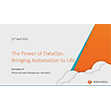
In Pittsburgh, Two-Step Machine Learning Process Sorts Rare Stamps
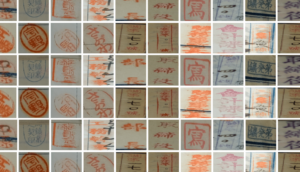
Setting aside the relatively recent rise of electronic signatures, personalized stamps have been a popular form of identification for formal documents in East Asia. These identifiers – easily forged, but culturally ubiquitous – are the subject of research by Raja Adal, an associate professor of history at the University of Pittsburgh. But, it turns out, the human expertise required to study these stamps at scale was prohibitive – so Adal turned to supercomputer-powered AI to lend a hand.
“[From] the perspective of the social sciences, what matters is not that these instruments are impossible to forge—they’re not—but that they are part of a process by which documents are produced, certified, circulated and approved,” Adal explained in an interview with Ken Chiacchia of the Pittsburgh Supercomputing Center (PSC). “In order to understand the details of this process, it’s very helpful to have a large database. But until now, it was pretty much impossible to easily index tens of thousands of stamps in an archive of documents, especially when these documents are all in a language like Japanese, which uses thousands of different Chinese characters.”
Specifically, Adal was working with a document archive from the Japanese company Mitsui Miike Mine that constituted one of the largest repositories of business documents from modern Japan, spanning fifty years and tens of thousands of documents – including 5,056 images of stamps. Documenting these thousands of diverse stamps would be a gargantuan task for a number of highly specialized research assistants – so Adal reached out to Paola Buitrago, director of AI and big data at the neighboring PSC.
The Mitsui Miike Mine database posed problems for training a machine learning model, since many of the diverse stamps only appeared a few times – or even just once. So the team, instead, applied a two-step machine learning process: first, they trained the model to classify general objects; then, they layered on top of that a classification model to group the stamps, allowing the rare stamps to be grouped together.
Running this machine learning model took computational firepower – which, of course, the Pittsburgh Supercomputing Center had in-hand. Adal and Buitrago first took advantage of the GPUs onboard the Bridges supercomputer, and then, when it was retired in February, moved on to its successor, Bridges-2 – equipped with even more (and more powerful) GPUs for image analysis. Taking advantage of the resources, the team showed that repeatedly training the model nearly doubled precision (from 44.7 percent to 84.3 percent). Now, the team is looking at applying the model in other research areas.
To learn more, read the reporting from PSC’s Ken Chiacchia here.
Header image: examples of stamps from the repository. Image courtesy of PSC.