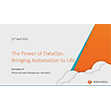
May 22, 2019
Spark NLP Becomes the World’s Most Widely Used NLP Library in the Enterprise Within 18 Months
DELAWARE, May 22, 2019 — The annual O’Reilly report on AI Adoption in the Enterprise, released in February 2019, is a survey of 1,300 practitioners in multiple industry verticals, which asked respondents about revenue-bearing AI projects their organizations have in production and also to list all the ML or AI frameworks and tools which they use.
Spark NLP library was listed as the 5th most popular across all AI frameworks – following only scikit-learn, TensorFlow, keras, and PyTorch. It was also by far the most widely used NLP library – twice as common as spaCy, which was the closest on this ranking.
- Accuracy. More accurate than spaCy, Stanford CoreNLP, nltk, and OpenNLP, due to implementation of recent deep learning networks and embeddings
- Speed. NLP pipelines can run 2-3 orders of magnitude faster for training of custom NLP models
- Scalability. Built on Apache Spark ML, Spark NLP can scale on any Spark cluster, on-premise or in any cloud provider.
- Production-grade codebase. Built for enterprises, in contrast to research-oriented libraries like AllenNLP and NLP Architect.
- Permissive open source license. The library can be used freely, including in a commercial setting.
- Full Python, Java and Scala APIs. Supporting multiple programming languages and enables to take advantage of the implemented models without having to move data.
- Frequent Releases. Released about twice a month – there were 26 new releases in 2018.
John Snow Labs SPARK NLP 2.0 – the biggest release to date
This Spark NLP 2.0 release merges 50 pull requests, improving accuracy and ease and use. It’s the largest single release since the library was first introduced.
Spark NLP is the first library to have a production-ready implementation of BERT embeddings for named entity recognition. Here are the biggest enhancements in this release:
- Revamped and enhanced Named Entity Recognition (NER) Deep Learning models to a new state of the art level, reaching up to 93% F1 micro-averaged accuracy in the industry standard.
- Word Embeddings as well as Bert Embeddings are now annotators
- TensorFlow version upgrade and use of contrib LSTM Cells
- Performance and memory usage improvements
- Revamped and expanded pre-trained pipelines list, and new pre-trained models for different languages and new example notebooks
- OCR module improvements for increased accuracy.
Source: John Snow Labs