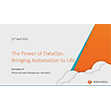
DARPA Expands ‘Lifelong’ Machine Learning Effort
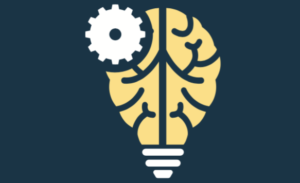
As AI and machine learning systems advance, real-world applications are exposing limitations such as the inability to adapt to situations beyond the narrow tasks those systems were trained to perform.
Indeed, some observers note that AI researchers still do not understand the mechanisms by which automated systems learn.
As AI researchers grapple with these unknowns, the Defense Advanced Research Projects Agency (DARPA) launched a program last year aimed at advancing “lifelong machine learning.” This week, the research agency announced funding for three university research teams that will tackle various components of the adaptability problem.
Those efforts augment initial research at Columbia University focused on reproducing neural networks.
“We are not looking for incremental improvements in state-of-the-art AI and neural networks, but rather paradigm-changing approaches to machine learning that will enable systems to continuously improve based on experience,” said Dr. Hava Siegelmann, program manager for DARPA’s Lifelong Learning Machines effort.
A team led by the University of California at Irvine will study the “dual-memory architecture” of the human hippocampus, thought to be the center of memory and the autonomic nervous system, along with the cerebral cortex. DARPA said the team will attempt to create a machine learning system “capable of predicting potential outcomes by comparing inputs to existing memories, which should allow the system to become more adaptable while retaining previous learnings.”
A second team lead by Tufts University researchers will look to the animal world for analogs that could be applied to adaptive learning. Among the approaches will be examining regenerative mechanisms observed in amphibians like salamanders to create “flexible robots” capable of altering the structure and functions in response to changes in their environment.
University of Wyoming investigators will develop a computational system that attempts to use context to identify appropriate “modular memories” that the system would attempt to “reassemble” based on new sensor data. The goal is to “rapidly form behaviors to suit novel circumstances,” DARPA said. The research also would draw on adaptation techniques associated with memory reconsolidation, a biological process in which memories are formed, stored and used for tasks like problem solving.
Early stage research funded under the DARPA machine learning program has yielded advances in building and training a “self-replicating” neural network, the agency reported in Thursday (May 3). As neural networks learn, the research is predicated on the assumption that it adapts to new situations. The goal is to develop a system that can adapt “by using knowledge of its own structure,” DARPA said.
The results of the DARPA-funded research at Columbia University’s engineering school are available here.
“The research team’s work with self-replicating neural networks is just one of many possible approaches that will lead to breakthroughs in lifelong learning,” added Siegelmann, the DARPA program manager.
The machine learning initiative is the latest AI and big data effort launched by the DoD top research agency as it again looks to jumpstart another high-risk, high-payoff technology effort.
Recent items:
US Pursues ‘Abductive Reasoning’ to Divine Intent
Why Cracking the ‘Brain Code’ Is Our Best Chance For True AI