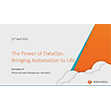
Data Gravity Pulls to the Cloud
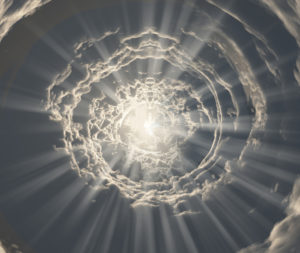
(Bruce Rolff/Shutterstock)
Last month, Spotify grabbed headlines by announcing plans to get rid of its data centers and move onto Google’s Cloud Platform (GCP), claiming that the storage, compute and network services in the cloud are as high quality as on-premise alternatives. While few people take a second look at a digital-native company choosing to store data in the cloud, it seems to be generally accepted that for certain companies and industries, the cloud just isn’t a fit.
Hadoop distribution vendors like Cloudera and Hortonworks (NASDAQ: HDP) have traditionally posited that big data is best done in company-owned data centers, alleging superior security, cost and performance. And while that may have been true in the past, the cloud has never been a better option for big data.
Data Gravity
One of the major reasons put forth by the distribution vendors for not moving big data to the cloud that is the theory of “data gravity,” in which that all of the data that needs to be correlated for analysis must move to the location of the largest data set, which historically has been the data center.
While this concept is correct, the data created in the cloud is far outpacing data created on-premise, causing a gravitational shift. Companies are building applications and processing massive datasets on the cloud at a scale that is very difficult to reach on premise for most enterprises. These use cases were on display at last year’s AWS re:Invent, where companies across industries revealed how they are creating applications that are generating massive data sets on the cloud.
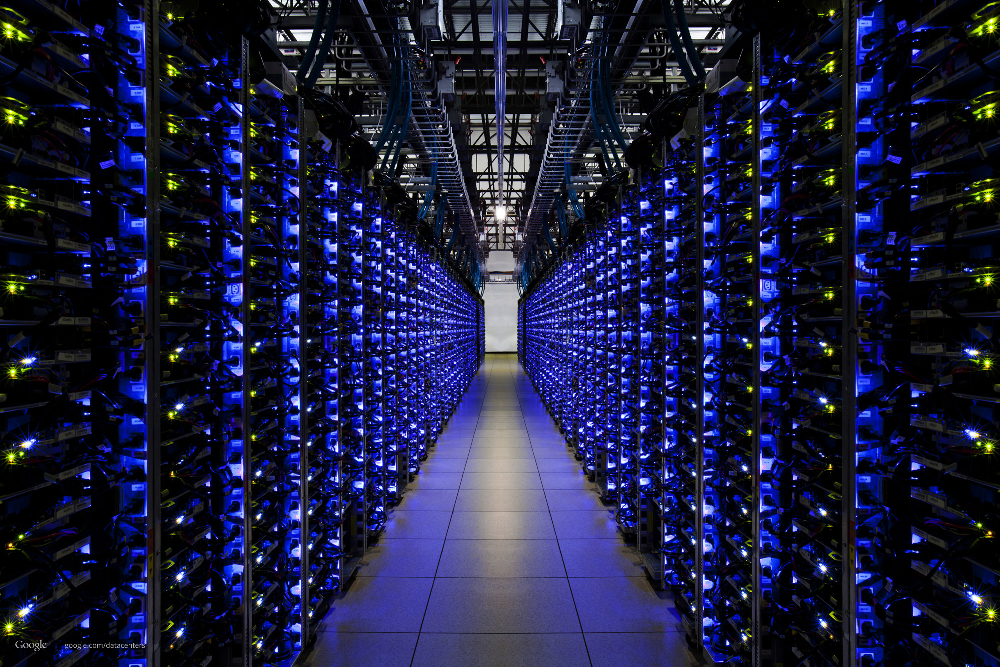
Do you want to be in the data center business?
Philips’ healthcare division gives providers the ability to analyze and store 15PB of patient data and receive real-time diagnostic insights that directly benefit a patient’s care. Major League Baseball has created Statcast, a tool that analyzes the previously intangible features of the game, providing a real-time, in-depth analysis of every play and forever changing how players are evaluated.
Hadoop distribution vendors, however, don’t see this as an all or nothing proposition and think that a hybrid approach to data infrastructure is the best of both worlds. But when looking at the idea of data gravity, if all of the data needs to be close together, how can the hybrid approach be effective? While it may work for applications that are fragmentable across a network, the very principal of data gravity makes hybrid deployment uniquely inappropriate for big data infrastructure.
Security
Larger companies in highly regulated industries are quick to mention security and compliance as a key deterrent from moving to the cloud. CIOs fear that because they don’t own the cloud, they don’t control who is accessing its data. But the reality is that cloud vendors are using some of the most cutting-edge technology and practices to handle everything from identity and access control, logging and monitoring, vulnerability analysis, data protection and encryption.
Organizations can point to compliance issues – whether geographic or industry specific – with the cloud, but most major clouds have worked to build compliance right into it. This makes it much more secure than on-premise, which is built from scratch and compliance is only one of thousands of components the architects considers. A simple look at AWS, GCP or Azure’s approach to security and compliance clearly shows that it is more comprehensive than what a typical enterprise could achieve within their own data centers.
Price, Performance
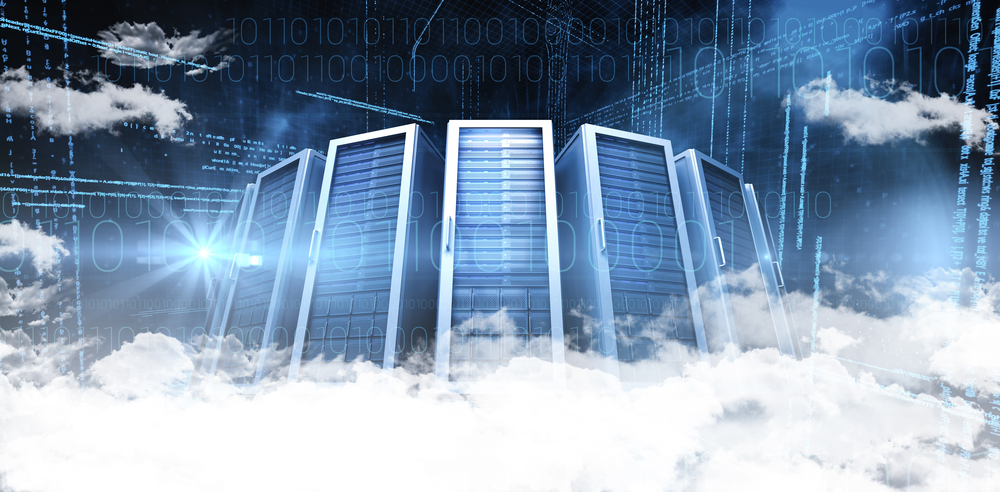
(vectorfusionart/Shutterstock)
There are more mundane arguments to migrating big data to the cloud, but they bear repeating. While its accepted that, in operation, the cloud is less expensive than on-premise data centers, the upfront cost and complexity of migrating to the cloud far outweighs the long-term savings. But with the elastic nature of the cloud, enterprises can size the infrastructure to fit the actual usage instead of peak usage. This single ability of the cloud substantially changes the total cost of ownership (TCO). These savings become more significant when looking at big data analysis, due to its bursty nature.
A recent study by Accenture shows that the elasticity and the availability of cheap resources on the cloud make performance a non-issue, and the price-performance metrics come out to be in favor of a cloud deployment . As competition continues to heat up in the cloud, prices will continue to decrease, making it the most budget-friendly option.
Agility and Self-Service
The cloud offers a huge opportunity for enterprises. For the first time, we have the ability to turn the infrastructure model on its head.
On-premise deployments require building infrastructure first and then deploying software and open-source distributions on top of them. This approach involves altering the infrastructure whenever the big data workloads change, as well as extensive and exact planning around forecasting usage. Cloud, on the other hand, allows users to change the infrastructure on the fly to match workloads, making it inherently more agile for supporting big data workloads, which change all the time.
With the market moving toward self-service big data offerings, enterprises need to ensure that their infrastructure scales as it is opened to more users and use cases. Cloud offerings can use the near infinite, elastic compute resources to do this more effectively than fixed data center compute. Self-service platforms are much easier for IT teams to implement on the cloud, especially when combined with a SaaS model.
Migration
With the increasingly fast pace data gravity is moving to the cloud, there is a dramatic shift away from on-premise data centers. The only remaining question is how to handle the migration. The market offers two very distinct paths to the cloud. The first is the lift-and-shift approach of replicating in-house big data deployments in the cloud to avoid re-architecting them entirely. This approach is expounded by distribution vendors as a cheaper and faster road to migration, but when applications are lifted and shifted, they can’t take full advantage of cloud-native features.
The second (and only) way the migration should be done is to use big data infrastructure built specifically for the cloud. The elasticity, speed and performance of cloud-first big data infrastructure is unparalleled, and using that approach maximizes the use of cloud features to enable IT teams to provide a self-service platform to their users. This empowers them to handle all types of data projects at scale in an affordable and efficient way.
About the author: Ashish Thusoo is the CEO and co-founder of Qubole, a cloud-based provider of Hadoop services. Before co-founding Qubole, Ashish ran Facebook’s Data Infrastructure team; under his leadership the team built one of the largest data processing and analytics platforms in the world. Ashish helped create Apache Hive while at Facebook.
Related Items:
Inside the Panama Papers: How Cloud Analytics Made It All Possible
Five Reasons Machine Learning Is Moving to the Cloud