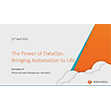
Researchers Use Deep Learning to Plow Through NASA Snow Radar Data
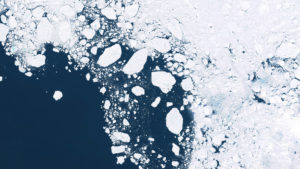
From 2009 through 2019, NASA’s Operation IceBridge sent out observation flights over the Arctic, Antarctic, and Alaska. Those 11 years of flights produced detailed data on the dimensions and fluxes of snow, sea ice, glaciers, and ice sheets thanks to a medley of radar, lasers, cameras, gravimeters, magnetometers, and other instruments. Now, researchers at the University of Maryland, Baltimore County (UMBC) are using AI to speed analysis of that data deluge.
Specifically, the researchers set out to process the radar data gathered from polar regions – data that can, in the absence of advanced analytics, take months or years to meaningfully parse. “Radar big data is very difficult to mine and understand just by using manual techniques,” said Maryam Rahnemoonfar, associate professor of information systems lead author of the paper, in an interview with UMBC’s Morgan Zepp.
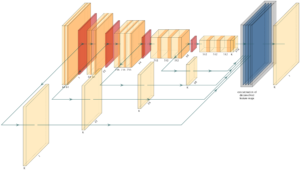
A multi-scale convolutional neural network with convolution layers (orange), pooling layers (red), and a fused layer (blue). Image courtesy of the authors.
To accelerate that process, the researchers utilized a multi-scale deep learning algorithm that traced boundaries of snow layers, allowing analysis of snow-covered areas. The algorithm has helped the team cut through the noisy data, which inhibits techniques like transfer learning. Neural networks trained to detect those snow boundaries on optical imagery experienced only limited success when trying to detect the same boundaries in radar imagery. Then, the researchers found that if the algorithms were trained on the radar imagery from the start, they yielded “far better results.”
“Deep neural networks owe their success to the availability of massive labeled data,” the team wrote. “However, in many real-world problems, even when a large dataset is available, deep learning methods have shown less success, due to causes such as lack of a large labeled dataset, presence of noise in the data or missing data.”
While the algorithm is not yet fully trained (data from more sensors across more locations need to be incorporated), process automation has already begun. The researchers are also tackling the issue of generating sufficient training data in order to scale up the model.
“[Training from scratch] requires annotated data provided by the domain experts,” they wrote. “One way to avoid this would be to generate synthetic data. Although the synthetic data used for training in this work only loosely match the actual snow radar, the results indicate that synthetic data could be successfully used for training. Future work should explore training with synthetic data which matches the noise and signal statistics of the actual snow radar data.”
“In the future, we plan to combine AI and physical models to expand the simulated dataset and therefore better train our network,” they added. “We also plan to develop [an] advanced noise removal technique based on deep learning.”
To read the full paper, which was published in the Journal of Glaciology, click here.