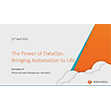
U.S. Pursues ‘Abductive’ Reasoning to Divine Intent
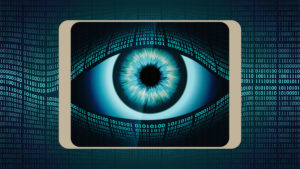
(Valery Brozhinsky/Shutterstock)
The 9/11 Commission identified a lack of coordination among U.S. intelligence agencies as a reason for failing to detect attacks on U.S. soil. One of the responses to the panel’s findings was a multiagency effort to upgrade analytics efforts with particular emphasis on an emerging approach known as abduction that combines higher-order reasoning and machine intelligence to gauge an adversary’s intentions.
Responding to directives to adopt a more formal approach to uncertainty associated with intelligence analysis, including scrutiny of “alternative hypotheses,” Pentagon and intelligence research agencies have been pouring funding into abduction technology. Those efforts focus on developing frameworks that can automatically gather evidence—known in military parlance as “indications and warnings”—that would help intelligence analysts form competing hypotheses about enemy intent.
“Abduction assumes there is insufficient evidence to deduce any single explanation or cause,” said Rick Pavlik, director of analytics and machine intelligence at Polaris Alpha, an early developer of the abduction, or “anticipatory analysis” technology. Inferring intent is “really the emphasis of what we are trying to do.”
The approach seeks to move beyond traditional deductive and inductive reasoning and machine intelligence approaches that often require large data sets to train models. The problem for spy applications like detecting terror attacks is a lack of data needed to train models.
“People would say, “Well, why can’t you figure out terrorism?” Eric Schmidt, former Google executive chairman, told a recent AI security summit.
“Well, the good news is terrorism is very rare. So it’s much, much harder to apply AI to that problem” whereas “something that occurs every day, is far, far easier because you have so much training data,” Schmidt added.
Hence, intelligence agencies are looking for new frameworks that rely less on training data and more on new tools like abduction that seek to provide plausible scenarios about an adversary’s intentions that could be used to thwart future attacks.
Pavlik distinguishes abduction from deductive and inductive reasoning, noting that the approach reverses the standard cause-and-effect approach. In contrast, abduction reasons from effect to the plausible causes “with the goal of identifying that most likely cause,” he explained. If, for example, spy satellites detected unusual troop movements, abduction could be used to provide “the best explanation for an adversaries’ intentions,” Pavlik added in an interview.
While Apache Spark and a growing list of development tools has helped commoditized inductive reasoning approaches to machine learning, Pavlik notes there currently are no comparable frameworks or tool sets for abduction. That is one of the focus areas of R&D efforts funded by the Defense Advanced Research Projects Agency and its intelligence agency counterpart, IARPA. Those efforts seek to go beyond relatively straightforward automated reasoning systems to provide intelligence analysts with new tools that can be used to gather intelligence, develop hypotheses and assist policy makers in determining an adversary’s true intent.
A DARPA program called Active Interpretation of Disparate Alternatives draws on a variety of structured and unstructured data sources to apply abduction. The goal is to “develop a multi-hypothesis semantic engine that generates explicit alternative interpretations of events, situations, and trends from a variety of unstructured sources, for use in noisy, conflicting, and potentially deceptive information environments,” a program summary notes.
Analytics startups like Polaris Alpha, Colorado Springs, Colo., argue that scalable abductive reasoning frameworks don’t yet exist. “We believe that this is about to change in a big way,” Pavlik said.
Formed in 2016, the startup is working with DARPA and U.S. intelligence agencies to develop what Pavlik dubbed “general purpose hypothesis orchestration systems” used to automate scalable abductive reasoning.
Recent items:
Machine Learning Tracks U.S. Spy Planes
Gaining Control Over AI, Machine Learning