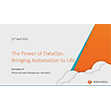
GenAI Doesn’t Need Bigger LLMs. It Needs Better Data
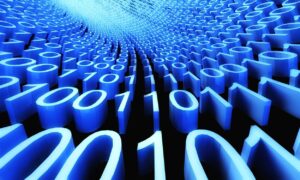
(Leszek-Glasner/Shutterstock)
An exorbitant amount of time and energy is being spent developing and talking about the technology that goes into large language models. While the tech is indeed impressive, businesses that are building generative AI applications realize that what’s really moving the needle in GenAI is the availability of high quality and trusted data.
The fact that GenAI is putting a spotlight on data quality issues shouldn’t come as a big surprise. After all, data and AI are inseparable at the end of the day, as AI is simply one distillation of data. But sometimes hard lessons need to be relearned after a period of over stimulation, such as the current GenAI craze.
The good news is that many of the same tools and techniques that the market has developed for ensuring data quality for advanced analytics and machine learning projects also work with the newfangled GenAI applications. That’s helping to drive business for Monte Carlo, a provider of data observability software.
“Obviously, most of the teams that we work with cared about data reliability before, otherwise they wouldn’t be working with us,” Monte Carlo Co-founder and CTO Lior Gavish said. “But when [data] comes front and center through a chat interface that any layperson can use and potentially can be exposed to millions of their customers, the stakes are higher, and so it becomes even more important.”
There’s been a definite learning curve when it comes to data quality, as companies move their GenAI applications from proof of concept into production, said Monte Carlos CEO and Co-founder Barr Moses. The education process has not been an entirely positive experience for companies that have not invested in systems to observe and improve data quality, she said.
“Folks are building proof of concepts and then they’re putting it in front of internal users typically, and the data is wrong,” she said. “That creates a very bad experience and actually puts them back many months behind in terms of actually being able to use it.”
Some companies are realizing that their data is so untrustworthy that they can’t even get to the POC stage, Moses said. “They need to get their data in order first, and they recognize that,” said Moses, a 2023 Datanami Person to Watch.
While GenAI requires some new tools, many of the investments that companies made for earlier advanced analytics and machine learning projects can be reused for GenAI. Companies that have parked their data in a Databricks or Snowflake repository are leveraging those data platforms to build their GenAI applications, Moses said.
“Instead of having a fully separate infrastructure just for generative AI, people are using the existing infrastructure and strengthening or augmenting it in order to build these generative AI products,” Moses said. “Obviously, wherever your data is today, just became a lot more important.”
Monte Carlo, which was founded in 2019, uses a variety of statistical methods to detect when problems may be arising in customers’ data pipelines. Traditionally, the company’s tech was deployed in ETL/ELT pipelines moving data from transactional systems into data warehouses. As GenAI becomes more popular, the companies are using Monte Carlo to help make sure that what goes into retrieval augmented generation (RAG) and fine-tuning workflows are accurate.
Monte Carlo has been involved in a number of GenAI projects. Cereal manufacturers, healthcare companies, and financial services firms are all looking to the company’s software to help them keep their data pipelines running well and able to feed high quality and trusted data into GenAI applications like chatbots and recommendation engines, the executives said.
The whole experiment has served as a reminder to companies how important data is to their operations, Gavish said.
“The thing they can differentiate with is data, their own proprietary data,” he said. “To a degree, what’s new is old. You have to get your data in order, in order to build generative applications on top of it. And to do that, you have to incorporate your internal data into the model, be it through RAG or fine tuning.
“But you have to somehow wedge your data in the model, and then it’s basically back to basics, right?” he continued. “How do you figure out what data you have, where is it, how good it is, and then how do you keep it trusted and reliable? We’re not solving all these problems, but we’re definitely focused on the reliability and trust part.”
Monte Carlo embraces the new role it’s playing, particularly when it comes to helping to address some of the various issues LLMs have around hallucinations and nondeterministic outcomes, Gavish said.
“And so really the reliability of the underlying data becomes even more critical, because that’s the mitigation,” he said. “At the end of the day, people are doing RAG, among other reasons, because models in and of themselves and not super accurate. So RAG is a way to make them more accurate, but then that kind of doesn’t work if the data isn’t trusted.”
Related Items:
Data Quality Is Getting Worse, Monte Carlo Says
Data Quality Top Obstacle to GenAI, Informatica Survey Says