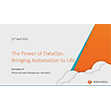
How to Maximize the Value of Data with Data Mesh
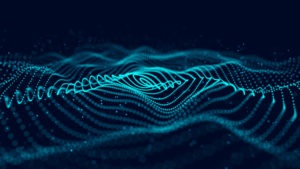
(Oleksii Lishchyshyn/Shutterstock)
It’s no secret that data today is more complex than it’s ever been, and it continues scaling at an unthinkable pace – global data creation is projected to grow to more than 180 zettabytes by the year 2025. As businesses try to keep pace with this explosion, as well as changing market conditions, it’s mission critical that they have easy access to data they can trust for analysis.
Of course, management solutions help enterprises with the processing and storage of this data, but the increase in both volume and complexity creates friction for IT teams and data offices tasked with the overwhelming responsibility of managing ever-growing data sets.
We’re at an inflection point in enterprise data management. As organizations aim to remove inefficiencies and bottlenecks in their data processes, the data mesh model offers a flexible framework that can help organizations be more competitive in 2023 and beyond.
The Modern Data Landscape
Many organizations use traditional data warehouses and BI platforms, but the challenge with centralized platforms is that they are monolithic in nature and require their own team of managers and administrators to govern them.
Continuously unloading data into centralized repositories means someone must take on the burdensome role of managing, curating, and delivering this data across teams, stretching thin the resources of those teams and creating the potential for bottlenecks. Large enterprises also often have massive amounts of data stored in on-premise data warehouses, which can take an inordinate amount of time to migrate to the cloud. These migrations can be draining on both time and financial resources.
While these systems have been ingrained as a part of everyday business intelligence solutions for decades, their benefits are now counterbalanced by the proliferation of data and its growing complexity.
The Core Pillars and Benefits of Data Mesh
One solution to overcoming these challenges is to embrace data mesh, an approach developed by Zhamak Dehghani that shifts data processes from a central data platform to a modern distributed architecture where organizations can truly unify data from disparate systems and sources.
Data mesh is a people and process-focused method that is centered on four guiding principles: domain-driven ownership, data as a product, self-service data infrastructure and federated computational governance. Each of these principles can be worked on individually as companies begin their transition to a data mesh framework, but each pillar is integral to the power of data mesh.
Pillar 1: Domain Ownership
Domain-driven ownership is the key differentiator between a data mesh architecture and a traditional, monolithic method of data collection. With a data mesh model, data is sectioned and controlled by the data experts of that business domain, for example, the HR team or marketing team. When data is pumped into a data lake or warehouse, the data is often separated from the experts, making this data less decipherable and clogging the entire system.
However, with data mesh, experts have control of the data and can harness their expertise to clean, enrich and translate the data, maintaining the data’s quality as well as streamlining the overall process of data cleaning and creating a more seamless process for the organization itself. The data experts understand the unique purpose of the data and how that set of data can meet the needs of that particular domain, and subsequently, meet the needs of the organization overall. Through data mesh, each organization can cater to its own data, while at the same time benefiting from a framework of overarching governance and the corporate-wide infrastructure.
Pillar 2: Data as a Product
Like domain-driven ownership, the ‘data as a product’ thought process is a similar approach to understanding data on a deeper level. By putting domain experts in charge of the data, data mesh views the data from a product lens, ensuring the data meets the accessibility, governance and needs of the organization, similar to how a product would be measured by its effectiveness and impact on the organization. Through a data mesh approach, data is given an overall vision and roadmap as well as a maintenance plan. By constantly measuring the data’s effectiveness, this model ensures organizations are constantly using their data and avoiding data swamps, large amounts of data that sit untouched, growing increasingly stale over time.
Pillar 3: Self-Service Data Infrastructure
To prevent each business domain from being overwhelmed with the task of managing their data, data mesh leverages a self-service infrastructure to promote healthy data systems across the organization, made possible by cloud.
The self-service model allows data to be seamlessly shared throughout the organization, enabling the business to foster innovation through a shared understanding of the data. The model abstracts data complexity for domain owners while simultaneously reducing friction for data consumers, fostering a deeper understanding of the data on all levels, and creating more opportunities for the data to be leveraged.
Pillar 4: Federated Computational Governance
Good data management must have data governance at its core. The data mesh model employs a federated approach to data governance, meaning it creates an environment that fosters both enterprise-wide authority as well as domain-specific need. To achieve this level of automation and integration throughout the data infrastructure, policy, classification, security, and quality are key.
Adopting a Data Mesh Model
For organizations currently struggling with a complex data landscape, the idea of transitioning to a data mesh model may seem daunting. However, the beauty of data mesh is that it can be implemented gradually based on the readiness of the organization.
As they say, ‘Rome wasn’t built in a day’ — and these changes don’t need to happen all at once. A major benefit of the data mesh model is it can be adopted domain by domain, pillar by pillar. Organizations can start with one business domain and progress to others after learning what works well and what challenges need to be addressed.
The key is to understand that data mesh is a cultural shift. Data mesh empowers subject matter experts to take ownership of their data, and the people and process components are the most important pieces to get right. Data leaders should take on the role of helping their teams embrace and drive cultural change. Once implemented, data mesh will enable organizations to better manage and access their data and make faster, better business decisions.
About the author: Jay Militscher is the Head of Collibra’s Data Office, coming to the company with over 20 years of leadership experience in data analytics, quality assurance, and security. Jay uses creative problem-solving to identify critical enterprise problems and opportunities, envision solutions, and lead the department to move the business strategy forward. Prior to joining Collibra, Jay was FactSet’s SVP of Corporate Insights & Analysis and served on several advisory boards. He measures his success through the success of his teammates, elevating, mentoring, and coaching them along the way.
Related Items:
Data Mesh Creator Takes Next Data Step
Data Mesh Vs. Data Fabric: Understanding the Differences
Data Mesh: What’s In It For The Business?