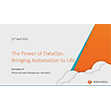
Twitter Ranking Tweets With Machine Learning
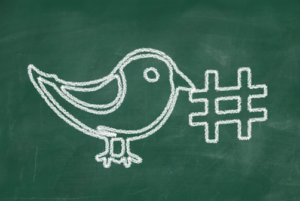
via Shutterstock
Machine learning is entering production at Twitter as a way of ranking tweets and boosting engagement.
Twitter engineers this week unveiled the social media platform’s ranking algorithm driven by deep neural networks. In a blog post, company engineers said their approach leverages an in-house artificial intelligence platform that includes new modeling capabilities.
Among the results, wrote Nicolas Koumchatzky, a software engineer with Twitter’s AI team called Cortex, are “more relevant timelines now, and in the future, as this opens the door for us to use more of the many novelties that the deep learning community has to offer, especially in the areas of [natural language processing], conversation understanding and media domains.”
Currently, Twitter (NYSE: TWTR) timelines are arranged chronologically based on a user’s last visit. That alone is a daunting infrastructure task, the company notes. The new ranking algorithm gathers all tweets from accounts being followed by an individual user. It then scores those tweets using a relevance model, with the goal of predicting the most relevant comments. The highest-ranked tweets are then display at the top of a user’s timeline, Koumchatzky explained.
Twitter’s new ranking model takes into account factors such as the number of re-tweets, likes, the inclusion of images and video and other responses to a post. It also attempts to gauge a user’s past interactions with authors along with the “strength” and origin of the follower’s relationship to an author.
“This scoring step imposes an even greater computational demand on timelines serving infrastructure, as we are now scoring thousands of tweets per second to satisfy all of the timeline requests,” the Twitter engineer noted. “The unique challenge is to perform scoring quickly enough to instantly serve tweets back to the people viewing their timelines, yet have powerful enough models to allow for the best possible quality and future improvements.”
Along with prediction models, Twitter said similar requirements are applied to its machine learning frameworks. A set of tools is used to train and launch a prediction model, with particular attention given to training speed and scaling when dealing with very large datasets, much of it unstructured.
Based on the breadth of research into new AI algorithms and model architectures, Koumchatzky argued, “betting on a platform that natively supports deep learning and complex graphs is key to leveraging the promises of that work.”
The Cortex team of data scientists and machine-learning researchers works on Twitter’s deep learning platform. New members were added last year when Twitter acquired London-based AI startup Magic Pony Technology. These and other deals are part of a push by social media and other hyper-scalers to train rather than just program algorithms. It also underscores how AI technology is finding new automation applications required to organize huge datasets.
Having worked out most of the kinks in its deep learning models and platform, Twitter’s Koumchatzky said “online experiments have also shown significant increases in metrics such as tweet engagement and time spent on the platform.”
Recent items:
Twitter Buys Another Machine Learning Startup
Unleashing Artificial Intelligence With Human-Assisted Machine Learning