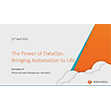
ML Scaling Requires Upgraded Data Management Plan
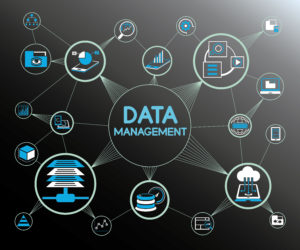
Successful data strategies are built on a foundation of meticulous data management, creating enterprise architectures that “democratize” data access and usage, yielding measurable results from machine learning platforms.
The reality, according to an examination of the emerging “AI organization,” is that few data-driven organizations are able to deliver on their data strategy. A survey commissioned by Databricks and conducted by MIT Technology Review Insights found that a mere 13 percent of those polled actually achieve measurable business results.
MIT Technology Review Insights said it polled 351 CDOs, chief analytics officers as well as CIOs, CTOs and senior technology executives. It also interviewed several other senior technology leaders.
The shift to cloud-based platforms, including databases and analytics tools with machine learning capabilities, is offset by legacy systems and the resulting data silos.
“Fragmentation of architecture is a headache for many a chief data officer, due not just to silos but also to the variety of on-premise and cloud-based tools many organizations use,” the MIT survey concludes. “Along with poor data quality, these issues combine to deprive organizations’ data platforms—and the machine learning and analytics models they support—of the speed and scale needed to deliver the desired business results.”
One consequence is the inability to scale machine learning use cases. The biggest challenge, more than half of respondents said, is the current lack of a central repository for discovering and storing machine learning models.
That disconnect contributes to the inability to push AI workloads to production, suggesting “severe difficulties in making collaboration between [machine learning], data [science] and business-user teams a reality,” 39 percent of respondents said.
What’s to be done? The survey predicts an accelerating shift over the next two years to cloud-native platforms better equipped to support data management—especially growing volumes of streaming and unstructured data—thereby boosting data analytics and machine learning capabilities and the data strategies they support.
Along with cloud migrations, data managers struggling to forge new architectures that advance machine learning cite the need for open data formats and other open-source standards.
Study sponsor Databricks used the results to promote its “lakehouse” architecture unveiled last year that incorporates real-time streaming, batch processing, SQL analytics data science and—last but not least—machine learning.
The MIT study “suggests organizations need to build four different stacks to handle all of their data workloads: business analytics, data engineering, streaming” and machine learning, the Apache Spark creator said.
“All four of these stacks require very different technologies and, unfortunately, they sometimes don’t work well together.”
The MIT survey’s “highest achievers” in terms of effective data strategies were financial services firms and, surprisingly, the government and public sector. Among the keys to success were reduced data duplication, ease of data access, fast processing of large data volumes and improved data quality.
Recent items:
Databricks, Partners, Open a Unified ‘Lakehouse’
Will Databricks Build the First enterprise AI Platform?
Machine Learning Hits a Scaling Bump