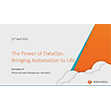
From Data Wrangling to Data Harmony
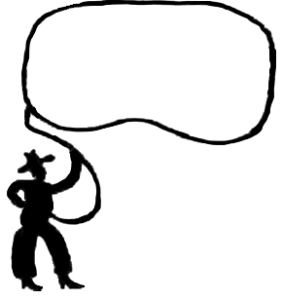
More and better automation tools such as machine-learning technologies are needed to free data scientists from mundane “data-wrangling” chores. Those tools would allow scientists to focus on gleaning insights from prepared data, a range of experts told the New York Times in a recent survey of the state of big data.
The newspaper reported that data scientists spend from 50 percent to 80 percent of their time organizing data, or “data janitor work,” before they could begin sifting through it for nuggets. It also noted that a group of startups are developing automation software and other tools to help gather and organize unstructured data from increasingly diverse sources.
“It’s an absolute myth that you can send an algorithm over raw data and have insights pop up,” Jeffrey Heer, a professor of computer science at the University of Washington and a co-founder of San Francisco-based data tools startup Trifacta, told the Times.
The data wrangling problem is growing as different types of unstructured data or data in varying formats are pouring in from sensors, online and from traditional databases. All these data must be cleaned up and organized before data analytics tools can be applied.
This is where automation tools come into play. The Times story cited the Silicon Valley startup, ClearStory Data, which has developed a tool for organizing data from a variety of sources, then presents the organized data in charts and graphs.
ClearStory leveraged the results of an Apache Spark project at the University of California at Berkeley called AMPLab to speed development of a data harmonization tool that merges diverse data streams into prepared data ready for diagnostic and discovery analysis.
The startup had broad experience with MapReduce, but found it to be too slow for data with a shelf life of only a few months. Hence, ClearStory leveraged the Berkeley lab’s work with Apache Spark to develop a data visualization tool.
Adopting the open source Hadoop processing engine ended up saving the startup millions of dollars in development costs while speeding up tool development. “Spark is pretty critical to how data harmonization functions at this point,” ClearData cofounder Vaibhav Nivargi told Datanami last month.
Along with data visualization, other startups are approaching the data wrangling problem with machine-learning software that flags potentially useful data for further investigation. “We want to lift the burden from the user, reduce the time spent on data preparation and learn from the user,” Joseph Hellerstein, chief strategy officer of Trifacta and a computer science professor at the UC-Berkeley, told the Times.
All these automation efforts aimed to make it easier to prepare and harmonize data, thereby speeding mainstream adoption of big data techniques. Indeed, the growing diversity of data coming from emerging networked sources like the Internet of Things is fueling demands for more and better automation tools as data scientists become inundated with information in a variety of formats from a plethora of new sources.
Recent items:
How Spark Helps ClearStory Achieve Data Harmony
Apache Spark: 3 Real-World Use Cases