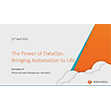
How Acceldata Helped T-Mobile’s Data Modernization Strategy
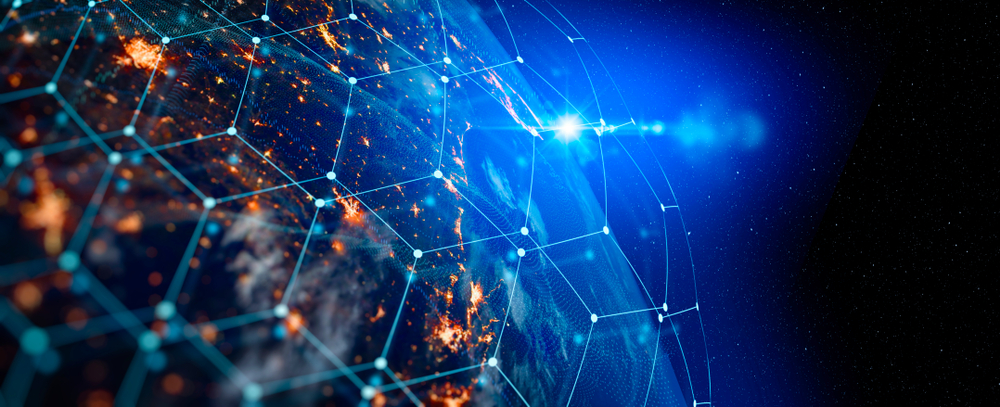
(greenbutterfly/Shutterstock)
When T-Mobile started migrating some of its data estate from an on-prem Hadoop system to cloud-based data platforms, it found the move liberating. But as it settled into a hybrid-cloud world, T-Mobile realized costs were getting out of hand. That’s when it brought in data observability vendor Acceldata to get a better handle on its data.
Like many large enterprise, T-Mobile relied on a traditional data warehouse to surface critical information to inform business decisions. But as the big data boom commenced about a decade ago, it found relational databases could no longer scale to meet its data storage and processing needs.
Around 2015, T-Mobile adopted the Apache Hadoop platform. The telecommunications giant found that its on-prem Hortonworks Data Platform (HDP) cluster opened up new horizons in terms of the size of the network event data it could collect, store, and process, according to Vikas Ranjan, senior manager of data and analytics engineering at T-Mobile.
“Hadoop was definitely a game-changer in terms of how people were able to unlock the possibility of big volume data sets, high complexity data sets, and distributed data processing,” Ranjan says. “Going from 2TB of data per day to more than 1PB of data per day processing became a reality for us.”
The early days of T-Mobile’s Hadoop experience went very well, Ranjan says. The company adopted powerful frameworks like Apache Spark and Apache Hive to process network event data. The event data arrived in proprietary flat-file like formats, and T-Mobile transmitted them into industry standard Parquet.
But the big data challenges that drove T-Mobile into the arms of Hadoop in the first place refused to go away. With the growth of Web traffic and advent of new technologies like 5G and virtual reality, the data just kept getting bigger, with greater variability. Managing the Hadoop cluster amid this growth became a challenge in its own right, Ranjan says.
“As we started doing a lot more analytics and modernization of things on Hadoop, we ran into scalability issues,” he says. “About 2019 we saw a tipping point on what Hadoop can do with some of the limitations and some of the gaps and where the data was going in terms of scale.”
T-Mobile needed to process a large number of very small files, on the order of one to two trillion network events per day. However, HDFS isn’t very good at handling large number of small files, as it leads to namenode and memory utilization issues that drag down performance.
Another issue was machine learning and AI. While Hadoop data lakes were good for processing and analyzing data, they’re not the best platforms for running machine learning and AI, Ranjan says.
“Hadoop was working for us, but it was not giving us the advanced analysis capabilities, the machine learning capabilities,” he says. “Hadoop is better for data lake and data processing, but not as good for a lot of use cases.”
So in 2019, T-Mobile started exploring how it could augment its data approach. Data creation continued to grow exponentially thanks to 5G and the metaverse, but Hadoop’s data scalability issues were causing it to miss SLAs in terms of making data accessible.
“The most critical currency is time,” Ranjan says. “We don’t have patience to do things four hours from now, or 12 hours from now or 24 hours from now. You want to solve the problems as they’re happening.”
T-Mobile ended up taking a two-pronged approach to its data platform modernization. One branch stayed on prem, while another branch led to the cloud.
For T-Mobile’s most critical network event data, which resided on its 40PB HDP cluster, the company built a custom, Java-based in-memory data processing system that runs atop Kubernetes. That system runs on prem next to its Hadoop cluster, which T-Mobile continues to run for data persistence and some Spark and Hive workloads.
T-Mobile also started its cloud journey, around the year 2021. According to Ranjan, the company wanted the flexibility to run on all the major cloud platforms, including AWS, Microsoft Azure, GCP, Databricks, and Snowflake. Like its move from a traditional data warehouse to Hadoop, the move from Hadoop to the cloud was eye-opening.
“As we go into the cloud world, immediately we saw the benefits of cloud in terms of elasticity, in terms of agility,” Ranjan says. “There were things we could not do in our on-prem Hadoop system for months. Within days, we were able to innovate. We were able to ideate, come up with new use case, on board new users, given them the art of possibilities in terms of AI and ML which were not available in the traditional Hadoop when we were working in our journey in the past.”
But, alas, the cloud turned out not to be the land of milk and honey. While T-Mobile increased its agility in the cloud and gained access to a host of new ML and AI tools, it came at a cost.
“The cloud works really, really well. But we don’t have an infinite budget,” Ranjan says. “We have very limited budgets now. We want to be very cost efficient, and the way the whole cloud is [billed] brings some very complex challenges in terms of how to manage the cost.”
As previously mentioned, T-Mobile’s data journey has not led away from Hadoop, which remains a critical data persistence layer for the company’s most important network data in the US. The company needed to get a better handle on costs, both with its on-prem data lake and new cloud repositories. That’s where Acceldata comes in.
“Acceldata is helping us with the overall observability,” Ranjan says. “Acceldata helped us with optimization of cost on cloud [and] on-prem Hadoop. I think there was a lot of wasting of the data we were storing. We have multiple petabytes of data that was not accessed. And then the whole tuning of Hadoop was very, very complicated and complex because this is a high-scale platform.
What attracted T-Mobile to Acceldata in the first place was its support for Hadoop, which is a platform that other data observability vendors do not support. According to Ranjan, the company liked Acceldata because it could provide a single pane of glass for all of its data estates, both on prem Hadoop and cloud data platforms.
“Our [proof of concept] was around Hadoop, and then from there we kind of started seeing that value and expanding,” Ranjan says.
While hasn’t yet gone into production with Acceldata for its Databricks implementation, the early POC shows promise, he says.
“What I really like about this is we were getting a single pane of view to get the cost of all your workspaces, broken down by the user, broken down by the workloads, for all the different Databricks implementations we have and the cluster,” he says. “It gives you everything in one place, so you don’t have to chase. You don’t have to go to different places. You don’t have to build your custom dashboards. It’s all in one place.”
Ultimately, Acceldata enabled T-Mobile to optimize its Hadoop platform, improving manageability and enabling it to hit its SLAs again. Considering that the pace of data creation and innovation shows no signs of letting up, having a tool like Acceldata likely will pay dividends for T-Mobile in the future.
Related Items:
Observability Platform Acceldata Goes Open Source
How T-Mobile Got More from Hadoop
The 5G Data Deluge Has Been Smaller Than Expected