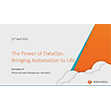
How ML-Based Data Mastering Saves Millions for Clinical Trial Business

(sfam_photo/Shutterstock)
As the country’s largest provider of clinical trial services, WCG has a sizable impact on the route to market for many drugs and medical devices. But as a conglomeration of more than 30 previously separate companies, WCG struggled to get a consistent view of data in support of those services. That’s where a data mastering solution from Tamr stepped in to help.
As a clinical services organization, WCG (WIRB-Copernicus Group) handles just about all aspects of a clinical trial on behalf of pharmaceutical companies and device manufacturers. From human resources and IT to patient engagement and ethical reviews, the Princeton, New Jersey company provides critical services to drug giants like Merck and Roche–as well and thousands of small and midsize pharmaceutical startups and research groups–that seek to gain regulatory approval of new drugs and devices.
Just about the only service the company doesn’t provide is running the actual trial. “We don’t do that,” says Art Morales, the company’s vice president of data and technology solutions.
WCG established the profitable niche in the clinical trial industry by acquiring 35 companies over the course of the past decade. Each of the companies–some of which are more than 50 years old–specialize in handling some aspect of the clinical trial process. The firms developed their own custom software applications to automate their various business processes, providing a very valuable source of intellectual property.
Having disparate systems makes great sense from the perspective of each individual business, but it poses a challenge to WCG, which desired a consistent view of operations across all of its subsidiaries. “We do some consolidation where it makes sense,” Morales tells Datanami. “But generally we say, look, just keep working as you are.”
Your Data Master
The company initially tried to hammer out the data inconsistencies by hand. A team of about five to 10 people worked for two years to root out instances of misspellings, duplicate entries, and other data errors contained in the disparate systems used by the 35 subsidiaries, Morales says. The cleaned, standardized data was stored in WCG’s data warehouse running in the cloud, where all manner of powerful analytic engines can be brought to bear on the data.
Much of the data mastering centered on properly identifying entities, such as customer names and locations. For example, determining what constitutes a “site” from data entered into a form or located in a database field is not always easy or straightforward, Morales says.
“One of the big problems we had was how do you identify that a site is the same site across different organizations?” he explains. “In some of the systems, it’s all free text. So I may have an address or I may not have an address, or the address may not be spelled correctly. And some of the data may just be missing, so I can’t just say ‘Only match things when I know exactly what I know and who matched this.’ There’s really a lot of uncertainty.”
Because of that uncertainty and the need to make decisions on a case-by-case basis, the process of mastering the data by hand was tedious and time-consuming. The company spent millions of dollars in support of data mastering, and the data still had inconsistencies.
“We got to a level that was acceptable, but we knew there were issues with the data,” Morales says. “It was slow, because it was one at a time. We were saying ‘OK, these two things are here, but we missed that. Let’s just make a hard link between those two entities because we know that we know it’s wrong.’”
Morales realized there had to be a better way. He heard about a data mastering tool from Tamr that uses machine learning to automatically identify known entities across large data sets, and he decided to give it a try.
ML-Based Data Mastering
Tamr is a data quality tool that emerged eight years ago from academic research conducted by renowned computer scientist Mike Stonebraker at the Massachusetts Institute of Technology. As the creator of Ingres (predecessor of Postgres), Vertica, and VoltDB, Stonebraker’s accomplishments are legendary (he has a Turing Award to his credit, to boot).
According to Anthony Deighton, the longtime Qlik executive who is now Tamr’s chief product officer, Stonebraker had the insight that machine learning was necessary to solve longstanding data quality problems, which are exacerbated at big-data scale.
“Five, 10, 15 years ago, the number one complaint that I heard out of analytics users is ‘I don’t trust the data behind this dashboard. I know it’s wrong. We’re looking at this report and I know we didn’t put the data from the Salesforce system in here, or this division over here, they used SAP,’” he says. “Or ‘We put all the data in the data warehouse and now we’re looking at the report, but I see 15 copies of IBM, all with slightly different versions of IBM’s name.’”
The prescribed solution to this dilemma for years has been to embark upon a master data management (MDM) project. Instead of relying on each individual system to get everything right all the time, the individual data systems instead would have pointers to a known good copy of the data – a golden record, if you will.
Tarnish on the Golden Record?
The golden record approach would solve the problem, or so they thought. However, the best laid plans have a habit of turning to dust once they meet reality. And this is exactly what happened with traditional MDM, Deighton says.
“I have yet to meet a customer who’s had a successful MDM deployment,” he says. “Relying on humans to clean and curate data is a fool’s errand. It’s never going to work.”
Stonebraker’s great insight into this problem was to use machine learning to catalog the data, in much the same way that Google used machine learning to automatically catalog websites on the early Internet, which trounced Yahoo’s effort to manually curate a map of the World Wide Web.
“The question that Mike and his PhDs were looking at was, if we imagine a world where we have thousands of tables of data, and the problem you were trying to solve was to understand business topic areas inside those data tables. Who are my customers? Who are my suppliers? What parts do we use in what products we sell? Who are our employees?” Deighton says.
“What was exciting for me is, as someone who spent so long on the front-end of the visualization and analytics question, that was a problem that I saw many of our customers struggling with and, frankly, having no real answer to how to solve,” he says.
By training a machine to recognize entities in business systems, Tamr has found a way to automate the creation of a golden record. A key insight that Stonebraker’s team made is that humans do much better when asked to confirm sameness with just a limited set of options, as opposed to dozens or hundreds of entries at once. “The term of art here is a bias to large clusters,” Deighton says.
So the MDM approach lives on–saved by the power of ML and a recognition of human bias.
Clinically Golden
WCG’s Tamr trial started in May 2021. After a training period, during which time the Tamr software watched and learned how employees handled data discrepancies, Morales set Tamr loose on WCG’s disparate data systems.
Today, a team of WCG employees work with Tamr to go through and cleanse all of the data sources feeding its data warehouse. The software identifies “clusters,” or two or more terms that mean the same thing across different applications, and those are loaded as golden records in WCG’s cloud data warehouse.
Each data source is run through Tamr before loading the data into the warehouse. The data source range in size from about 50,000 records to more than a million records, with perhaps 200 or so columns for each entity, Morales says. “It’s not in the volume–it’s in the complexity,” he says.
In addition to accelerating the data mastering process by about 4x, the Tamr tool is resulting in more standardized data, which means greater clarity into business operations, Morales says.
“As you clean the data, now you can actually use that cleaner data to get better operational insights,” Morales says. “We can match things across Salesforce and our applications to know that these are the right things. Before, if they had not been cleaned enough, you would match 50%. Now we can match 80%. So there are very obvious operational benefits to using what we’re doing.”
Tamr doesn’t successfully match all the entities into clusters. There are still edge cases that require the expertise of a human. In those situations, the software will let the operator know that it has low confidence in a match. But according to Morales, Tamr is very good at finding the obvious matches. He says the accuracy rate has been about 95% from day one.
“You have to accept any data mastering project there is going to be mismatches. There’s going to be Type I and Type II errors that happen,” he says. “If you can track where those errors are coming from….that’s perfectly fine. Because a human would have made the same error.”
As a side benefit, Tamr also helps WCG understand its data better.
“Sometimes we have brought things into Tamr, and we realize as we’re going through the process, ‘Oh, we actually should standardize those two into one, because those two fields that we didn’t know could be related, they are related, and then they could be standardized,’” Morales says. “So that that’s another benefit that we that we have seen.”
All told, the company has gone from millions of dollars of expense with its manual data mastering approach to less than a million with Tamr, Morales says. The improvements to data quality are harder to quantify, but arguably are more important.
“It’s not just about going fast. It’s about having this better and cleaner data that we can trust,” Morales says. “Every data you have, you have to treat with a grain of salt. It’s just how big that grain is. Now that grain is a lot smaller, because we’re really confident in the quality of our data.”
Related Items:
How ML Helps Solve the Big Data Transform/Mastering Problem
Breaking Down the Seven Tenets of Data Unification
Editor’s note: This article has been corrected. WCG is based in Princeton, New Jersey, not Puyallup, Washington. Datanami regrets the error.