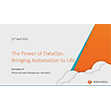
Zilliz Unveils Game-Changing Features for Vector Search
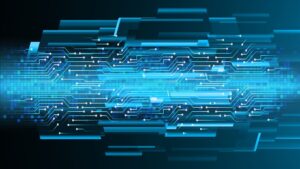
(Titima-Ongkantong/Shutterstock)
Vector databases have emerged to become an integral component of many AI applications. However, as AI applications continue to grow, vector databases are presented with more complicated use cases. This requires vector databases with high levels of recall, low latency, and high throughput.
With the release of Milvus 2.4 by Zilliz, a vector database management system, users can now benefit from enhanced vector search capabilities. The new release features GPU Indexing capability leveraging NVIDIA’s cutting-edge CUDA-Accelerated Graph Index for Vector Retrieval (CAGRA).
The GPU-based graph indexing technology significantly surpasses traditional CPU-based indexes like HNSW, which has long been a top-performing index for vector similarity search. This enhancement is ideal for building similarity search applications requiring minimal latency.
“We are thrilled to introduce the next-gen GPU indexing in Milvus 2.4, a release that underscores our commitment to advancing the field of vector database technology, with GPUs” said Charles Xie, CEO at Zilliz. “These features empower developers with unparalleled capabilities, enabling them to build highly efficient and scalable applications that leverage the combination of vector search and GPUs.”
Milvus 2.4 also features multi-vector search capabilities for improved management of multiple vector searches and retrieving within its framework. This helps with one of the biggest challenges of vector databases – scalability.
The multi-vector search simplifies searches and improves recall of retrieving information. In addition, this feature makes integrating and optimizing custom reranked models straightforward and enables users to model data more efficiently for real-world applications. Users can also use prebuilt reranking algorithms to help further enhance retrieval performance.
Another challenge with vector databases is indexing and query optimization for large datasets, such as documents or videos segmented into vectorized chunks. Aggregate searching by attributes is time-consuming and resource-intensive.
The new Grouping Search feature in Milvus 2.4 offers a way to simplify aggregation so users can retrieve top results by the group fields directly, removing the need for any custom code. This feature boosts resource efficiency, and developer productivity and allows for a more effective decision-making process.
Zilliz has also expanded its Hybrid Search to include the much-anticipated beta version of sparse vector embeddings. This feature enables efficient and semantically rich approximate nearest neighbor (ANN) searches for statistical models such as BM25 and neural models like SPLADEv2.
Scheduled for general availability later this year, this beta version aims to enhance the accuracy of text search using hybrid search methodologies, delivering more relevant search results. It also helps users transition from keyword-based systems. Other enhancements in Milvus 2.4 include a tantivy-based inverted index and fuzzy matching for improved scalar query performance.
Zilliz recently advanced its vector database tech with the unveiling of the latest evolution of Zilliz Cloud, a highly performant, scalable, and fully managed vector database platform built on open-source Milvus. The release of Milvus 2.4 further reinforces Zilliz’s commitment to continuous improvement in its quest to enhance search capabilities for vast datasets.
Related Items
How Real-Time Vector Search Can Be a Game-Changer Across Industries
Zilliz Vector Database Research Featured at VLDB 2022
AWS Adds Vector Capabilities to More Databases