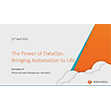
New Techniques Turbo-Charge Data Mining
While the phrase “spectral feature selection” may sound cryptic (if not ghostly) this concept is finding a welcome home in the realm of high performance data mining.
We talked with an expert in the spectral feature selection for data mining arena, Zheng Zhao from the SAS Institute, about how trends like this, as well as a host of other new developments, are reshaping data mining for both researchers and industry users.
Zhao says that when it comes to major trends in data mining, cloud and Hadoop represent the key to the future. These developments, he says, offer the high performance data mining tools required to tackle the types of large-scale problems that are becoming more prevalent.
In an interview this week, Zhao predicted that over the next few years, large-scale analytics will be at the forefront of both academic research and industry R&D efforts. On one side, industry has strong requirements for new techniques, software and hardware for solving their real problems at the large scale, while on the other hand, academics find this to be an area laden with interesting new challenges to pursue.
As Zhao told us, “High performance data mining techniques allow researchers and engineers to handle much bigger problems or many more problems in a shorter time. Both are game-changing factors for data mining applications. The first one resolves the large scale problems in data mining, for instance, allowing a finance institute to analyze their data sets of billion samples in just a few minutes. The second one facilitates rapid model development and near real time analytics, which are also of great significance in data mining industry. Due to its importance, SAS, IBM, SAP, R Community all have ongoing projects on high performance data mining.”
Zhao, along with co-author Huan Liu from Arizona State University detailed their findings in a recent book called, “Spectral Feature Selection for Data Mining.” As Zhao explained, “Spectral feature selection studies how to use the extracted spectrum information to objectively evaluate feature relevance. It is a general framework for unsupervised, supervised, and semi-supervised feature selection. Based on the framework, families of novel feature selection algorithms can be developed to address the challenges from in real applications.”
For instance, spectral feature selection can be used to address large scale feature selection problems through parallel processing, and can address the small sample problems through multi-source feature selection.
Zhao says that although the technique has only been developed recently, it has been applied in various areas for solving real problems. For instance, a group of Chinese researchers from the Shanghai Jiaotong University applied the technique in genetic analysis to assist their ovarian cancer study. And Dr. Chang’s research group from the Biodesign Institute at Phoenix used the technique to study the toxic effect of TiO2 nanoparticles to aquatic creatures such as the zebra fish.
In industry, a version of spectral feature selection has been implemented by SAS as a high performance analytics procedure under the SAS High-Performance Analytics product. As Zhao told us, “Since we published the first paper for spectral feature selection in 2007, our works on spectral feature selection has obtained over a hundred citations from researchers over the world, which demonstrates the big impact of our work on spectral feature selection has generated.” He reiterated his belief that as time goes on, the spectral feature selection technique will find more applications in both academic and industry, and contribute more to the whole data mining community.
In the book, Zhao and Liu provide examples of how spectral feature selection can be harnessed to achieve multi-source feature selection to assist Microarray based genetic analysis. A significant problem related to the Mircoarray data is that its sample size is usually very small. Multi-source feature selection helps researchers to incorporate information from outside to enrich information, therefore improves the reliability of the analysis. Another example of the application of spectral feature selection is cited in the book that involves a large finance institution, which used the techniques to perform dimensionality reduction and variance analysis for their billion-record data sets.