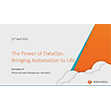
It’s Time to Implement Fair and Ethical AI
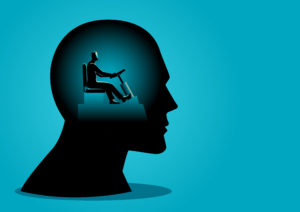
(rudall30/Shutterstock)
Companies have gotten the message that artificial intelligence should be implemented in a manner that is fair and ethical. In fact, a recent study from Deloitte indicates that a majority of companies have actually slowed down their AI implementations to make sure these requirements are met. But the next step is the most difficult one: actually implementing AI in a fair and ethical way.
A Deloitte study from late 2019 and early 2020 found that 95% of executives surveyed said they were concerned about ethical risk in AI adoption. While machine learning brings the possibility to improve the quantity and quality of decision-making based on data, it also brings the potential for companies to damage their brand and reduce the trust that customers have placed in it if AI is implemented poorly. In fact, these risks were so palpable to executives that 56% of them say they have slowed down their AI adoptions, according to Deloitte’s study.
While progress has been made in getting the message out about fair and ethical AI, there is still a lot of work to be done, says Beena Ammanath, the executive director of the Deloitte AI Institute.
“The first step is well underway, raising awareness. Now I think most companies are aware of the risk associated” with AI deployments, Ammanath says. “That has been the first step. The second step is really about driving adoption….[and stating] ‘these are the ethics rules that your organization is going to follow in the context of AI.’”
Trustworthy AI
In August, Deloitte published its Trustworthy AI framework to serve as a guide to help companies implement fair and ethical AI. Every AI deployment is different, and carries different risks. Nonetheless, Deloitte has boiled the risks down to six broad areas and issued broad recommendations to address this.
The six components of Deloitte’s Trustworthy AI framework include:
- Fair and impartial use checks. Biased algorithms and data can lead to unexpected outcomes for customers. To avoid this outcome, companies need to determine what constitutes fairness and actively identify biases within their algorithms and data.
- Transparency and explainability. Companies should be prepared to explain how their algorithms arrive at their decisions or recommendations.
- Responsibility and accountability. Companies should have a clear and open line of communication with customers about their AI systems, and should clearly delineate who is responsible for ensuring fair and ethical AI.
- Security and safety: Trustworthy AI should be protected from risks, including cybersecurity risks that could lead to physical and/or digital harm, Deloitte says.
- Robustness and reliability: The systems, processes, and people involved with implemetning AI should be as robust and reliable as the traditional systems, processes, and people that it is augmenting, Deloitte says.
- Privacy: Protecting the privacy of personal customer data and ensuring that this data is not leveraged beyond its intended use, are fundamental aspects of trustworthy AI.
Not every company will need to implement each aspect of the Trustworthy AI framework, Ammanath says. For example, a manufacturing company that’s using computer vision algorithms to determine the quality of parts on the factory floor may not worry about bias in algorithms. However, a retail company that deals with consumers’ personal data will absolutely need to take algorithmic bias into account.
“We started this framework to have a broad discussion to say, these are the different things you should consider in context of AI ethics,” she says. “We don’t expect every organization to take the framework as is and say, okay, come and implement it.”
While executives ultimately will be responsible for their companies’ adoption of fair and ethical AI, everybody in the company needs to participate in driving change, Ammanath says. That’s something that Deloitte is actively doing, she tells Datanami.
“We’re….training our entire workforce how to think about ethics,” she says. “I don’t think that ethics is one person’s job in an organization. Every employee has to be empowered to think about the ethical implications, and what Trustworthy AI provides a framework of the different nuances around it.”
We are still very early in the process of setting the guidelines for fair and ethical use of AI, and there are not a lot of hard and fast rules. Eventually, different industries will begin to set their own guidelines for what constitutes fair and trustworthy AI for their particular industry, Ammanath says. A similar dynamic will unfold around particular technologies and use cases, such as facial recognition, she says.
“I think we’re going to see more technology specific nuanced policies coming up as we mature in the third stream of AI ethics,” she says.
Protecting Privacy
One man who takes AI ethics very seriously is Pankaj Chowdhry.
As the CEO of FortressIQ, Chowdhry has led the development of computer vision technology that model a company’s workflow by monitoring all of the screens that an employee uses, and mapping the flow of those screens across applications.
The potential for abuse is quite high with that AI, specifically as it relates to snooping on employees. That’s why FortressIQ invested so much time in building controls into the software to ensure that it is not abused.
“We can observe every single interaction going on across your entire organization at a scale that couldn’t even have been imagined 10 years ago,” Chowdhry tells Datanami. “We said, okay, just because we built the technology to observe this, we have to build the same level of technology to protect privacy.”
To that end, FortressIQ developed AI methods to identify when an employee is conducting their own person business on their employer’s computer. That includes building computer vision technology to identify whether an employee is visiting a site like Facebook. It also involved using natural language processing (NLP) technology to determine when an email is a private email, and to essentially mask the details or drop it from FortressIQ’s surveillance system.
“We want to focus on the process, not necessarily the person,” he says. “So what can we do in order to do that? We can turn it into big data. We can say, we’re not going let you to look at the individual level. We’re going to aggregate the data so you can’t do that. We’re going to say, if it doesn’t’ happen statistically significantly, we’re going to drop it automatic. It’s not even going to get into the data set at all. Those are the sorts of things [we’re doing] to try to make sure that an individual’s privacy and their agency is respected, at the same time we’re trying to optimize a business outcome.”
Protecting people’s privacy is one of the most challenging aspects of living in our digital age, and FortressIQ’s approach is to be commended. Unfortunately, in today’s surveillance economy, there are many bad actors that collect data they have no business collecting, and consumers are never the wiser.
“Every signal that you get can make AI better, and there’s often a business reason to do that. But you really do have to weigh that with the privacy implications of it and then whether they’re going to be good stewards of the data,” Chowdhry says. “It’s the idea that, if you build something that has this incredible potential to be used badly, then you have to spend the time and resource to put in the safeguards so that it can’t be used improperly. That’s one of the things that I think a lot of people are coming to terms with.”
Related Items:
AI Ethics Still In Its Infancy
AI Ethics and Data Governance: A Virtuous Cycle
Giving DevOps Teeth To Crunch Down on AI Ethics Governance