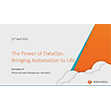
Empowering Citizen Data Science
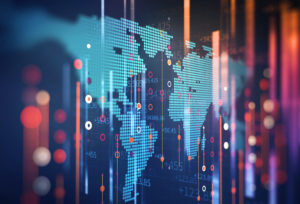
(whiteMocca/Shutterstock)
Companies of all stripes are turning to data science to unlock the value in their data. However, finding highly trained data scientists to build the systems has proven to be a very difficult task. Now many organizations are looking to citizen data scientists to help them find actionable insights within big data.
While the idea of citizen data science isn’t new, the practice is still fairly new to many companies. The entire discipline of data science is still in its early stages, which means the citizen data science movement is even earlier in its evolution. Despite the novelty of citizen data science, the practice is growing quickly thanks to a number of factors, including difficulty in finding full-fledged data scientists and steady improvement in the quality of tools.
Gartner has been a leader in promoting citizen data science concepts for the past several years. In 2014, the analyst group predicted that the number of citizen data scientists would grow five times faster than data scientists through 2017. While it’s unclear if the numbers panned out, it would not be surprising if the citizen data science cohort exceeded that prediction.
Citizen data scientists do many of the same tasks as their higher trained colleagues, according to Gartner analyst Carlie Idoine. In her recent blog post “Citizen Data Scientists and Why They Matter,” she writes that a citizen data scientist is somebody who “creates or generates models that use advanced diagnostic analytics or predictive and prescriptive capabilities, but whose primary job function is outside the field of statistics and analytics.”
Citizen data scientists, Idoine continues, are “‘power users’ who can perform both simple and moderately sophisticated analytical tasks that would previously have required more expertise,” she says. “They do not replace the experts, as they do not have the specific, advanced data science expertise to do so. But they certainly bring their OWN expertise and unique skills to the process.”
There are a variety of job titles that citizen data scientists might have in the real world, and many of them are some variation on the business analyst role. Depending on the industry, a company may have several experienced analysts in finance, marketing, or logistics that they can turn to for answers on tough questions.
Many of these business analysts are fluent in SQL, which has been data’s lingua franca for decades. Knowing how to write a SQL query to get answers out of relational databases remains a critical skill in today’s marketplace. After all, there’s a lot of business data storedi n relational databases.
But increasingly, the analysts are being asked to get answers from data that’s not sitting in a relational database and therefore can’t be easily queried through SQL. These analysts – these citizen data scientists – are being asked to work with machine learning models that can generate predictions from a range of data types being collected by the company. SQL is definitely helpful here, but knowing how to leverage other tools and technologies, such as Python statistical libraries or Jupyter notebooks, can take them farther.
DataRobot develops a proprietary data science and machine learning automation platform that’s also finding plenty of usage among the non-data-scientist set. According to Jen Underwood, a business intelligence industry veteran who was recently hired as DataRobot’s director of product marketing, says there’s a spectrum of users.
“Almost half of the customers successful with DataRobot today are in this business analytics segment,” she told Datanami recently. “It’s fascinating. These were things that certainly were not done in the past.”
DataRobot helps citizen data scientists swing above their weight through the power of automation. The company’s platform essentially puts up “guard rails” that guide analysts, power users, and even application developers through the machine learning process – including algorithms selection, feature engineering, model training, and deployment – and hopefully prevents them from making mistakes.
“There’s a lot happening behind the scenes that folks don’t realize necessarily is happening,” Underwood says. “When I was doing data science, I would run one algorithm at a time. ‘Ok let’s wait until it ends, see how it does, and try another, one at a time.’ [With DataRobot] a lot of the steps I was taking are now automated, in addition to running the algorithms concurrently and ranking them.”
While DataRobot enables citizen data science by automating model management and accelerating training, it often leaves other aspects of data science – specifically the time-intensive data preparation and transformation tasks – to others. That’s where its partner Trifacta comes in.
Trifacta’s Data Wrangler software uses a variety of techniques (including machine learning) to automate the data cleansing tasks. It’s been well-documented that data scientists spend upwards of 80% of their time manually prepping data to make sure the machine learning algorithms give the most accurate answers. What better way to turbo-charge data science progress in a company by putting the data-prep phase in the hands of citizen data scientists?
Trifacta recently conducted a persona study for users of its software and discovered there was a huge variety of people with a large number of titles using its software. “Their job title doesn’t have that much correlation to what they do on a daily basis,” says Wei Zheng, Trifacta’s vice president of products.
That is basically what you would expect to find when data science moves from the ivory tower to the self-service world. As big data technology progresses, data science capabilities that were previously restricted to the domain of a few highly skilled individuals are now accessed through a much wider pool of interested parties. It’s the democratization of data science, and the beneficiaries are data science citizens and the companies they serve.
“You don’t need to be an advanced or an official data scientist to be able to, for example, understand churn or how do you want to model your customer retention, or all these use cases that previously would take much higher technical skills to do end-to-end,” Zheng says. “It’s crazy to me how much we’ve advanced.”
Related Items:
How To Build a Data Science Team Now
What Kind of Data Scientist Are You?
Taking the Data Scientist Out of Data Science