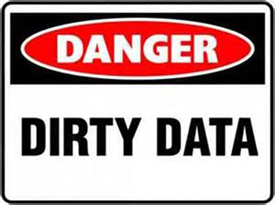
The twin phenomena of big data and machine learning are combining to give organizations previously unheard of predictive power to drive their businesses in new ways. But behind the big data headlines that tease us with tales of amazing insight and business optimization lurks an inconvenient truth: raw data is very dirty and requires an enormous amount of effort to clean.
Data scientists are undoubtedly the rock stars of the big data movement, as they use their keen understanding of statistics and machine learning to glean patterns in huge data sets, and then set up operational systems so their employers can profit from those insights. While this does happen on a daily basis, it glosses over the reality of the situation, which is that data scientists spend most of their time as data janitors.
According to a recent survey commissioned by Xplenty, which provides a Hadoop-based ETL service that runs in the cloud, raw data is so dirty that 30 percent of business intelligence professionals spend 50 to 90 percent of their time cleaning the data so that it can be analyzed.
“Reformatting, cleansing and consolidating large volumes of data from multiple sources can be overwhelming,” Yaniv Mor, CEO and co-founder of Xplenty, said in a press release. “BI professionals should be spending the majority of their time evaluating data and deciphering patterns gleaned through the analytics process—not readying data for analytics.”
When Xplenty asked more than 200 BI professionals what the biggest challenges they faced in making the data “analytics ready,” 55 percent of them said integrating the data from different platforms followed by transforming, cleansing, and formatting incoming data (39 percent), integrating relational and non-relational data (32 percent), and the sheer volume of data that needs to be managed at any given time (21 percent).
The study mirrors the anecdotal evidence provided by others in the big data cleansing business. Joe Hellerstein, the co-founder of Trifacta and a computer science professor at Cal Berkeley, last year told Datanami that data professionals often spend 50 to 80 percent of their time munging, wrangling, and cleaning their dirty data.
Trifacta is one of the companies, like Xplenty, that’s aiming to get customers out from under the data cleaning business. “We’re very proudly data janitors,” Trifacta’s new CEO Adam Wilson said at the recent Hadoop Summit. “We love the fact that we take care of this nasty, messy problem.”
Xplenty’s Mor elaborated on the dirty-data problem in a November interview with Datanami. “Most of the time you cannot perform analytics on raw data. It’s just too complex,” he said. “Most business analysts and data users need to have the data massaged and transformed before they do the analytics. Then, data scientists–the really smart people–need to gain access to the raw data and to write code on Hadoop to identify the trends that no one else can identify, and see the things that no one else can see.”
Mor says Xplenty is the first company to offer a dedicated Hadoop-based data integration and cleansing service that runs on public cloud platforms, such as those from Amazon, Microsoft, IBM, Google, and Rackspace. Customers can build their data integration and transformation pipelines using a graphical interface that doesn’t require the user to have specialized skills.
“What we’re doing is not new in the sense that people have been doing that since the dawn of the database age, definitely when the data warehouse methodologies started to emerge,” Mor said. “You have raw data. You transform it, normalize it, prepare it, and then put it into data warehouse. This is nothing new. But what’s new with our product is that it’s built on Hadoop as a big data technology and that it’s a SaaS cloud service. It allows you to do it in an intuitive and easy way.”
As more companies begin their big data journeys and uncover this unfortunate little secret, they’ll increasingly look to best-of-breed point products like those from Xplenty, Trifacta, Tamr, Paxata, and Progress Software to automate the transformation and cleansing process. They’ll have to, because a data scientist is a horrible thing to waste.
Related Items:
Automating the Pain Out of Big Data Transformation