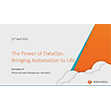
Rethinking Education in an AI-First World
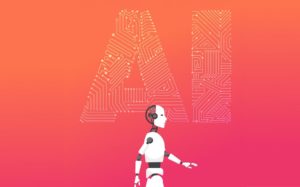
(Bluehousestudio/Shutterstock)
Universities have been ramping up their data science education initiatives ever since 2012, when Tom Davenport and DJ Patil declared data scientist “the sexiest job of the 21st century” in the Harvard Business Review. While there’s still a shortage of data scientists coming out of universities, the coming AI revolution necessitates a fundamental transformation of education–not only for the data scientists building AI apps, but for the rest of us living with them.
According to the website Data Science Programs, there are more than 500 universities across the United States with data science degree programs. All told, there are more than 980 individual programs, with Master of Data Science being the most popular. This number has increased substantially in recent years, according to past numbers shared by this website.
While the supply of data scientists emerging from universities is up, strong demand for data scientists at American companies continues to outstrip supply, according to Martial Hebert, the dean of the School of Computer Science at Carnegie Mellon University. “Yes, we stepped up [supply], and no we’re not meeting [demand],” Hebert said. “We’re off by millions, basically.”
CMU has been at the forefront in trying to ramp up supply of data scientists and AI experts by expanding its data science degree programs. In fact, CMU was the first university in the U.S. to offer a Bachelor’s of Science degree in AI, and is still is the only school offering such program across the country, said Hebert, who joined CMU in 1984 as a researcher for computer vision and autonomous systems.
“We’ve had a couple of cohorts now, but it’s still very, very small compared to the kind of needs that we have,” Hebert told Datanami in a recent interview. “And part of the reason why there are those needs, in the case of AI, [is it] goes far beyond what we think of as the tech industry. It goes into every single segment of activity.”
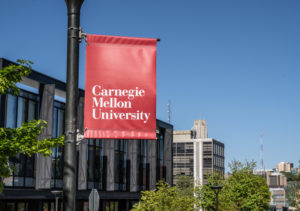
Carnegie Mellon launched the nation’s first Bachelor’s program for data science (Amy Lutz/Shutterstock)
Finance isn’t just finance anymore–it’s finance and AI. Political science isn’t just political science anymore–it’s political science and AI. From healthcare to agriculture, the military to mining, many academic and human disciplines are being refabricated, to some extent, around AI.
“The vision of the field is that we’re going to see more and more of the development of the new disciplines that are AI-and,” Hebert said. “For example, there’s a lot of development in automated science and AI for scientific discovery, which is not just taking tools from AI over there, and just applying them to some scientist stuff here. It’s actually bringing them together to create a completely new discipline.”
These new disciplines are starting to take root. CMU has a new graduate program to enable students to apply AI broadly, called the Master of Science in Artificial Intelligence and Innovation. It is also working on a new degree program at the Heinz School of Public Policy that will explore the use of AI in public policy, including topics surrounding bias and equity, Hebert said.
“So it’s not just, you learn those tools and then you can just apply them to various applications, essentially building those disciplines,” he said. “We will have degrees in all of those combinations, basically.”
Students entering these graduate-level programs will be expected to have a solid technical understanding of data science, just like the students pursuing a traditional data science degree, as typically taught in a computer science department. But the programs will go beyond that core understanding in data science to AI to create an environment where the students essentially create new tools and technologies for the specific discipline, Hebert said.
“For example, when you look at AI for public policy, there are things that are specific to the public policy environment, namely, how they affect people having to do with social sciences, and things like this, that need to be taken into account in the design of those AI [systems],” he said. “And that requires new techniques, new approaches, new disciplines. So that’s where things get really interesting, when it’s not just taking a startup course in AI, and then having maybe a project course for the application. It actually requires those new techniques.”
Not every program will benefit from a dose of AI. It’s tough to see how a study of English literature, for example, or Renaissance Art, will benefit from AI. On the other hand, it would seem there are clear applications of AI in other aspects of the liberal arts, such as journalism or music, for example.
But Hebert’s vision goes beyond creating degree programs that are a hybrid of traditional programs and AI. He wants to see AI topics taught broadly at all levels of school, including K-12. As AI plays a bigger role in society, having citizens who understand how it fits in and can work with AI will be beneficial to the broader community.
“I think one thing that is really important is to understand the expectations [of AI],” he said. “In other words, this is what it does, this is what it does not do. This is what it cannot do. This is what you need to be able to access an AI system. Here are the questions you need to ask.”
It’s important for students to learn about AI’s capabilities and limitations so they can work around them, Hebert said. “So if you don’t have the technical background, if you don’t understand the details of the system, at least you know how to evaluate them, how to compare them, how to anticipate basically where you meet those issues, and so forth,” he said.
People often make poor assumptions about what so-called “artificial intelligence” programs are capable of. In fact, some leaders in the field, including Microsoft chief technology officer Kevin Scott, are questioning whether AI is even a good name for what the industry is creating.
“As soon as you utter the words ‘artificial intelligence’ to an intelligent human being,” Scott told the Wall Street Journal last week, “they start making associations about their own intelligence, about what’s easy and hard for them, and they superimpose those expectations onto these software systems.”
That is not to take away from the impact that AI–as we understand it today–is expected to have on this world. The collective surge in capabilities around computer vision, natural language processing (NLP), traditional machine learning, and advanced analytics are unleashing a tidal wave of data-based automation and innovation on the world (dare we call it a Datanami).
Three years ago, McKinsey pegged the impact of AI at $13 trillion by 2030. After watching digital transformation accelerate due to COVID-19, that number may actually be low. That makes it all the more important to understand the fundamental limitations of AI technology as it exists today.
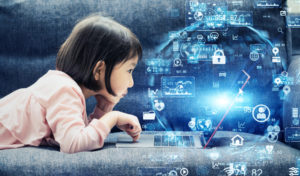
Introducing younger students to AI concepts is seen by some as a priority (metamorworks/Shutterstock)
For example, even with the biggest, most powerful neural network model on the planet–“the most powerful architecture you can think of,” Hebert said–you can’t rely on predictions generated from data that falls outside the range of the data that the model was trained on. These are fundamental limitations that will not change, he said. Ethical questions present more of a moving target. That’s one of the reasons why all of CMU’s programs include elements about the ethical implications of AI.
Some of these issues are so important that the should be taught before students arrive at university, according to Hebert. That’s why the university is working with the public schools in the Pittsburgh, Pennsylvania area to help with teaching these core AI concepts.
“It’s difficult, by the way, because there’s always a lot of stuff that students need to learn in high school, so we have to be careful how we inject those things,” Herbert said. “But some of the principles, some of the concepts, understanding again the limitations and capabilities–those are things that that can be introduced.”
Related Items:
Why Data Scientists and ML Engineers Shouldn’t Worry About the Rise of AutoM
Why Data Science Is Still a Top Job
Data Science Back to School: Accelerate Your Education