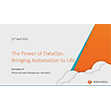
Understanding the Limits of AI
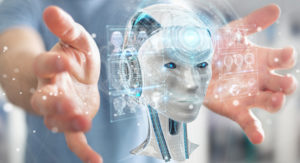
(sdecoret/Shutterstock)
There’s no denying that artificial intelligence is having a huge impact on our lives. According to PwC, AI will add $16 trillion to the world’s economy over the next 10 years as automated decision-making spreads widely. Despite this incredible impact, AI doesn’t bring much value for some problems, like predicting a viral pandemic, forecasting the winner of the presidential election, or servicing clients with diverse needs, experts say.
Data is, of course, the rootstock for all forms of AI, whether it takes the form of a basic search engine or a self-driving car. But it turns out that some data are quite hard to come by, even for some of the most high-impact events. And when there’s no data, there can be no AI.
Take COVID-19, for example. While public health officials have been warning about the likelihood of a viral pandemic for years, there was no way to predict the exact timing of an outbreak like we’re currently having, says Mike Gualtieri, a vice president and principal analyst with Forrester.
“The idea that you could have some sort of model that would just spit out the answer that would say, yes today or next month we’re going to have a global pandemic is probably not possible with AI,” Gualtieri says. “And the reason for that is AI models are generally machine learning models that are trained on historical data and patterns. And if you don’t have enough of those patterns of cases where something happens and where something doesn’t happen, then you’re probably not going to be able to predict that.”
The novel coronavirus that’s currently ravaging the globe is thought to have originated in a live animal market in the Chinese city of Wuhan sometime last November. Experts say the new virus likely crossed over from a bat into one or more people that visited that “wet market.” Trying to predict the likelihood of that one crossover event happening within any reasonable timeframe is likely beyond the power of statistics as we know it. Like global weather predictions more than seven days out, there is simply too much randomness, or entropy, to make anything more than an educated guess.
In lieu of solid data, we can look to proxies make educated guesses for things like global pandemics. “For a virus, especially a pandemic like this, the only hope really is to understand what those proxies are and them try to predict those to get some idea or probability of an event like that happening,” Gualtieri says.
With a proxy approach, instead of directly detecting the presence of a virus, data scientists would be looking for indirect impacts. They have to be very creative in hypothesizing what other proxies could be used, how to measure them, and whether they’re correlated.
But even then, how do you know the models right? They could try to back test it, Gualtieri says, but even that doesn’t bring any guarantee of finding predictability. “We’re just fitting variables to a circumstance that already occurred,” he said. “We don’t know if that will work in the future.”
Black swan events like COVID-19 are notoriously tough to predict. But even when you know the exact timing of an event, AI can’t always help us predict the outcome. That’s the case with US presidential elections, which occur, like clockwork, every four years – and often bring surprises every four years.
“People would love to be able to predict that, but people can’t predict presidential elections very well at all,” Gualtieri says. “That’s because there are so many variables and the variables that matted in one presidential election are different variables that matter in the next one.”
Limits of AI
AI is not a one-size-fits-all solutions, according to Chris Meyer, a professor at Rensselaer Polytechnic Institute. Each company needs to decide for itself whether to adopt AI and in what way, Meyer writes in his recent paper, “AI and Machine Learning in Service Management.”
“AI has the potential to upend our ideas about what tasks are uniquely suited to humans,” Meyer told Rensselaer News last week, “but poorly implemented or strategically inappropriate service automation can alienate customers, and that will hurt businesses in the long term.”
Before investing in AI, Meyer recommends that business leaders carefully examine their strategies for managing knowledge resources. Replacing human-made decisions with decisions made by algorithms can work in some instances, particularly where businesses limit choice and interaction with employees. But algorithmic-powered decision-making has the potential to backfire when a company relies on the human touch or offers a range of services that change from client to client, he says.
“The ideas are of use to managers, as they suggest where and how to use automation or human service workers based on ideas that are both sound and practical,” Meyer tells Rensselaer News. “Managers need guidance. Like any form of knowledge, AI and all forms of service automation have their place, but managers need good models to know where that place is.”
Much to Learn
The range of possible uses for AI are as varied as the extent of human knowledge. That’s one of the great characteristics about AI – it can be used to predict the outcome of just about any phenomena that can be sufficiently quantified or qualified. The possible uses of AI are staggering when applied to the macro and the micro worlds.
But AI has its limits, and ironically, one of AI’s biggest blind spots is people. Take, for example, the Fragile Families Challenge, a machine learning project to predict and measure life outcomes for children, parents, and households across the United States.
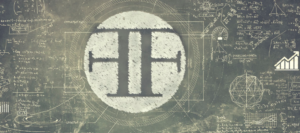
The Fragile Families Challenge is a mass collaboration that combines predictive modeling, causal inference, and in-depth interviews to yield insights that can improve the lives of disadvantaged children in the United States.
Even when armed with high-quality dataset containing 13,000 data points for more than 4,000 families, the best AI models were not very accurate. Brian J Goode, a Virginia Tech research scientist and one of 112 co-authors of the resulting paper published last month in the Proceedings of the National Academy of Sciences, says there’s much to learn.
“It’s one effort to try to capture the complexities and intricacies that compose the fabric of a human life in data and models,” Goode says. “But, it is compulsory to take the next step and contextualize models in terms of how they are going to be applied in order to better reason about expected uncertainties and limitations of a prediction. That’s a very difficult problem to grapple with, and I think the Fragile Families Challenge shows that we need more research support in this area, particularly as machine learning has a greater impact on our everyday lives.”
Organizations are investing billions of dollars to build AI-powered applications and systems that will enable them to compete in an increasingly data-driven world. But it’s becoming increasingly clear that knowing when to apply AI, and when not to, is perhaps even more important than knowing how to build an algorithm.
Related Items:
A Race Against Time to Model COVID-19
5 Things AI Is Better At Than You