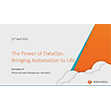
DataRobot ‘Guard Models’ Keep GenAI on the Straight and Narrow
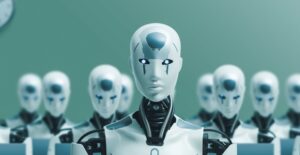
(Stokkete/Shutterstock)
Businesses are eager to deploy generative AI applications, but fears over toxic content, leaks of sensitive data, and hallucinations are giving them pause. One potential solution is to deploy “guard models” alongside GenAI apps that can immediately detect and prevent this kind of behavior. That’s the approach espoused by DataRobot, which today added new AI observability capabilities to its AI Platform that are aimed at preventing large language models (LLMs) from running amok.
In addition to a handful of pre-configured guard models, the DataRobot AI Platform gains new alerting and notification policies, new ways to visually troubleshoot problems and traceback answers, and new diagnostics to check for data quality and topic drift, among other capabilities.
It’s all aimed at alleviating the concerns that customers have around GenAI and LLMs, says DataRobot Chief Technology Officer Michael Schmidt.
“By far the number one thing we hear from our customers is this confidence problem, the confidence gap,” Schmidt tells Datanami. “A lot of them build generative AI systems and chatbots, but they actually don’t feel comfortable putting them into production because they don’t how they’ll behave. They don’t know where they break or how they’ll perform.”
The Web is full of stories of chatbots going off the rails. In early 2023, Microsoft’s Bing Chat Mode, based on OpenAI’s ChatGPT, famously threatened to break up a journalist’s marriage, compared the journalist to Hitler, and fantasized about releasing nuclear codes.
In addition to concerns about chatbots spouting toxic content, there is LLM’s persistent hallucination problem. LLMs will always make things up because of how they’re designed, so it takes a third-party to step in and detect the hallucinations. Then there are the implications of personally identifiable information (PII) potentially leaking out of LLMs, let alone people sharing PII with LLMs.
DataRobot has years of experience helping companies build, train, deploy, and manage machine learning models. For years, it sailed the seas of predictive analytics. When the GenAI tsunami arrived, the company quickly pivoted its wares to handling the new class of language models that have proved so promising, yet also vexing.
“That’s our number one focus, this confidence problem,” Schmidt continues. “Go talk to large organizations. What’s stopping them from putting more GenAI applications into production? You’re going to get something that’s related to ‘I don’t like the quality of it’ or ‘We need to improve the quality of it’ or ‘I don’t trust it’ or ‘I don’t know how well it’s going to behave under different scenarios’ or ‘I’m worried if it’s going to talk about competitors and I don’t have a good way to mitigate that. I’ll have to build a bunch of this really boring infrastructure myself if I wanted to do that and I don’t know what I don’t know.’ And we’re trying to attack that as respectively as possible.”
The new guard models DataRobot has introduced with in its platforms give customers a method for addressing some of the most pressing concerns. With its Generative AI Guard Library, the company now offers pre-built guard models that can detect prompt injections and toxicity, can detect PII, and can also mitigate hallucinations. Customers can also build their own guard models.
Some of the pre-configured guard models continually scan user input to prevent PII from being sent to the LLM. Other models guard against inappropriate output from the LLM reaching the end user’s eyes, including toxic content or even comparisons with competitors. When deployed alongside other new capabilities in the DataRobot AI Platform, the models can function as end-to-end guardrails for LLMs and entire GenAI applications, Schmidt says.
“We’ve also added an ability to do assessments and evaluation of not just the models and the pipeline, but actually the combination of guardrails you put together,” he says. “So how effective are they once you’ve combined different guardrails for the problems that you care about and for the grounding data you’re using to help answer questions?”
DataRobot can also generate test scripts and test prompts to determine whether the LLM is working as it should. If customers are using a vector database to store grounding data that’s fed into the LLM at inference time, DataRobot can use that, too.
“To me, that combination is very effective at really narrow in on trusting applications,” Schmidt says. “So now you can have safeguards in place and actually have visibility into their performance.”
This release also brings new feedback mechanisms that allow organizations to improve their GenAI applications. If a change to a GenAI model creates negative experiences for customers, that feedback is reported. The platform can then predict when other similar changes are expected to generate the same types of negative outcomes.
That’s part of DataRobot’s heritage in tracking model performance, Schmidt says.
“How well is your model performing? You can now use that to go evaluate your candidates for working AI systems that you have,” he says. “So if make an edit to a prompt now, you can see immediately what’s the acceptance rate, estimated acceptance rate metric, or estimated feedback metrics for that prompt. Or maybe you updated your vector database or maybe you swapped in Llama 3, swapped out GPT 3.5 or you made some sort of switch like that, and now you can actually measure what the effect is.”
While classic machine learning methods and predictive AI are still important use cases for DataRobot, the majority of new prospects are looking to implement LLMs and build GenAI applications. DataRobot is able to leverage much the platform it built for predictive AI for the new GenAI use cases, Schmidt says.
“That really helped us to go really big into GenAI quickly,” he says. “We had built up more and more capabilities for hosting and working with custom models, custom components. Even our MLOps platform, all that monitoring of drift and accuracy and features and feedbacks–you can do that with DataRobot models. You can do it with non DataRobot models. You can do that with remote model that are running on the edge or in some arbitrary environment with an agent.
“The value there is you have a single paint of glass to see all the deployments in one place, whether it’s on Google or Azure or DataRobot or something else custom,” he continues. “That flexibility also allows us to really quickly be able to support arbitrary unstructured models for generative AI workloads. To us it’s just another kind of custom model that we can natively support.”
DataRobot hosted a Spring ’24 Launch Event event today. You can watch it here.
Related Items:
DataRobot CEO Sees Success at Junction of Gen AI and ‘Classical AI’
DataRobot Unleashes 9.0 Update and Partner Integrations to Drive AI ROI