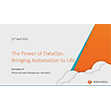
Is Your Data Safe? How To Assess and Reduce Your Data Risk
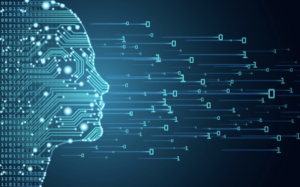
Data is one of any company’s most valuable assets — but it’s probably the one we understand the least. We have codes and inspections for physical infrastructure, we have satisfaction surveys for employees, we even have up-time monitors and stability tests for websites. But are we doing everything we can to understand the degree to which our data is exposed to risk?
There’s more to security than protecting yourself from hackers. On one end of the spectrum, you have those big exposures to governmental regulations and security breaches that can shake an entire organization. But even small things — like a little bit of bad data entering the system — can cause a trickledown effect that affects every department.
We could all be doing a better job of assessing (and mitigating) risk to our data. The key is to start small: Just make sure that you have the right data in the right place. Then you want to make sure that the right people have access to the data and the wrong people don’t have access to the data. Once you have that covered, and you’ve defined processes for keeping your data clean and standardized, then you can start focusing on making that a daily practice. All it takes is the right combination of people, processes, and technology.
What Do We Mean By ‘Risk’?
When most people think about the risks associated with data, they immediately recall the headline-grabbing data breaches that seem to flood our newsfeeds with alarming regularity. But it doesn’t take an epic leak affecting millions of users to have serious consequences for most companies. Even a handful of exposed records could have serious legal, financial, and reputational repercussions. Fines for GDPR violations alone can run in the millions of dollars, to say nothing of the incalculable cost of losing consumer trust in an increasingly connected and competitive marketplace.
How do these breaches happen? It can be something as simple as the right data in the wrong place. So much of our conversation about security centers around personally identifiable information (PII). If PII data isn’t identified or isn’t in the right field — for example, payment information erroneously mapped to an unprotected field and viewed by unauthorized individuals — you could be at risk of exposing some very sensitive information.
But external risks aren’t the only dangers we should be worried about. A few years ago, IBM famously calculated that bad data costs US businesses over $3 trillion per year. This is death by a thousand cuts, parceled out in seconds, minutes, and hours lost to manual data correction, re-running suspect reports, and pursuing strategies and programs that were originally scoped based on data that was later revealed to be faulty. Of course, the volumes of data we must deal with has grown by over 400% since IBM released that study — and it’s only growing. So how much could we be losing today? And how much do we stand to lose over the coming years?
Taking all these dangers together, one thing is clear: no company can afford to expose its data to risk.
What’s Involved In Risk Assessment?
When it comes to your data, there is no single magic bullet that can protect you from every scenario. But you can improve your overall data health by taking a closer look at the three aspects of data risk: sources, security, and compliance.
Data Sources
Understanding both the quality of individual sources and the quality of your data mapping is key to assessing your risk. When we talk about data sources, we have to consider not only where data comes from, but how it enters our systems.
For example, it’s probably safe to assume that the lead list you purchased from a vendor isn’t as accurate or up-to-date as the list of leads you captured from a recent, targeted, double-opt-in campaign. But even if you could 100% trust the accuracy of every record from every source — including manual entry by salespeople, submissions from any range of online forms, engagements within products or mobile apps, and shared data from partners or parent companies — you would still be looking at a multiplicity of fields, standards, and definitions across sources. One source may require a country code in the phone number field, while another does not. One source may have a single name field, while all the others break out first and last names.
Getting these sources to all speak the same language (so to speak) can be a challenge in and of itself, but it is well worth the time and consideration. Fortunately, there are technologies available that will automate data quality as part of the data integration process, so you can avoid risk with the steep time investment of manual data correction.
Data Security
If all your data were collected in a single Excel spreadsheet, it would be pretty easy to assign a person or two to watch over that data, to keep it secure, and to validate it, line but line. But that’s not the world we live in. For most of us, the landscape of our data infrastructure is a complex network of interconnected programs and platforms. There are obviously tools that specialize in connecting systems and ingesting data into a repository. And some businesses have success just doing that — but are they really getting a true sense of data health? Would they even know if they had data quality issues?
The first step of data security is securely connecting to our data sources, ingesting the data, and performing that first pass of data quality checks to ensure that we’re getting the right data in the right fields. Next, data profiling technology can help us make sure that phone numbers look like phone numbers, and emails look like emails, and so on, so we can feel safe that we haven’t miscategorized sensitive information. Some profiling technologies may even be able to automate resolution for common data errors.
After that, it’s time for people to get involved, so the data experts can manually correct, reconcile, and validate any records that cannot be confidently evaluated by the automated data quality tools. Proper processes and workflows need to be in place so that the right people can look at it in a formal way. This will require technology for data inventory, data stewardship, and data preparation.
Compliance
Good intentions — even good intentions backed by good technology — can only take you so far. A recent study by the UK Information Commissioner’s Office (ICO) discovered that up to 90% of data breaches can be traced back to human error. Believe it or not, this is good news — back in 2015, IBM reported that a full 95% of data breaches were caused by human error. So… progress, I guess?
Technology — including our own Data Catalog — can help here by providing a centralized infrastructure for managing and ensuring compliance across the organization. These products allow you to establish clear access protocols and permissions that will protect your data, without creating false barriers to access that might make people less effective at their jobs. They also make it possible to automate the classification of data through semantic types and build a well-defined business glossary, so that everyone speaking the same business language when it comes to your data.
How to Reduce Your Data Risk
If you try to do everything at once, you’ll burn yourself out. Instead, take it slow and go one step at a time. Start by making sure that you’re getting good, trustworthy data into the system. Then you can build out the people, policies, and programs you need to keep that data healthy for the long haul.
Step 1: Data integration
The easiest way to protect yourself from compromised data is to make sure that it never enters your systems in the first place. Ideally, you will want to set up automated checks for data quality as part of your ingestion process.
- Prioritize your data sources. Some are more trustworthy than others, so you’ll want to make sure to choose the sources that provide the most value. And it sounds obvious, but you should always make sure that any data ingestion or migration should be done via a secure transfer protocol.
- Collect your data. Whenever possible, bring your data together into a data lake or data warehouse. Centralized data will be easier to monitor and manage than data spread across a range of systems and departments.
- Profile and cleanse your data. Check from incomplete or inaccurate records, remove duplicates, and make sure that every field of every record is correctly mapped and labeled.
Step 2: Data Governance
Data governance is the collection of processes, roles, policies, standards, and metrics that ensure the effective and efficient use of information in enabling an organization to achieve its goals. The specifics of data governance will vary from company to company, but usually there are at least three groups involved:
- IT (or data engineers). This is the group who collects the data, builds the process, and makes the data available within the organization.
- Data stewards. These are the people who really understand the data, not just as pure data points, but how it will be used by the business. They will review the data and make sure it can be used and trusted.
- Business users. These are the consumers of data, from analysts to department heads, from the C-suite to individual contributors. There should be clear rules and permission settings that determine who has access to the data, and when and how they can access it.
Step 3: Automation
Unless you’re keeping critical data in a simple spreadsheet — which would be a really inefficient way to do business — you’re going to need technology to automate the repeated tasks of managing your data.
The heavy lifting will come from IT, as they set up technology and rules that will automate data integration, data quality, data preparation. From there, governance and workflow processes can all work together. If something can’t be automated, it goes through a formal review process with the data stewards.
Once you get that initial process defined and outlined, it’s not so much an exercise as just business as usual. As new data comes into the organization, defined processes automate the cleansing, enriching, and standardization of the data. Whatever data can’t be confidently conformed through automated means gets sent through defined workflows and rectified by those that know the data best. This becomes the natural lifecycle of data in your company.
That may sound utopian, but you don’t have to do it all at once. It can take time — and maybe a shift in mindset — but it is possible. Once you have that practice, like a muscle, it will grow stronger the more you exercise it.
Protecting Yourself From Risk
Your data is too important to leave anything to chance. It will take a balance of people and processes, supported by the right technology and automation, for you to keep up with the never-ending flow of data through your company. In a perfect world, we would all have top-of-the-line security solutions and 100% compliance with every piece of advice from the IT team. But, even in this imperfect world, we can make significant progress.
If you’re getting ready to make a change, start small: make sure that your data is standardized, cleansed, and adheres to whatever standards you have. Solving the problem of compromised data sources will have a ripple effect throughout the organization, making everyone more effective and efficient, and freeing up resources to devote to larger data issues.
About the author: Felipe Henao Brand, senior product manager at Talend.
Related Items:
Multi-Cloud Complexity Heightens Security Threats
Navigating Data Security Within Data Sharing In Today’s Evolving Landscape
Why Security Pros Need To Think Like Data Gurus