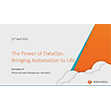
Data as a Service: Where It Works, Where It Still Doesn’t
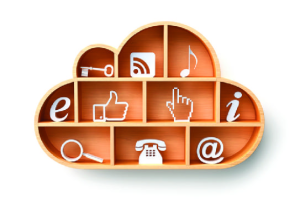
The latest craze in the business intelligence market today is data as a service (DaaS), i.e. the idea that data or analysis can be sold and ‘served’ to end users on a per-usage basis, with data management, storage and integration handled by the vendor rather than the end user. Data is supplied “on-demand” via cloud platforms, as opposed to the traditional models in which the data remains in the customer’s hands, and the vendor provides the tools that make it easier to access and explore.
While this growing trend certainly carries significant promise, it is important to distinguish between two different meanings of DaaS: when it comes to providing insight based on data which is possessed by the vendor itself (we’ll refer to this as data brokerage), DaaS has many potential benefits; on the other hand, the picture becomes more complicated when it’s the customer’s own data that is being exported to third parties who control both its storage and the underlying structure in which it is managed (we’ll refer to this as cloud business intelligence).
Rise of the Data Brokers
Data brokers, which Gartner predicts will gain significant traction in providing access to big data for enterprises, are service providers that deliver data which can be used to support or drive business decisions. This data is external to the organization that has purchased the service — e.g., financial, social or open government data. The data broker collects it, models it into a useable form, and provides access to consumers who can access its cloud platform to use this data in the course of their business operations.
I believe that this form of DaaS can deliver meaningful business value for organizations: it is beneficial for both parties involved and provides a service which many companies would otherwise not have been able to create for themselves.
Most companies–even those with an in-house BI department–don’t have the necessary infrastructure to deal with truly big data, both in terms of procuring and storing the data and in terms of effectively analyzing it. Furthermore, there is no real reason for a manufacturing company (for example) to invest heavily in building in-depth analyses of social media outliers. Their need for this data is limited in time and scope, and so it makes sense for them to purchase it from an external provider as their business demands, rather than devoting extensive resources to reach the same results internally.
When we are dealing with data that is produced outside the organization, it could often be simpler and more cost-effective to outsource the related services, rather than store and process it locally, unless you want to mesh it with your own proprietary data. Of course, there are cases when you would also want to handle external data sources in-house (for example, to cross-reference them more efficiently against your own records), but I believe this to be the exception and done by more advanced organizations, and not the rule.
The Challenge of Cloud BI
The second type of services often dubbed ‘DaaS’ are what we will refer to as cloud BI: these are software companies that deliver business intelligence platforms as cloud applications. These systems require the customers (or end users) to upload all their data to the vendor’s cloud storage and from there to “leave it all to the vendor”–meaning the gritty work of modeling the data, transforming it and preparing it for analysis, dashboard reporting and data discovery. In these cases the service pertains to the customer’s own data, sometimes in combination with cloud data, rather than data provided by the vendor.
These types of DaaS tools also have their benefits: many companies might prefer not to handle the daily struggle to turn their data into something that is easily serviceable for end users — as well as dealing with software and all the other benefits traditionally associated with SaaS and cloud applications.
However, unlike data brokers, here there are certain obstacles that still pose a challenge for these services, and should be considered before one turns to a cloud solution.
The first challenge is scalability. Cloud BI solutions typically rely on per-usage subscription models, which seem great when a business is working with small amounts of data. But since there is somewhat of a consensus that in the near future, the typical organization will be dealing with much more data than it does now, every company has to ask itself not how much data it currently has, but how much it might have in a few years’ time. In these cases the costs of cloud storage could become prohibitive — for example, storing terabyte-scale data on Amazon Redshift could incur an annual fee of tens to hundreds of thousands of dollars. To this you must add the costs and latencies associated with transferring large amounts of data over the world wide web. According to the current pricing of cloud storage, it is a less than optimal solution for Big Data, particularly if this data is frequently updated.
A second issue that needs to be addressed is data ownership. Corporate data can often be sensitive, as well as bound by legal obligations regarding its storage and permissions to access and modify it, with one notable example being the healthcare industry, wherein data related to patients is bound by various restrictions that stem from HIPAA requirements. Transferring this data to third parties could raise legal and contractual issues, which must be worked out with the vendor beforehand to prevent complications down the road.
A third consideration is data management. The way data is modeled and structured can significantly affect the types of analyses that can be performed on it, and the way different data sources can be joined, compared and referenced. Many cloud BI providers promise to make their customers lives initially simpler by providing these services for them, but it makes the end-users dependent on the vendor for any schema changes that might need to be made when adding additional sources, or in order to optimize performance. Leaving these matters to the vendor could still be a good fit for certain companies, such as those seeking a “quick fix” for visualizing their data, but might be less suitable for users who want a more flexible and agile analytics tool.
The Cloud is not for Everyone (Yet)
When it comes to DaaS, as with any emerging technology, the terminology used to describe it can be muddled and confusing. Hence it is important to make the distinction between external data as a service versus your own data as a service, and to understand the strengths and limitations of each before jumping on the DaaS bandwagon.
I believe that the ‘data broker’ scenario presents a relatively simple use case, and provides clear value for companies who need to access large external datasets on a limited basis; whereas cloud BI software also bears a lot of promise, but there are still certain challenges it needs to overcome before it becomes the go-to solution for business intelligence.
However, and to end on an optimistic note–I would venture to guess that within a few years, as more vendors enter the space and the industry and user-base mature, we are likely to see many of these challenges resolved.
About the author: Adi Azaria is a cofounder of business intelligence software provider SiSense. Azaria is a passionate entrepreneur, author, computer scientist and established thought leader. Adi has used his extensive experience to help the company triple its growth for four years in a row, as well as raise tens of millions of dollars in investment.
Related Items:
Peering Into Computing’s Exascale Future with the IEEE
Tracking the Rapid Rise in Cloud Data
Five Reasons Machine Learning Is Moving to the Cloud