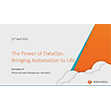
Google Cloud’s 2023 Data and AI Trends Report Reveals a Changing Landscape
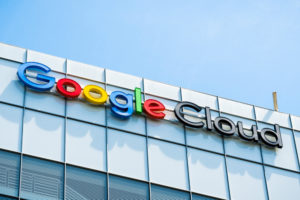
Google Cloud has released its 2023 Data and AI Trends Report that looks at 5 key trends surrounding data and AI strategies. The report notes that consumer demand, market conditions, and new AI and machine learning technologies have evolved, and increasing data complexity is creating a different landscape than even a year ago.
The IDC-conducted study surveyed over 800 global organizations asking them to name their biggest challenges in using their data, the benefits they are enjoying from data and AI cloud solutions, and where they are going next with these solutions.
Trend 1: Siloed data is out . . . unified data clouds are in. The report states that by 2026, 7 petabytes of data will be generated per second on a global scale. Currently, only 10% of that data is original, with the remaining 90% being replicated data. These stores of siloed data aren’t doing organizations any favors, and Google Cloud says a better way to store, manage, analyze, and govern this data is needed. The report explores how a unified cloud can be the solution, as it provides a common infrastructure to house databases, data warehouses and lakes, streaming, BI, AI, and machine learning.
Andi Gutmans, Google Cloud GM and VP of engineering for databases, says a unified data cloud enables integration of data and insights into transformative digital experiences and better decision making, and “as a result, users can have the right information, exactly when they need it, to reach the best possible outcomes.”
Trend 2: Open Data Ecosystems are helping companies avoid lock-in and siloed data by allowing data to move freely between platforms. Helping to speed up development and lower costs are pre-built, open source services and applications like PostgreSQL, Kafka, TensorFlow, PyTorch, Presto, JanusGraph, and Apache projects. On the other hand, the report notes that these open standards and open architectures can also help minimize data movement and egress fees by analyzing data where it lives.
Trend 3: We’re at the brink of an AI tipping point where managing data clouds and AI clouds separately is out, according to Google Cloud. Applications powered by AI are solving more problems and drawing more insights from data than ever before. June Yang, VP of cloud AI and industry solutions at Google Cloud says that data scientists, analysts, developers and ML creators are now closely collaborating, and they want a single interface where tools, data, and insights are accessible in a unified portal. The report notes that 80% of organizations say that having embedded support for AI/ML model execution makes them more likely to choose a particular data cloud platform.
Additionally, pre-trained models and low-code training methods are helping companies meet their AI and ML project goals by enabling citizen data scientists. The report found that 81% of organizations state that having more citizen data scientists would substantially improve their ability to apply advanced analytics to more projects.
Trend 4: Organizations are rethinking BI. They are leaving the traditional, dashboard-focused model behind in favor of adopting an action-focused BI paradigm where insights are served to more people in more environments to support more types of workflows, says Google Cloud. BI and analytics can help identify underlying trends, data anomalies, and underlying problems, and 87% of organizations want their BI software to support the development and deployment of predictive models. Embedding BI and analytics into enterprise applications is also on the rise, as organizations wish to reach a broader internal audience, as well as improve customer facing applications.
Trend 5: Data risk management is coming to the fore. Companies are getting to know their unknown data in order to improve security, governance, and trust. As more unstructured and structured data is collected, the need to know exactly what data is being collected is vital for knowing how to secure it and keeping it compliant. Manually finding, scanning, and classifying each data set for risk is challenging, especially for use cases like companies using customer chat applications where sensitive information could end up in chat transcripts.
Google Cloud says gaining visibility into all your data and understanding data ingestion pipelines and storage silos is the most critical step in data risk management. Next comes classification, and many organizations are using ML and business automation tools for this. Implementing automated controls can help reduce risk as data is stored and shared. For example, if customers will be providing sensitive data, an automated process can redact sensitive information before it is stored. Google Cloud predicts that by 2027, 66% of large enterprises will make major investments in data control plane technologies that can measure the risk inherent in data and reduce risk through security and screening.
Access the full report and Google Cloud’s companion blog post at this link.
Related Items:
Turning Quintillion Bytes of Data Into Opportunities
Why Truly Open Communities are Vital to Open Source Technology
Google Cloud Bolsters Storage with New Options for Block, Object, and Backup