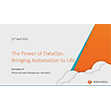
Why DataOps-Centered Engineering is the Future of Data
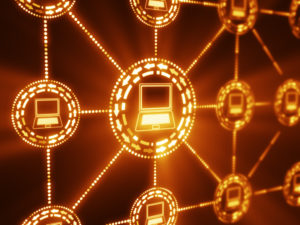
(3dreams/Shutterstock)
DataOps will soon become integral to data engineering, influencing the future of data. Many organizations today still struggle to harness data and analytics to gain actionable insights. By centering DataOps in their processes, data engineers will lead businesses to success, building the infrastructure required for automation, agility and better decision-making.
DataOps is a set of practices and technologies that operationalizes data management to deliver continuous data for modern analytics in the face of constant change. DataOps streamlines processes and automatically organizes what would otherwise be chaotic data sets, continuously yielding demonstrable value to the business.
A well-designed DataOps program enables organizations to identify and collect data from all data sources, integrate new data into data pipelines, and make data collected from various sources available to all users. It centralizes data and eliminates data silos.
Operalization, through XOps including DataOps, adds significant value to businesses and can be especially useful to companies deploying machine learning and AI. 95% of tech leaders consider AI to be important in their digital transformations, but 70% of companies report no valuable return on their AI investments.
With the power of cloud computing, business intelligence (BI) – once restricted to reporting on past transactions – has evolved into modern data analytics operating in real-time, at the speed of business. In addition to analytics’ diagnostic and descriptive capabilities, machine learning and AI enable the ability to be predictive and prescriptive so companies can generate revenue and stay competitive.
However, by harnessing DataOps, companies can realize greater AI adoption—and reap the rewards it will provide in the future.
To understand why DataOps is our ticket to the future, let’s take a few steps back.
Why Operationalization is Key
A comprehensive data engineering platform provides foundational architecture that reinforces existing ops disciplines—DataOps, DevOps, MLOps and Xops—under a single, well-managed umbrella.
Without DevOps operationalization, apps are too often developed and managed in a silo. Under a siloed approach, disparate parts of the business are often disconnected. For example, your engineering team could be perfecting something without sufficient business input because they lack the connectivity to continuously test and iterate. The absence of operationalization will result in downtime if there are any post-production errors.
Through operationalization, DevOps ensures that your app will evolve instantly, as soon as changes are made, without you having to pause work entirely to modify, then relaunch. XOps (which includes DataOps, MLOps, ModelOps, and PlatformOps) enables the automation and monitoring that underpin the value of operationalization, reducing duplication of processes. These features help bridge gaps in understanding and avoid work delays, delivering transparency and alignment to business, development, and operations.
DataOps Fuels MLOps and XOps Value
DataOps is the engine that significantly enhances the effectiveness of machine learning and MLOps — and the same goes for any Ops discipline.
Let’s use ML and AI as an example. When it comes to algorithms, the more data the better. But the value of ML, AI, and analytics is only useful if that data is valid across the entire ML lifecycle. For initial exploration, algorithms need to be fed sample data. When you reach the experimentation phase, the ML tools require test and training data; and when a company is ready to evaluate results, AI/ML models will need ample production data. Data quality procedures are possible in traditional data integration but built upon brittle pipelines.
As a result, when enterprises operationalize ML and AI, they are more frequently relying on DataOps and smart data pipelines that enable constant data observability and ensure pipeline resiliency. In fact, all Ops disciplines need smart data pipelines that operate continuously. It’s this continuity that fuels the success of XOps.
Delivering XOps Continuity with DataOps
DataOps delivers the continuous data that every Ops discipline relies on. There are three key pillars of DataOps that make this possible:
- Continuous design: Intent-driven continuous design empowers data engineers to create and modify data pipelines more efficiently and on an ongoing basis. With a single experience for every design pattern, data engineers can focus on what they’re doing versus how it’s being done. Fragments of pipelines can also be reused as much as possible thanks to the componentized nature of continuous design.
- Continuous operations: This allows data teams to respond to changes automatically, make shifts to new cloud platforms and handle breakage easily. When a business adopts a continuous operations strategy, it allows for changes within pipelines to deploy automatically, through on-premises and/or cloud platforms. The pipelines are also intentionally separated whenever possible, making them easier to modify.
- Continuous data observability: With an always-on Mission Control Panel, continuous data observability eliminates blind spots, makes information within the data more easily understandable, and helps data teams comply with governance and regulatory policies.
The Future of Data
In the future, data teams will harness a macro understanding of data by monitoring evolving patterns in how people use data- all of data’s characteristics will be emergent.
Data engineering that takes a DataOps-first approach will help successfully and efficiently achieve this goal. Moving forward, data consumers should demand operationalization, and data engineers should deliver operationalization. That is the only way that data will truly become core to an enterprise, dramatically improving business outcomes.
About the author: Girish Pancha is a data industry veteran who has spent his career developing successful and innovative products that address the challenge of providing integrated information as a mission-critical, enterprise-grade solution. Before co-founding StreamSets, Girish was the first vice president of engineering and chief product officer at Informatica, where he was responsible for the company’s corporate development and entire product portfolio strategy and delivery. Girish also was co-founder and CEO at Zimba, a developer of a mobile platform providing real-time access to corporate information, which led to a successful acquisition. Girish began his career at Oracle, where he managed the development of its Discover Business Intelligence platform.
Related Items:
Demystifying DataOps: What We Need to Know to Leverage It
Data Pipeline Automation: The Next Step Forward in DataOps
Sports Follies Exemplify Need for Instant Analysis of Streaming Data