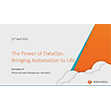
Empowering the Data Consumer: Living, and Breathing Data Governance, Security, and Regulations
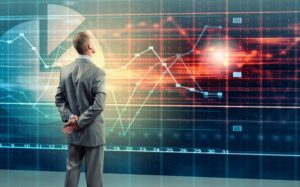
(SB Professional/Shutterstock)
Every organization wants to leverage their data as their strategic asset, scale data usage while effectively delivering speed, self-service, and ensuring security. To meet the growing demand for analytics in large enterprises, an automated approach to data privacy and governance is critical.
This poses a great opportunity for data professionals to develop an innovative enterprise-wide data strategy approach to disrupt existing labor-intensive, and complex legacy processes by ensuring automated Data Access Governance (DAG) without sacrificing core architecture guiding principles.
For years, enterprises have been bringing together data sources to expand data ammunition in the name of innovation. Along the way, things have gotten complicated with exponential data growth leading to environments where centralized governance and consistent security can be challenging and lead to slow data-sharing processes that create opportunities for data leaks. At the same time, while enterprises are still under pressure to “move fast,” regulations like the General Data Protection Regulation (GDPR) and California Consumer Privacy Act (CCPA) must be followed to protect the consumer and clients.
Key Business & IT Challenges
While data usage is expected to scale through the cloud-based data and analytical platforms, organizations must strategically drive the conversations, develop processes, and adopt technology capabilities to build trust, drive business value, and reduce data-related risks.
To drive an enterprise-scale solution, organizations should look to overcome these challenges as an initial step:
- Privacy and design: The inability to achieve consensus on the definition, and priorities around data security/privacy as an organization including legal, privacy, infrastructure security, data access management, and engineering teams;
- Discovery: Lack of end-to-end data mapping across the landscape including data relationships, critical patterns, and data movement across systems
;
- Data Catalog: The absence of a unified view of all data sets across on-premises, cloud applications and other data stores which inhibits data collaboration across teams;
- Security: Challenges around implementation of robust security controls due to lack of data tagging, classification, and other foundational metadata items needed for sensitive data identification/protection;
- Self-service: The inability to empower analytical teams by providing seamless access to both internal and external data without too many process burdens;
- Fine-grain security: Challenges around implementation of data privacy, and compliance policies across regions, systems, and data lifecycle while ensuring consistent performance;
- Audit: Data audits that do not paint a clear picture around who has access to data, when they’ve accessed and where; and
- Operating Model: The perception of security teams as “roadblocks” standing in the way of easy-to-use tools for data privacy and security for non-IT users. No clear ownership, and processes for like access, sharing, rights, policies, quality, retention, etc.
But these challenges don’t have to be a breaking point anymore.
When organizations are faced with challenges around data privacy/security, a choice has to be made between what type of data platform they want to fuel their business, operational model around data, and approach to data governance.
Top-Down vs. Bottom-Up
A top-down approach to building data and analytics platforms, based on data governance best practices and policies, is often the choice. This approach can provide a cohesive and robust solution that complies well with privacy regulations, and where all the components interact well, adhering to strict security policies. Unfortunately, it can often become cumbersome for users and slow the time-to-value, with data consumers forced to adapt their data usage and consumption to the strict compliance and security-driven protocols driving the platform.
On the flip side, a bottom-up approach to data analytics is engineering and design-focused, with the goal of introducing incremental deliverables that add value to the platform in response to the user’s needs. The advantage is that value is realized early and often, because it allows architects to adopt the best of breed for each subject area. The downside is that integrating components incrementally might result in poor integration and technical difficulty securing the platform and complying with regulations.
Whether top-down or bottom-up, it’s critical for organizations to start with documenting privacy, security, data risks, controls, and technology needs around data access to address topics like culture of federated data ownership, adoption of self-service or collaboration across teams around critical data sets, and enterprise-wide technology standards for certain key areas. This quantitative approach helps with building enterprise data platforms that have privacy and security as a foundational layer that is risk and controls-based.
Aon’s Story: Self-Service Data Access & Automated Security
Data is a key strategic asset at the core of our business. It’s the secret sauce that helps us thrive and gain a competitive advantage, so the need to manage, maintain, and protect it is absolutely critical. There is no one solution out there that can address the complexity of our privacy and security needs, so data security and privacy must be baked into the design process, with automated controls.
At Aon, we have a large community of data consumers, engineers, and scientists spread across the globe, who glean insights for clients from millions of data sets while complying with restrictions related to compliance, privacy, and regional/industry regulations (e.g. Health Insurance Portability and Accountability Act [HIPAA], General Data Protection Regulation [GDPR], Personally identifiable information [PII], and the California Consumer Privacy Act [CCPA]).
We have navigated privacy, security, and compliance challenges by architecting a multi-region and multi-tenant cloud-based data and analytics services platform which offers:
- Enterprise platform which is built based on public cloud with data lake as center piece, where existing data privacy/security controls can support multiple tenants, and clients across regions;
- Democratized global data catalog with thousands of data sets with enriched metadata, tags, ownership, and classifications which support our data community to search, browse, and request access in an automated manner;
- Empowerment of data owners who manage, and control access to their data without IT intervention using Immuta‘s automated governance product. This allows us to achieve automated data governance and advanced data privacy administration by applying global/local policies leveraging metadata tags, and data classification from data catalog;
- True self-service of data for a community consisting of data scientists, data engineer, data analysts, and business user by providing access to easy-to-use data preparation tools along with isolated data landing zones for data storage;
- Multiple capabilities around large-scale data processing mechanisms including traditional cluster computing programming, and public cloud native capabilities;
- Cloud-based architecture and design which supports advanced digital product and application development using data, and analytics platforms as the backbone; and
- Training ground for advanced analytics around machine learning, artificial intelligence leveraging agile tools, and auto-scale with containers with native security.
Our Approach
Our ultimate goal is to enable and empower our teams with tools allowing self-service access for data analysts, data scientists and line of business users so data can be safely used, shared, and distributed based on business need, and without any technical hurdles.
When data architects face a proliferation of rules that need to be implemented to process sensitive data, it can be difficult to enforce those rules in a scalable way. This is largely because they are driven by the security/compliance team in response to ever-changing rules around data use from data protection laws, data use agreements, employment laws, intellectual property controls, etc. The rules are not created from a user-centric perspective. As a result, data teams will often receive a list of requirements that, while effective in their ability to protect data and adhere to regulations, will also disrupt platform operations.
From a privacy and security standpoint, it’s a big commitment for those data teams. We need to architect and design our platforms based on global compliance needs and local laws, ensuring that it can scale to handle any future privacy/compliance needs while meeting all the necessary security controls, such as fine-grain access controls, global/regional data policies, data tagging, data masking, data classification and auditing. We have put into place robust processes, and reinforced deep alignment across data architecture, cloud engineering, network security, and platform security architecture teams, ensuring that we have reviewed data flows from end to end – from data discovery to data distribution.
All of this is designed to embed privacy into the DNA of our data security practices, but buy-in and participation from data consumers is also incredibly important. Right from the onboarding stage, data owners should go through extensive training and are held accountable for their actions – and security teams should take into account their concerns about usability.
In addition to data access governance, we need to match it with extensive processes, operational tasks, periodic reviews, automated alerts, and automated steps to identify discrepancies. Even with this level of meticulous planning, gaps will arise. That’s when we turn to partners like Immuta to scale user adoption with increased granularity and automation for fine-grained access and privacy controls, including centralized auditing and monitoring, dynamic policies based on both role and attribute-based access controls leveraging metadata from our enterprise data catalog, and to define entitlements and data access policies for heterogeneous data sources in our analytics infrastructure on public cloud.
To meet the goals of data-driven organizations, to disrupt your existing business model, or to promote innovation at scale, there needs to be embedding of data platforms, collaborative user communities, self-service technology capabilities, and robust data privacy/security processes. While there is no single solution currently available to manage data privacy, access, security, governance, compliance, and audit capabilities, these three pillars – privacy/security by design, technology partners, and strong internal processes – are essential to support consumers, and turn data into a differentiator.
About the author: Srinath Reddy is currently Head of Data at Aon, and part of Global CTO office. He has extensive expertise in building advanced analytical platforms leveraging public cloud.
Related Items:
Making it Real: Effective Data Governance in the Age of AI
Building a Successful Data Governance Strategy
Not All Data is Created Equal: Why Companies Need a Data Governance Strategy to Succeed