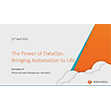
AutoML Tools Emerge as Data Science Difference Makers
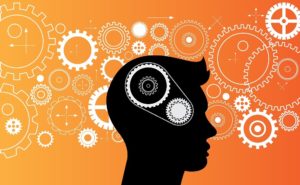
(admin_design/Shutterstock)
The days of handcrafted algorithms aren’t quite over, but it’s hard to dismiss to impact that automated machine learning (AutoML) is having on the data science field. As companies look to imbue intelligence into their products and services, AutoML tools will lower the barrier of entry into data science and open the door for data-driven automation on vast scales.
In the past few years, we’ve seen a surge of interest in AutoML tools, which automate a range of tasks in the data science workflow. While automated ML features may be found in a range of tools, the AutoML category has a fairly defined set of features, including: acquiring and prepping data; engineering features from the data; selecting the best algorithm; tuning the algorithm; and deployment and monitoring of production models.
Forrester says just about every company will have a stand-alone AutoML tool. “We expect this market to grow substantially as products get better and awareness increases of how these tools fit in the broader data science, ML, and AI landscape,” Forrester analysts Mike Gualtieri and Kjell Carlsson write in a May Forrester New Wave report on the AutoML market.
Gartner, meanwhile, says that by 2020, more than 40% of data science tasks will be automated. That will boost the productivity of citizen data scientists, which as a group is growing 5x faster than professional data scientists, Gartner says.
In the May report, Forrester analysts ranked DataRobot, H2O.ai, and dotData as the three leading providers of AutoML solutions out of a field of about 10. DataRobot received high marks across the board and has the early lead in the field, but H2O.ai is right there with its Driverless AI solution, which Forrester days is mainly geared toward empowering existing data scientists.
In terms of the number of customer deployments, H2O.ai and DataRobot are far and away the biggest vendors. They’ve also been around longer, which has allowed venture capitalists to invest nearly $225 million in DataRobot and $147 million in H2O.ai, about half of which was announced last week.
dotData, which was spun out of NEC in 2018, was characterized by Forrester as a bit of a dark horse candidate. It has a solid set of capabilities – particularly around feature engineering — but not a lot of market recognition as of yet.
“Currently the AutoML market is very hot and growing very rapidly,” says Ryohei Fujimaki, the founder and CEO of dotData. “We are still early in market development compared to the other two….We started in the Japanese market in 2016. In the US market, our market awareness is increasing.”
Fujimaki says a majority of dotData customers are citizen data scientists who use dotData’s GUI tool to lead them through the process of building machine learning models. More advanced users are employing a second product Python-interface that gives them more control.
DataRobot is targeting the citizen data scientist, says DataRobot’s Chandler McCann. “Somebody who’s familiar with Excel, somebody who has some kind of an affinity towards the data, but not necessarily a data scientist,” he says. “The key requirement to using DataRobot is really domain knowledge. We take care of the computer science and the stats part.”
McCann says organizations are turning to AutoML tools because of the difficulty of machine learning. “You hear about failed projects or not getting the return on investment that they wanted,” he tells Datanami. “The takeaway is that machine learning projects can be hard. That’s why at DataRobot we built an entire success organization to help organizations clarify what they want to do.”
Other AutoML vendors that made Forrester’s cut include Aible, Bell Integrator, Big Squid, DMway Analytics, EdgeVerve, and Squark.
Aible was listed as Strong Performers in the Forrester Wave thanks to above-average capabilites in user experience, model evaluation and training, and vision. EdgeVerse was also listed as a Strong Performer thanks to above average capabilites in data, model training, and roadmap.
Another new entry into the AutoML business is Databricks. The Apache Spark backer launched its AutoML solution last week with a set of automated features around feature engineering, model search, hyperparameter tuning, and deployment.
Databricks is hoping to empower three groups — data scientists, data engineers, and citizen data scientists – to help them build machine learning applications. “The common theme we hear from our customers is how can we increase the productivity of all these people?” says Clemens Mewald, Databricks director of product management for machine learning and data science. “As part of that we are making things easier by bringing in automation.”
There are many AutoML solutions available on the cloud as well, with Amazon Web Services Sagemaker getting the most attention. With the growth of the cloud, Google Cloud’s AutoML and Microsoft Azure’s Machine Learning Service are also expected to see plenty of use in the months to come.
Related Items:
Databricks Offers Something for Everybody with AutoML Solution
H2O.ai Tops Off Funding to Accelerate AI Adoption
Cloud Tools Rev Up AI Dev Platforms