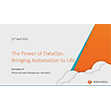
AIOps: Beat the DevOps Arms Race
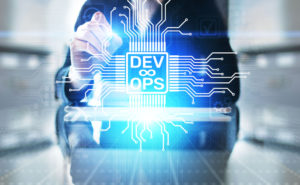
(Wright Studio/Shutterstock)
When we consider operational challenges in the technologi field, it’s tempting to think of them as a continual battle. We detect an issue, remediate it, and put improvements in place to prevent it from recurring. Detect, respond, adapt. This cycle is a powerful self-improvement model that allows organizations to keep up with their operational challenges as they scale and pursue their goals.
However, organizations like KPN, Google, COTY, and William Hill are learning how to break the cycle.
The Arms Race of Outages
This model of operational improvement in the DevOps world is an “arms race.” We improve, a new type of bug comes along, and we improve again. It doesn’t attempt to get ahead of unknown issues, because that isn’t part of the cycle, and how would we implement fixes and improvements for an issue we don’t even know about yet?
In the traditional method of operational improvement, we wait until our existing monitoring tells us that something has broken. This may take the form of a sudden spike in HTTP 500 errors from our API, or it could be error logs from our database server.
These errors tell us that something has broken. If we have already thought of this error, we might have alarms that tell us immediately. If we haven’t thought of this error, we might have to wait until our users tell us. That means we typically find out about an issue at the same time as our users, or worse… after.
This is where AIOps comes in.
What is AIOps?
AIOps leverages the immense power of artificial intelligence (AI) to detect issues. Rather than relying on alerts we already know about, AIOps offers observability that can detect anomalies in your system that you haven’t found.
It may be a sudden spike in logs from an application or an application that logs one error an hour suddenly fires 30 before settling back down again. All of these “quirks” could be symptomatic of a larger issue that you simply haven’t found yet.
The outcome of this constant analysis is simple. Rather than waiting until an issue has manifested itself in the form of an outage, you detect the subtle signs of a misbehaving system. Sudden changes in log volume, fluctuations in the number of background errors in an application, or a slowdown in latency that resolves itself. Traditionally, these things would be missed. AIOps visualizes and surfaces this data, so it can be examined and, quite often, result in actionable insights.
How Does AIOps Work?
The AIOps manifesto details five dimensions that align to form a valuable process of organizational learning. First, a dataset is detected. This is a combination of business decisions, upfront engineering effort, and the application of some selection algorithms to create a clear, useful set of data that can be analyzed.
Patterns are then detected in the dataset. The patterns might not link back to any business outcome. Possibly, some information has been detected as anomalous. These patterns are then run through the next stage, inference. Inference is the process of attempting to understand the causal relationship in the patterns that have been detected. This is the step that goes from a “pattern” to an “insight.”
These findings are then packaged up in the communication step. In this stage, the goal is simple. Transfer the knowledge from your machine learning algorithms into the minds of your engineers. This can be in the form of an API, a human-readable paragraph, or a letter in the mail.
The final and most complex stage is automation. In this stage, you seek to automatically remediate issues that have been detected. This is a complex problem. Many organizations find that the effort required simply doesn’t stack up to the value. Still, it is a fascinating vision and as the field progresses, no doubt this will become more accessible.
The Big Challenge with AIOps
Machine learning is hard. If you’re about to embark on your AIOps mission, you should begin by considering how much you want to build yourself. Rather than build it from the ground up, you can utilize SaaS providers that offer machine learning-driven observability.
How much do you need to be able to control your AI implementation? Do you want the results, or are you looking to embed machine learning into your technical strategy for years to come? This is not an easy question. For the vast majority of users, they want to reap the benefits without the painful learning. In this case, we strongly recommend that you use a SaaS provider.
So is AIOps Going to Change Everything?
AIOps is gaining popularity because our datasets and our observability challenges are growing beyond the limitations of traditional methods. That said, AIOps isn’t likely to replace your traditional alerts. Instead, it should be viewed as an upgrade. A safety net that catches the things you didn’t consider when you were designing your solution.
A fusion of traditional alerts for the “known” issues and AI-driven alarms for the “unknown” issues creates a phenomenal operational capability that will scale with your ambitions and maintain a stable, high-performing software system for years to come.
About the author: Ariel Assaraf is the CEO and co-founder of Coralogix, a provider of log analytics and AIops solutions.
Related Items:
Who’s Winning In the $17B AIOps and Observability Market
Coralogix Brings ‘Loggregation’ to the CI/CD Process