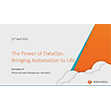
The Future of Data Science
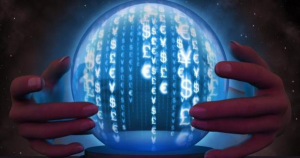
What does the future of data science look like? If you’re Forrester analyst Mike Gualtieri, the future of data science is all about predictive models—lots of them running in semi-automated fashion at truly massive scale. But will that eliminate the need for data scientists?
Gualtieri laid out his vision on the future of data science and the role that data scientists will play in it during a recent webinar sponsored by Skytree, a developer of software for automating predictive analytics using machine learning models. That in itself should give you a hint as to where the Forrester analyst was going with his predictions.
The past of data science was all about descriptive analytics, or describing what has already taken place, Gualtieri says. But the future of data science will hinge on advanced analytics—specifically using predictive analytics and real-time analytics in pursuit of business goals, such as improving the customer experience, improving products and services, and reducing costs and churn.
“What data scientists use today is a combination of statistical and machine learning algorithms to find patterns in the predictive models they use,” he says. “Traditionally, they’ve had to have a strong mathematical foundation. You hear many data scientists saying ‘I did the math.’ That also refers to running statistical machine learning algorithms.
“In the future,” he continues, “the tools are going to de-emphasize the mechanics of doing machine learning. So the data scientists are going to be more creative about the types of models they create, freeing them up to have more time for curiosity to discover new things that may be of value.”
Data scientist are relatively hard to find today, especially in tight job markets like the Silicon Valley. In the future, advances in data science tools will help leverage the existing data science talent to greater effect, Gualtieri says.
“Data scientist face an unprecedented demand for more models, more insights,” he says. “There’s only one way to do that: They have to dramatically speed up the insights to action. In the future data scientists must become more productive. That’s the only way they’re going to” get more value from the data.
Eliminating bottlenecks in the data science process–such as data preparation, which can suck up 80 percent of a data scientist’s time–is a huge goal of the data analytics community. According to Gualitieri, big strides will be made in this area.
“We’re seeing a number of vendor tools focused on faster data preparation–acquiring those sources and preparing them,” he says. “That’s one of the keys for them – they have to be able to get faster algorithm and data iterations.”
Another barrier to data science productivity that will come down are walls separating data. “The data silos have to disappear,” he says. “That’s a key challenge of data scientists, is getting the data form those hundreds of different applications into a centralized store, and then having a platform that can not only store it but can scale to run algorithms on that massive data set.”
In the future, the scalability of advanced analytics applications will need to be absolutely massive to support the humongous data sets that data scientists will throw at them. “The scale should not limit the analytics,” Gualitieri sys. “Data scientists are used to sampling. Oftentimes that’s statistically accurate, but oftentimes it’s not. Oftentimes you get a lot more accuracy when you use a lot more data, and there’s a lot more data to use.”
Gualtieri envisions data science teams adopting massive model automation (MMA) tools to automate the model building and running process. (Skytree, by the way, is in the middle of building such an environment.)
“What if you had a model for every one of your customers? Three to four million models? That’s massive,” Gualtieri says. “There’s not going to be a data scientist sitting in front of the computer and creating three to four million models from an old fashion toolset. So the data scientist from the future, instead of walking through that iterative model process, instead will configure a massive model automation tool to automate the modeling process and to better iterate themselves.”
While the tools will improve, Gualtieri doesn’t seem to buy into the notion that we won’t need data scientists in the future, that the machine learning tools will be so automated and advanced that mere data analysts can run them without the supervision of somebody in a white lab coat and multiple PhDs.
“To be clear–a business person [may] create certain kinds of models, maybe for analytical reporting,” he says. “But a data scientist is always going to be needed because that depth and understanding of the model building process. It’s not getting easier–it’s getting more complicated, and they have to become more productive.”
That’s not to say that the data scientist of the future will need a huge range of skills. Today, data scientists often are expected to be a one-stop shop of mathematical, business, and technology acumen, which is one reason why they’re so hard to find. In the future, data science tools will help business people do some of the work of data scientists (although obviously not the hard-core model building part).
“People often say that data scientists have to have domain knowledge,” Gualtieri says. “I wouldn’t say they have to. I would say that the reason why people say that it’s good for a data scientist to have domain or business knowledge is because oftentimes it’s business people don’t have the imagination to have the hypothesis to come up with something they could predict in a business process or a customer journey.”
In the future, that won’t be the case. “We’re seeing business people understand there’s possibilities that they can predict,” Gualtieri says. “But they don’t need a data scientist to spend a huge amount of time in the business trying to find hypothesis. In many organizations, the business has more hypothesis than the data scientist can actually handle.”
Related Items:
The Rise of Predictive Modeling Factories
Predictive Analytics Now In Reach Of the Average Enterprise, Forrester Says