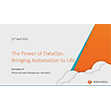
Before Strategizing, Conduct an AI Audit
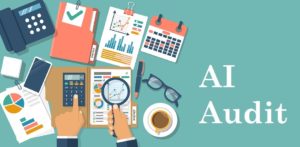
(hvostik/Shutterstock)
Studies forecast that AI will boost profitability by an average of 38% by the year 2035. If an AI program interpreted this data, the result would be conclusive: Artificial intelligence is quickly becoming one of the economy’s sharpest competitive edges.
According to McKinsey & Company, just 47% of executives report embedding AI into one business process, and only 21% report implementing AI in multiple ways. While the technology is progressing at historically unprecedented rates, the majority of enterprises still face either barriers to entry or difficulty determining next steps.
Whether a company is beginning its journey with AI, or ready to take the next measured step, it’s essential to conduct a comprehensive AI audit. This review process can help determine where AI will have the greatest impact, how much a team already knows, and which roles, if any, must be added to create an agile infrastructure.
Conducting an internal AI audit is an actionable step for business leaders before hiring third-party vendors or outside experts. Follow these steps to create a comprehensive AI audit successfully.
1. Review Current AI Systems
While implementing AI is currently on-trend, it’s not necessarily new. With mounting discoveries spanning decades, AI commonly pervades everyday tasks in payroll, scheduling, accounting, marketing, distribution, and beyond.
The first step in auditing the AI landscape is to highlight each way AI currently impacts the business. It could be add-ons to an e-commerce website, or licensed programs used in siloed departments.
2. Establish a Training Baseline
No department is immune to the potential of AI. If a company strives toward a future involving AI, it’s
imperative to educate staff about the basics and possibilities of the technology. For small- and mid-sized companies, there may not be data scientists or data engineers on staff; however, most companies employ IT staff, if not an entire department. Determine who knows what—platform expertise, data management, languages, etc.—and depending on the AI-literacy of the organization, set the groundwork for training.
Companies like Lynda and Udacity offer affordable online courses to either introduce or immerse learners into topics of interest, including AI. Infused with knowledge, each employee becomes a torchbearer for the cause. Being privy to the possibilities, team members become more likely to spot opportunities as they arise.
3. Note Opportunities
At any point in the AI journey, leaders should seek ways to build momentum.
For companies beginning with AI: start small. Large scale AI projects require significant investment, time, and human resources; experts encourage businesses to take baby steps. By limiting the scope of a pilot project, leaders give themselves a chance to learn the ins-and-outs of building AI.
While auditing, look for repetitive tasks requiring a small amount of judgment and document them. Consider ways to augment employees’ workflows, not replace roles entirely. These all make good starting points. Catalog potential ideas from stakeholders, leaders, and employees invested in the outcome.
4. Map the Talent Gap
There are two types of AI products: off-the-shelf applications and custom solutions. Many businesses utilize off-the-shelf products to enhance their business, whether it’s a predictive SEO website plug-in, or a chatbot living on the back end of the website. These products can mainly be managed by whoever oversees their domain, no specialty-skills necessary.
On the other hand, custom AI solutions involve several moving parts requiring different areas of expertise. Depending on the scope of the project, it could require data scientists, big data engineers, software developers, and the list goes on.
The good news is that many AI specialists learn crossover skills, languages, and programs. Document what the team already knows and identify which skills are missing. Take note if existing team members are eager to grow their knowledge in specific areas.
Without being aware of the talent already on-board, it’s impossible to know which gaps need filling.
5. Establish the Ground Rules for Governing AI
Experts warn against the misuse of AI. Whenever private or proprietary data is involved, companies face increased risk of hacking and breaches. When completing an AI audit, inspect the security measures in place and consider the steps necessary to prevent malicious use of the technology. Part of establishing the system’s security is recognizing which roles take responsibility if a problem occurs.
Cybercrime remains a significant source of worry for contemporary consumers, and companies are liable for their data. By fleshing out ground rules and security measures for AI systems, enterprises take a precautionary step to protect their interests and the well-being of their customers.
Determining Next Steps
Conducting an AI audit provides business leaders with a map to navigate their future. Once the audit is complete, stakeholders can consult the document to make informed decisions regarding next steps. As AI theorist Eliezer Yudkowsky said, “By far, the greatest danger of Artificial Intelligence is that people conclude too early that they understand it.”
About the author: Alex Fly is the CEO of Quickpath, a data science platform that bridges the gap between data science analytics environment and the IT operational environment. Alex has contributed to TechTarget, DZone, and TechRadar.
Related Items:
What’s the Difference Between AI, ML, Deep Learning, and Active Learning?
The Hard Questions of Hiring For Machine Learning