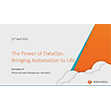
Drowning In a Data Lake? Gartner Analyst Offers a Life Preserver
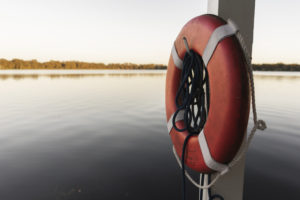
(John-McLenaghan/Shutterstock)
Gartner this week convened its annual Data and Analytics Summit Americas conference, which was held online again due to the coronavirus pandemic. From the role of AI in data management to avoiding data lake failures, Gartner analysts shared a host of useful knowledge.
Let’s start with data lakes, which in recent years have become popular repositories for storing massive amounts of data. First starting with Hadoop and now more recently with cloud-based object stores, companies have found data lakes to be efficient places to park petabytes of data.
But this new data lake paradigm has not been without its challenges, and many companies have watched as their pristine new data lakes turn into murky, muddy data swamps. For Gartner analyst Donald Feinberg, it’s like déjà vu all over again.
“We had the same situations with data warehouses back 10, 15, 20 years ago,” Feinberg said during his “How to Avoid Data Lake Failures” session on Tuesday. “For years and years and years, everybody tried to put everything in one place with the data warehouse, and that didn’t work. Well, it doesn’t work with a data lake, either.”
So, how can data lakes be saved? It turns out that some of the same techniques that Gartner advocated for saving data warehouse projects over a decade ago can also save data lakes. Some things, apparently, never go out of style, even in big data.
Feinberg’s first piece of advice was to avoid getting into huge implementation projects, which continue to be the bane of IT’s existence. “First of all, stay away from the big bang theory,” he said. “Don’t take on a five-year project to throw everything into one big data lake.”
Instead, start small, preferably implementing a data lake for a single business unit. Just like companies in 2005 were advised to implement smaller data marts rather than giant data warehouses, the smaller data lake projects have a better chance of success.
“But remember, it is a component of the whole picture, just not the whole picture,” Feinberg advised. “You’re going to need multiple [data lakes]. You’re going to need it to work in conjunction with other things, like maybe even a data warehouse.”
Plus, don’t conflate a data lake with having a data and analytics strategy. A data lake may be part of the D&A strategy, Feinberg said, but a data lake, in and of itself, isn’t a strategy.
“What happens is organizations get new executives, like a new chief data officer,” he said, “who says ‘We need a new analytics strategy’ and that’s to create a data lake. That is not the way to do it.’”
Feinberg also recommended that data professionals keep the prospective business value of the data lake project front and center in their minds. If you can’t identify what actual business value the data lake will bring to the business, you may be fishing in the wrong lake.
“People pretty much understand the value of data,” he said. “But they don’t look at the business value of the data lake or of the whole data and analytics strategy.”
Data lakes are generally quite flexible in terms of the data that can be stored in them, especially if they’re based on non-relational technology, such as Hadoop or object stores (which they don’t have to be, Feinberg advised).
However, that can lead to hoarding-like behavior, where companies start parking all kinds of different data of questionable value into the data lake. This tendency to dream of an “infinite data lake” generally is not a good thing, particularly when it comes to data governance and related issues of security, privacy, and regulatory compliance, Feinberg said.
“When you start bringing data from anywhere, all different types of data, you have a real issue with governance that’s going to hurt you, and hurt you badly,” he said. “You have no idea what the data is you’re bringing in and governance becomes really important.”
The solution to that challenge is to be more vigorous in ferreting out the data that doesn’t belong there, including getting rid of old data that is getting stale and may bring liability issues by continuing to store it.
Moving to the cloud, with its unlimited scale for storage and compute, may seem like a potential solution when on-prem data lakes start bogging down. But there are important caveats to keep in mind with the cloud, with Feinberg said is by far the most popular place where Gartner clients want to store data these days.
“In six seconds you can just add resources,” he said. “Some systems do it elastically, automatically for you. Just be careful when you get the bill at the end of the month because your CFO is going to have a heart attack.”
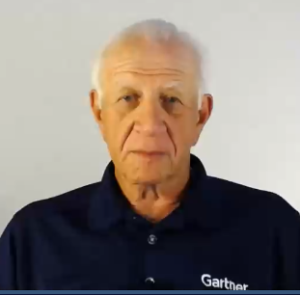
Donald Feinberg is a vice president and distinguished analyst in the Gartner ITL Data and Analytics group
When the problem is too much data in the data lake, you can’t fix the problem by just throwing resources at it, Feinberg said. “I caution you when you move to the cluod. That’s not a Band-Aid to fix the problems with data lakes.”
But above all, the number one way to address the data lake problem, according to Feinberg, is by fixing deficiencies in skills. This was how companies pulled themselves out of the data warehousing morass 10 to 20 years ago, and it’s eventually how companies will pull themselves out of self-made data swamps.
“Why were data warehouses suddenly successful?” he said. “The talent gap was closed.” The same will also work for saving data lake projects from failure, along with resetting expectations, using better tools, and following best practices.
Feinberg also recommended taking a look at relational databases as data lake repositories.
“Remember that data lakes do not have to be on a non-relational Hadoop environment. You can build your data lake on a relational database,” he said. “Many of the organizations we talk to, 90% of the data they’re putting on their data lake is structured relational data. So why not put it into a relational database?”
Now there’s a novel idea.
Related Items:
Cloud Data Warehouses and Cloud Data Lakes: There’s No Need to Choose
Overcoming Obstacles to Data Lake Success
To Centralize or Not to Centralize Your Data–That Is the Question