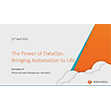
What’s Holding Us Back Now? ‘It’s the Data, Stupid’
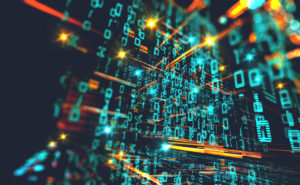
(carlos castilla/Shutterstock)
The good news is the barrier to entry for data science has lowered dramatically in recent years, thanks to better data science software and cloud computing. The bad news is that getting ahead with big data requires–you guessed it–access to more and better data.
In some ways, the “data is the differentiator” story has not changed. Even when organizations were struggling to get their Hadoop environments up and running 10 years ago and get all of the various software products working together, the goal was always to build a platform to do something creative, fun, or profitable with data.
The difference today is that a lot of the other stuff that previously got in the way of leveraging data–namely, assembling the hardware and software stacks needed to facilitate advanced analytics and training machine learning models–has gotten a lot better.
Thanks to the nearly unlimited compute resources available on public cloud platforms, and the “glut of innovation” that Gartner has identified data science and machine learning applications, the old big data barriers have been torn down. These are heady days for data science and big data practitioners, to be sure.
So now that ready-made data science and advanced analytic platforms that can crunch huge amounts of data are available at our beck and call, what’s holding us back from getting down to business and doing great things with the data? To paraphrase a political consultant, “It’s the data, stupid.”
The amount of data in the world continues to grow at a rapid pace. According to IDC, there was 64.2 zettabytes of data created or replicated in 2020. Over the next five years, IDC projects data to increase at a 23% annual compound growth rate. So there is plenty of data to be had. The big question is how will that data be distributed, and which companies will take advantage of it.
One vendor that’s aiming to get more and better data into the hands of data science teams is Narrative. The New York City company hosts a streaming data platform that connects data buyers with data sellers, enabling companies of all sizes to swing above their (data) weight.
“The tech is there for smaller companies to compete” with the FAANGS of the world, says Nick Jordan, the CEO and founder of Narrative. (FAANG, of course, refers to the tech giants like Facebook, Amazon, Apple, Netflix, and Google [plus Microsoft].) “In order to really compete though, they’ve got to figure out a way to have some semblance of the scale of data that the FAANGs have.”
Narrative’s platform helps automate much of the integration, security, and regulatory work that arises when working in a third-party data marketplace. The company has an equal balance of data buyers and data sellers, Jordan says. It turns out that, when a company starts the process to begin buying third-party data, they often come the realization that their data has value to others, too.
“Our job is to make it so someone who isn’t steeped in this type of technology can do this, and it looks like it’s magic, it’s no longer hard,” Jordan says. “Data used to be the purview of the nerds. And that was great. But to really realize the full value, it needs to be used everywhere in the organization, which means people who don’t have degrees in statistics are going to have to be able to figure it out.”
As the technological barriers to advanced analytics and AI begin to fall, companies are ramping up their activity levels. For instance, the average number of data sources used by organizations is 27, with a high of 90, according to a recent study by Precisely. About 75% of the chief data officers (CDOs) surveyed said that dealing with multiple data sources and complex data formats is “very” or “quite challenging.”
Similarly, a recent study by Ascend.io found that nearly 80% of data professionals say their infrastructure and systems are able to scale to meet their increased data volume processing needs. The survey found that 96% of data professionals are at or over capacity. In other words, the bottleneck has shifted to personnel.
Dealing with the data and all that entails (security, backups, regulations, governance, integration, transformed, prepped) as opposed to creating machine learning algorithms or building AI models, is increasingly where the bottleneck exists.
“Predictive models are almost commoditized, off the shelf,” says Maor Shlomo, the CEO of alternative data platform Explorium, which last month unveiled a $75 million funding round. “Data science and advanced analytics have become way more accessible and easier to do–easier to create predictive models, easier to create algorithms.”
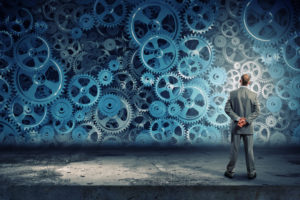
Having the right data, and the skilled professionals to utilize it, are the bottlenecks to AI success (ESB-Professional./Shutterstock)
With a lot of the infrastructure built and data science work commoditized, the big data game has shifted. Today, it’s about connecting organizations with the right data set that can make an impact. For Explorium, it’s hoping to get ahead by providing a solution that can automatically make suggestions for what third-party data sets to bear for a customer based on their existing data.
“A lot of the analysis in Explorium starts with the customer data,” Shlomo says. “We bring in data bout leads, about businesses, people, customers, locations and stuff like that. Then you enjoy the platform as a way to do automate matching and joining of data and discovery of correlations and variables.”
While data is increasingly the differentiator, there can be too much of a good thing. Reducing the universe of data to what can be impactful for a given customer is how Explorium hopes to help its customer and, in turn, grow its business.
“If I go to a customer and say ‘here’s a gazillion different variables, have fun with that,’ that would only make the problem for the customer worse,” Shlomo says. “Because now they have to search for the first-party and third-party data and understand how the data might connect to each other and what variables can you extract from that and how the data is actually inmpactful for the specific predictive model they’re trying to build.”
The nature of big data is changing. The volumes and variety are bigger today than they were 10 years ago, of course. Thanks to technological advances in hardware and software, the biggest barrier to data success is the data itself.
Related Items:
Data Pros Are Maxed Out: Survey
Data Integrity a Major Concern, Precisely Says
A ‘Glut’ of Innovation Spotted in Data Science and ML Platforms