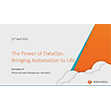
COVID-Driven Cloud Surge Takes a Toll on the Data
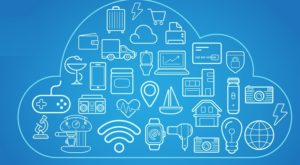
(CkyBe/Shutterstock)
The stepped-up pace of migration to the cloud over the past 18 months wasn’t exactly forced, but neither was it completely voluntary. For many companies, accelerating digital transformation during COVID was a matter of survival. But the journey hasn’t necessarily been free of drama, particularly as it relates to the data.
The cloud surge during COVID is well documented at this point. Work-from-home mandates forced employees across the board out of office buildings and into spare bedrooms, living rooms, and kitchens around the world. That included the IT workers who would normally be asked to configure new compute infrastructure. Instead of standing up servers, they looked to the public and private clouds to run their infrastructure for them.
There is data to back this up. AWS quarterly revenue since the start of COVID has been on an accelerated pace, reaching nearly $15 billion in the most recent quarter. Microsoft reported 51% growth in Azure for the for the quarter ended June 30, to $7.8 billion for its “intelligent cloud” segment.
Cloud spending was slightly to significantly higher at 90% of businesses, according to Flexera’s State of the Cloud Report 2021. A June 2021 survey Devo Technology found 81% of business leaders say COVID accelerated their cloud timelines and plans.
“It could not be more clear from our conversations with these companies that cloud considerations are no longer a project-based decision, but an ‘all-in’ business strategy,” said Jon Oltsik, a senior principal analyst and fellow at ESG Research, which conducted the survey on behalf of Devo Technology.
And of course, there is the comment that Microsoft CEO Satya Nadella made during a conference call with analysts following Microsoft’s quarter results in the spring of 2020. “We’ve seen two years’ worth of digital transformation in two months,” Nadella said.
Zoomin’ to the Cloud
COVID has laid bare many of the problems with data that have been simmering under the surface. We have charted the data quality concerns expressed by public health agencies and healthcare groups in their response to COVID, including tests, trace and trace methodologies, and rates of infections, hospitalizations, and deaths.
As it turns out, private companies are facing a similar raft of data management challenges during COVID, and much of it can be traced to the accelerated march to the cloud during the pandemic.
From the proliferation of Zoom and Teams meetings, to the adoption of Snowflake and Redshift data warehouses, to VPNs and online gaming, 2020 was a year unlike any other. COVID demanded that companies adapt to the new reality, or go out of business. That adaption, in many respects, meant moving applications to the cloud, or building new ones there.
Krishna Tammana, the CTO of Talend, says companies accelerated their existing cloud plans during COVID simply as a means to stay in business.
“Everybody has these plans to move to the cloud, but this pandemic just forced them to move faster,” he says. “In some cases, it was not even an option. So we just move right now, or else some of what we were doing would stop.”
Crouching Cloud, Hidden Data
Unfortunately, the cloud migrations did not always go as planned. As you would expect during a hasty transition, some elements of the move went poorly. In many companies, data management was an afterthought during the COVID-induced move to the cloud.
How did we get here? Tammana says a combination of factors led to some data management issues with the rapid cloud transition. The first factor is just knowing what data exists, and where it’s located.
“In some circles, we call it the dark data, because it’s just data that exists, but nobody knows it exists,” Tammana says. “The information that did exist is kind of locked in some corner.”
The bigger the company, the bigger the data issues become. A smaller company with fewer computer systems and data standards won’t have trouble getting a clear view of the facts. But a public company composed of multiple entities may need to go through data transformation exercises to ensure that they are comparing apples with apples, and not oranges or bananas.
“If you have two subsidiaries, do the definitions of a customer and the customer ID meet? Do they match? Does your definition of revenue from this system and that system match, or do we need to go through some transformation?” Tammana says.
“The third issue is now we’re collecting more data in a rush,” he continues. “You combine those things three things, and you have the perfect storm of creating more data, but not necessarily being able to put it all to work the way you would want to.”
Revenge of Hadoop Lake
Tammana sees some similarities between what is happening today in the cloud, and what the data analytics industry just went through with respect to the adoption of Hadoop data lakes. It comes down to how companies approach their data integration strategies–or whether they abandon strategy altogether in their rush to get somewhere fast.
There are two broad choices companies can make with regards to their data integration strategy: ETL or ELT. In some cases, companies can use both; they are not mutually exclusive, as the pace of data generation, the needs for timely analysis, and the willingness to accept the costs incurred by upfront data transformation vary from case to case.
With the traditional ETL process, companies opt to transform (i.e. clean, standardize, and normalize) the data before it lands in the data warehouse or lake, where data analysts and data scientists can use it to serve dashboards and look for trends. This is largely synonymous with the “schema on write” approach that was in place before the Hadoop era began in the 2011-2013 timeframe.
With the newer ELT-based approach, companies first land the data into the data lake or data warehouse, and transform the data later, as the needs arise. This is the “schema on read” approach that Hadoop made popular with largely unstructured and semi-structured data, and which continues today with many data lakes and data warehouse implementations.
The lessons learned from Hadoop loom large, Tammana says. But is anybody paying attention?
“ELT tends to be when people dump the data in the lake and then they want to process it later,” he says. “But they’re very wary of the problems that we run into as an industry with Hadoop, for example. Everybody thew a lot of data into Hadoop and everybody lost track of what that data meant, how to use it. And of course, that compounded the fact that you needed Java developer to put data put that use, it made it impossible to get value out of that.”
Our tools are better today, and users no longer need Java developers to get at the data. Straight SQL will suffice, and even Python is supported. Citizen data scientists and embedded analytics is democratizing access to data. But that doesn’t address the fact that data is piling up in cloud data lakes and warehouses, and folks may not be remembering to keep track of it all.
“The combination of not knowing what data is there, and needing some technical skills to get value out of that, created a problem for Hadoop,” Tammana says. “But the first problem of throwing all the data in, and then not knowing what to do with it, still exists in this world, even if you’re using Snowflake.
Tammana is optimistic that people learned from the Hadoop experience, and are being more careful with their data. “But I have no doubt that there is a problem, to some degree,” he says.
Related Items:
Still Wanted: (Much) Better COVID Data
The Big Cloud Data Boom Gets Even Bigger, Thanks to COVID-19