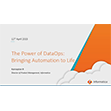
Sorting AI Hype from Reality
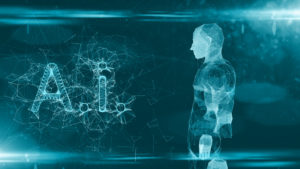
(Immersion Imagery/Shutterstock)
Several years ago, some wondered whether we had hit “peak Hadoop.” In retrospect, we had. Now we’re experiencing a similar phenomenon with the hype surrounding AI, and once again, some are wondering if it’s sustainable, and if not, when the hype will fade.
There are two sides to every coin, and so it is with artificial intelligence, which analysts predict will drive trillions of dollars in spending and value creation during the coming decades.
On the one hand, we’ve made incredible advances in machine learning, thanks largely to the advent of deep neural networks that are trained on huge amounts of data using very fast GPUs. This form of AI has propelled the state of the art in computer vision and speech recognition to the point where computers have exceeded the powers of human sensory perception in some areas.
But on the other hand, we still have a long ways to go before we start to see the types of automation that many expect from AI. While it’s true that companies are pushing the boundaries of what’s possible with self-learning machines and programs, we’re also running into the limitations of AI technology as it exists today.
Self-driving vehicles are a perfect example of this AI conundrum. Carmakers and other companies are investing billions of dollars in building GPU-powered deep learning systems that will allow a car to take data from various sensors and digital maps, and use it to safely navigate city streets. There has been a lot of progress made, but there are still serious obstacles that will prevent us from seeing self-driving cars become widespread anytime soon.
The limits of current AI technology for self-driving cars became evident in a recent story shared by DarwinAI, a Toronto, Canada AI company. One of the company’s clients is an autonomous car company, which discovered that its cars would suddenly turn left for no apparent reason. “It made absolutely no sense,” DarwinAI CEO Sheldon Fernandez said.
After extensive debugging, the company realized the neural network at the heart of its navigation system had made a spurious correlation between the color of the sky and the need to turn a given direction. The cars were trained in Nevada, where the dry desert air has been known to play tricks on human eyes, let alone digital ones.
This exposes one of the limitations of AI today, which is that the applications are very narrow. Machine learning models that are trained in one environment (the training data) will often not perform well when exposed to another environment (the real world). Hortonworks Data Science Evangelist Robert Hryniewicz hit on this point during his sessions “Overcoming AI Hype” at the recent Dataworks Summit.
“The limit of today’s AI … is being able to take it from one environment and put it in another and [have it] behave competently,” Hryniewicz says. “Taking what you’ve trained in one set and putting it elsewhere usually doesn’t work and it breaks miserably.”
That doesn’t take away from the huge leaps forward AI researchers have made when AI is confined to strict boundaries. When it comes to board games like chess and video games, neural network-powered AI programs display capabilities that far exceed the top human players. But when it’s let loose on real-world data, today’s AI programs often go awry.
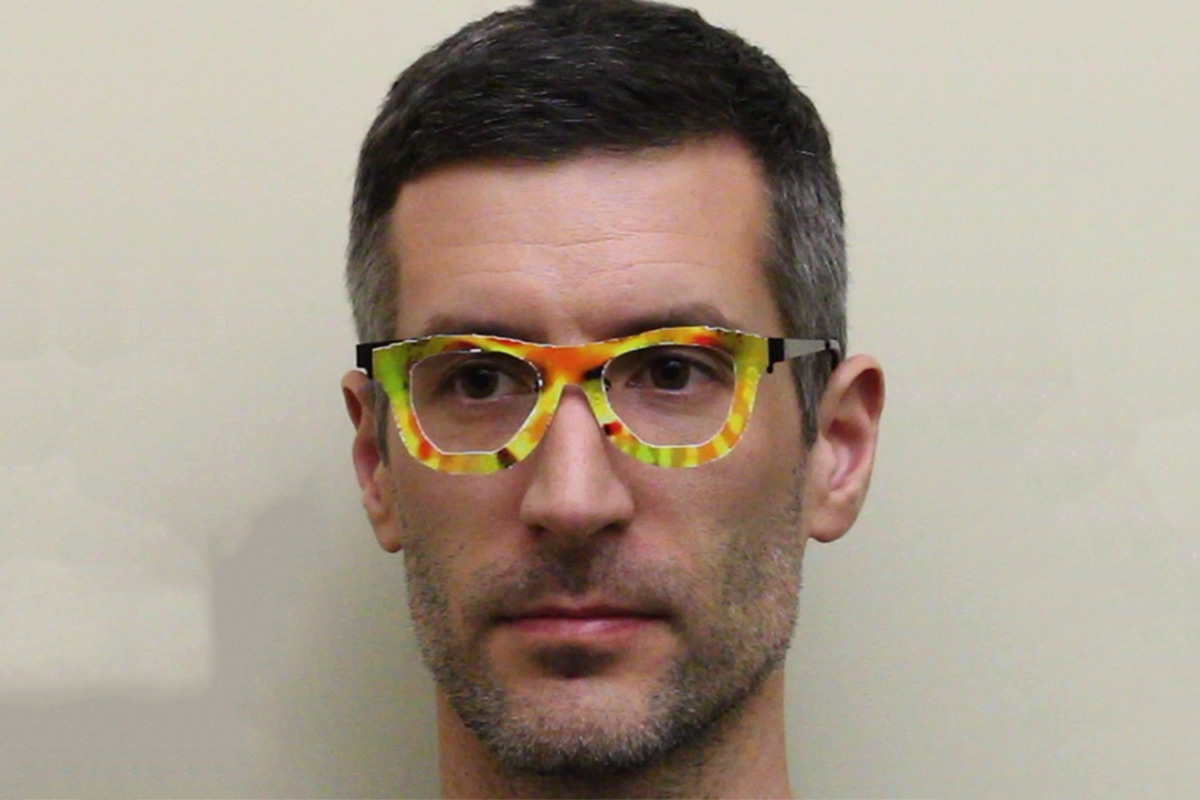
Carnegie Mellon computer science PhD student Mahmood Sharif, co-creator of the glasses that make AI think you’re Milla Jovovich
“This is part of a broader pattern of AI systems achieving superhuman levels of performance and yet making blunders that leave us scratching our heads,” writes Vincent Conitzer, a computer science professor at Duke University, in a story in today’s Wall Street Journal. “Researchers from Carnegie Mellon were able to fool a facial recognition system consistently into thinking that one of them, clearly a man, was actress Milla Jovovich, by wearing carefully designed eyeglass frames.”
It’s actually fairly easy to trick AI. In his Dataworks session, Hryniewicz shared the example of a computer vision algorithm being fooled into thinking a banana was a toaster simply by posting a small colorful sticker that contained the image of a toaster next to the delicious yellow fruit. If self-driving cars are ever allowed on the road, hackers could wreak havoc by tricking a car’s AI system into thinking a stop sign is a yield sign simply by using one of these stickers, he says.
But the problems don’t end there. Just as deep neural networks are excelling at recognizing sights and sounds, they’re also getting quite good at generating sights and sounds of their own. It’s great fun to see what kind of art AI can generate. But there’s nothing stopping hackers from using this capability to spoof the faces and voices of prominent individuals, and posting the content online.
“Very soon the problem we’ll have is we won’t be able to recognize what is real and what is not,” Hryniewicz says. To get in front of this problem, tech firms are hiring the AI experts who can build these types of systems to help build systems to detect the spoofs, he says.
There’s no debate that narrowly applied AI will play a big role in automated systems in the years to come. Even if AI gets the right answer only 90% of the time, the benefits of being able to instantly react to incoming data streams in a (mostly) correct manner will be extremely valuable. It’s also quite feasible that AI researchers will continue to improve on AI’s accuracy, and come up with novel ways to keep AI from going off the rails.
However, there’s no indication that we’re on the cusp of overcoming the core limitations to today’s AI techniques, which can be summed up as “statistics on steroids.” Short of a breakthrough in understanding how the human mind actually works — what has been dubbed “cracking the brain code” — it seems unlikely that the long held dream of creating a “general AI,” or a more human-like AI that works like we do, will come to pass anytime soon.
Since AI entered mainstream thought 70 years ago, there have been periods of promising innovation followed by periods where innovation seemed to stall. AI researchers like to call these AI summers and AI winters. We’re currently in the midst of a scorching AI summer. When will the heat of today’s AI breakthroughs give way to reality?
“Right now we’re at the peak of the hype cycle in AI,” Hryniewicz says, “so take that into consideration when you talk to developers, and take it with a grain of salt.”
Related Items:
Gartner Sees AI Democratized in Latest ‘Hype Cycle’
How AI Will Spoof You and Steal Your Identify
Bright Skies, Black Boxes, and AI