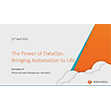
A ‘Breakout Year’ for ModelOps, Forrester Says
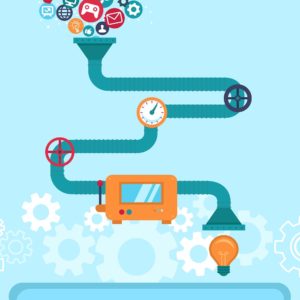
(venimo/Shutterstock)
The rapid maturation of machine learning operations (ModelOps) tools is leading to a “breakout year” for ModelOps, Forrester says in a recent report.
The ML lifecycle is a potential nightmare for many organizations, write Forrester analysts Mike Gualtieri and Kjell Carlsson in an August report, titled “Introducing ModelOps to Operationalize AI.”
“This process takes too long and is fraught with technical and business challenges, just with one model,” the analysts write. “What about a dozen use cases and models? A hundred? A thousand?”
The answer, of course, is ModelOps (also known as MLOps), which Forrester defines as “tools, technology, and practices that enable cross-functional AI teams to efficiently deploy, monitor, retrain, and govern AI models in production systems.”
Gualtieri and Carlsson identify three core ModelOps capabilities that organizations must have if they’re going to succeed with AI at scale.
For starters, they need to deploy and serve ML models. Data scientists and machine learning engineers have a wide range of tools available to them for building ML models, including open source products like TensorFlow and PyTorch. But developing the model is just the start, and they need to be moved into production to get any use out of them.
ModelOps tools can help alleviate the burden of manually deploying models into production, including in public clouds, private clouds, and on-prem environments, while minimizing the scalability, latency, and security tradeoffs of each environment, the analysts say.
Secondly, ModelOps provides monitoring capabilities to ensure ML models don’t go off the rails. On their own, models rarely get better with age. To keep models making accurate predictions, they need to be retrained. Only by closely monitoring the performance of the models can an organization know when it’s time (or past time) to retrain the model.
The Forrester analysts identify several types of drift that organizations need to be on lookout for, including: data drift; prediction distribution drift; concept or business KPI drift; and explainability and fairness. There are a lot of moving parts in AI, so monitoring is key to ensuring that everything is working as planned.
Finally, the ML lifecycle must be managed. Forrester recommends users track metadata, lineage, and other dependencies that go into a model, and use modern DevOps techniques to orchestrate the workflow amongst a distributed team.
Monitoring the lifecycle of ML model development is particularly important in light of emerging data privacy regulations and the increased attention that AI is receiving in s regards to biases and the potential for it to be used for discrimination.
Forrester highlighted Algorithmia, ModelOp, Modzy, Quickpath, and superwise.ai as vendors offering ModelOps solutions. It also referenced work done by IBM Watson, Cloudera, and Domino Data Lab in its report, which is available for download from the ModelOp website. All of the public cloud providers offer their own ModelOps (or MLOps) products, as well.
Forrester encourages organizations to look to the established vendors in the space before building their own ModelOps solution. DevOps isn’t the same as ModelOps, but there are similarities between the two and some lessons learned in DevOps can be applied in ModelOps. ModelOps works best when there is a clear chain of command and established processes are followed.
Related Items:
Google Joins the MLOps Crusade
Data Science and ML Platform Market Heats Up
Growing Focus on MLOps as AI Projects Stall