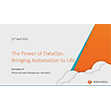
A Peek At the Future of Data Management, Courtesy of Gartner

(Yusak_P./Shutterstock)
Managing big data has gotten easier in some ways, thanks to better tools and technology. But it’s still a sizable challenge, especially when maintaining privacy and security in data-dispersed environments. So how will we tackle these challenges in the future? The smart folks at Gartner have some ideas, which they shared during last week’s Data & Analytics Summit.
According to Gartner analyst Mark Beyer, the cavalry will soon be here (if it’s not already) in the form of machine learning- and AI-powered data management automation.
“Our machine partners are now actual peers in operations for data management,” Beyer said during his sessions, titled “The Future of Data Management.” “Machines do certain things far more efficiently and much faster than humans do.”
Machine learning algorithms currently exceed human ability in areas like pattern-matching, categorization, and anomaly detection. One of the most promising areas where our machine partners can help us is improving data quality, including data profiling.
“Over the next three years in data management, we’re going to be teaching our machine partners quite a few things,” he continued. “But machines can only go so far. So just like in your peers of human partners, your machine partner are going to have to recognize how much they can do and where they have to stop.”
Getting our machine partners to know when to stop won’t be an easy task. Eventually, the machines will get better at adapting to difficult circumstances – that is, to improvising based on learned experiences – and that could very well mark a turning point in the history of data management.
“We have to teach our machine models when different pieces and parts can be reused in different combinations,” Beyer said. “And we also have to teach them how often those combinations change, the rate of those changes, and when one pattern actually reflects a…new pattern. That’s the definition of improvisation.”
Instead of letting machines experience failure and then asking them to adapt to it, which Beyer called “the school of hard knocks,” the future of data management will entail a meticulous transfer of skills from humans to the machines.
“At some point we’re going to have true augmented data management,” Beyer said. “And in true augmented data management, what happens is the humans sort of surrender the improvisational role.”
Beyer recommended that users look to adopt tools that can provide continuous data profiling capabilities that can automatically discover new data. Companies should look to tools from cloud providers or ISV tools that share open metadata standards, he said. He also recommended that companies adopt a data fabric that can automate metadata analytics across multi-cloud environments.
Data Hubs
Another way to alleviate the burden of data management is through the use of data hubs, according to Beyer’s colleague, Gartner analyst Ted Friedman.
“Your application and data landscape probably has grown quite vast, highly distributed, very diverse, siloed, and with constantly changing data throughout,” Friedman said during his session on data hubs at last week’s Gartner’ conference. “These are conditions that obviously are not conducive to fast, reliable, effective, trusted data flow.”
Forcing users to abide by the types of heavy-handed governance that IT folks implemented in years past will not work in the new data paradigm, Friedman said.
“You’re never going to be able to keep up with the ever-growing sprawl, and the increase in complexity,” he said, “and you’re never going to be able to make all system and all the people in the organization behave according to your expectations.”
One approach that could work is adopting a data hub strategy. According to Friedman, a data hub shares some similarities with data lakes and data warehouses. But there’s a key difference in the data hub approach that enables it to work where massive lakes and warehouses turn into ungodly messes.
A data hub is “an architectural pattern that enables the mediation, sharing, and governance of data flowing from points of production in the enterprise to points of consumption in the enterprise,” Friedman explained. A data hub will provide “a point of mediation, a place where producers and consumers of data can meet…and share data and share good governance along the way.”
The data may or may not be persisted in the data hub, and enterprises may have a single data hub or many different data hubs for different use cases. There could be a data hub for a master data management (MDM) project or another one for analytics, Friedman said.
“Data hubs basically have two facets, or two dimensions,” he said. “One is about integration about the flow, the sharing, the movement, the synchronization, the provisioning of critical data. The other is about the governance of data – doing the right thing from a quality, privacy, security perspective.”
At the end of the day, a data hub is designed to help an enterprise break data management challenges down into more easily digestible pieces, Friedman said.
“A data hub strategy is going to help you to take out a lot of that complexity and simplify and streamline data flow in the enterprise and increase the levels of trust,” he said. “In essence, data hub is the antidote. It’s the thing that’s going to help you capture the benefits of common understanding of data, reduce the cost and complexity of integrating infrastructure, and help you get back a little bit of control–hopefully a lot of control–from a governance point of view.”
Data historically has demanded concessions from the companies that would collect it, analyze it, and put it to use in high volumes. While technology has helped companies overcome some of those challenges, the continuously changing data landscape demands that companies recognize new challenges, and identify additional approaches to remediating them.
Related Items:
Drowning In a Data Lake? Gartner Analyst Offers a Life Preserver
Data Management Self Service is Key for Data Engineers–And Their Business