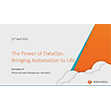
Putting Data to Work to Deliver the Best Care Everywhere
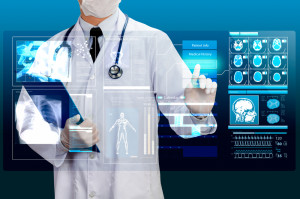
In healthcare, “big data” has been a topic of discussion since electronic health records (EHRs) were first introduced, spurring questions like, how is data stored? What’s the best way to organize it? And perhaps most importantly, how can it be applied to improve patient care? Today, clear-cut answers remain elusive.
What is clear is that vast amount of information being collected can and should be put to good use because fundamentally, data can play a vital role in helping healthcare deliver the best care everywhere. Advanced analytics tools – specifically those that leverage machine learning (ML) and artificial intelligence (AI) – have the potential to demonstrate data’s utility in a way that does not further burden already-taxed care teams. These tools can also help address the problems of equitable care and access to care in resource limited areas.
Data’s Healthcare Opportunity
Healthcare offers a unique use case for these technologies as data in this sector continues to grow exponentially. The human brain is limited in its ability to look at a given set of variables and understand how they interact, so there is a natural ceiling to the complexity of situations anyone – let alone a busy clinician – can successfully navigate.
In addition to the vast amount and different types of healthcare data being generated every day, the language of healthcare is largely unstandardized and unstructured, preventing widespread data sharing and restricting what can be understood across platforms and stakeholders.
Fortunately, advanced data analytics can evaluate a huge number of data inputs: lab results, white blood cell count, bilirubin, neutrophils, vital signs, medicine administration, concentrations and durations of meds, duration in hospital, demographics about the patients and demographics on a hospital—to name a few.
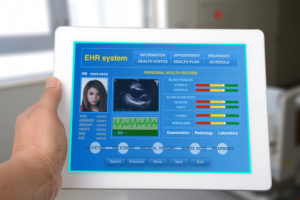
EHRs increase the variety of healthcare data that’s collected, but getting value from the data remains a challenge (pandpstock001/Shutterstock)
Machine learning can look at all these dimensions simultaneously mapping them to the answers needed by clinicians and other healthcare stakeholders, avoiding delays in treatment and improving outcomes for patients. In a clinical setting, the ability to extract these learnings can be lifesaving, providing an early warning or rapid diagnosis than would not be possible through manual processes.
Overcoming Obstacles to Broader Useability
The AI hype cycle would suggest applications for big data are seemingly endless yet, anytime multiple data sets, technology vendors, and human interventions are involved, there are bound to be challenges.
One of the greatest misses of healthcare IT, and the biggest hurdle for leveraging big data, is the lack of interoperability and compatibility among disparate systems, preventing it from delivering the most value it can for patients and providers. This becomes particularly apparent when health systems consolidate through mergers and acquisitions, creating siloed data that cannot be easily ported across providers or analyzed for a big picture view of the organization, or more importantly, for a seamless experience for patients.
Analytics tools sitting on top of EHR systems already help address this problem, pulling in multiple data sources and integrating them through an external system. Clinicians or administrators can then manipulate the data without altering anything in the original patient records. Applying AI to the data enables healthcare organizations to automate and scale the arduous process of mapping troves of fragmented data into something that makes sense and is cohesive.
For example, AI using natural language processing deciphers inconsistencies in EHR mappings for lab tests and then matches them to a commonly understood standard so resulting insights are actionable.
Data for Life-Saving Foresight
Another area where big data analytics is already delivering immense value is in infection prevention. Hospital infections, such as sepsis and Clostridioides difficile (C.diff), kill thousands of patients every year and cost millions of dollars. Infections like this can be hard to spot as symptoms stay under the radar until it is dangerously late.
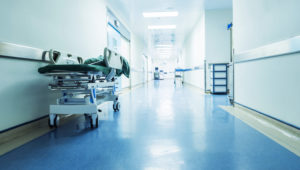
Hospitals have found some success using big data to monitor for infections, like sepsis and c.diff (hxdbzxy/Shutterstock)
It takes constant surveillance of patient data to prevent infections from getting out of control. Without technology, this involves scouring streams of routine results, tests, vitals, and observations from multiple patients. To actively surveil hundreds or thousands of patients across a health system, it becomes an impractical task. Healthcare teams are at their limits already, but big data tools that leverage AI and ML can automatically monitor data from patients to lessen the burden and strategically support care teams. Instead of asking providers to track yet another set of risk factors, technology can quickly audit patient data and flag areas critically needing intervention.
Hospitals like Tift Regional Medical Center in South Central Georgia hope to experience these benefits using an AI-supported sepsis surveillance tool with integrated workflows in their EHR. Sepsis is the deadliest and most expensive condition treated in hospital critical care units, with septic shock carrying a 34% mortality rate. Without exaggeration, early sepsis detection is critical to saving lives and stemming costly interventions.
Using sophisticated algorithms to continuously monitor the inpatient hospital population in real-time, the platform analyzes data including patients’ vitals, procedure documentation, patient demographics, medication orders, lab results–even parsing doctors’ text notes with AI-driven natural language processing–to alert clinicians to at-risk patients earlier so they can intervene with life-saving care. Tift Regional is set up for success to hopefully realize similar results validated in peer-reviewed study where sepsis-related mortality was reduced by 53%.
Enabling Equitable, Personalized Medicine
With clinician shortages and burnout on the rise, it’s increasingly challenging for providers to offer care beyond the appointment, especially with follow-ups. Unfortunately, healthcare settings in resource-limited areas are first in line to suffer in care quality.
We’re looking to technology tools like AI to extract insights from patient data and identify conditions like diabetes or high blood pressure for which engagement programs through phone check-ins, and even Alexa devices, can bridge some gaps in helping patients stay on track with better health.
The COVID-19 pandemic has highlighted the urgent need for industry-wide data connectivity and access, bringing together the entire healthcare ecosystem, including scientists, clinical researchers, hospitals, and outpatient settings. Smart technology platforms that incorporate the use of AI, ML, and NLP are an essential part of making this a reality and have proven their potential.
By leveraging these tools, hospitals and health systems can more easily surface trends in patient data, better identify patients at risk of complications, and establish sophisticated surveillance monitoring systems that increase efficiencies, reduce costs and, most importantly, save lives. Underpinning these efforts with a vision of achieving more health equity ensures we stay focused on the bigger goal of helping to deliver the best care everywhere.
About the author: Bill Flannery currently serves as Vice President of Advanced Technology Development at Wolters Kluwer, where he leads an AI Center of Excellence to support implementation, create best practices, provide training for business stakeholders, and support the envisioning of AI-enabled products. He has deep expertise in advanced technologies including Natural Language Processing (NLP), machine learning and deep learning for production application.
Related Items:
Why AI Has Yet to Deliver on Promises in Healthcare
How AI Boosts Human Expertise at Wolters Kluwer
Localized Models Give Hospitals Flexibility in COVID-19 Response