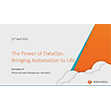
Building Monster Mash-Ups: How Data as a Service Can Crystallize Analytics
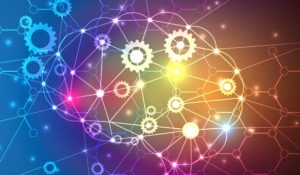
(vs148/Shutterstock)
The chances are that if you’re reading this you have heard the phrase “Data is the new oil,” coined by Clive Humby, the British mathematician and architect of early supermarket loyalty rewards schemes. Although it’s become a cliché, it’s an even more appropriate phrase than you might think.
Why? Because oil is toxic and dangerous in its raw form; it needs to be refined before it becomes valuable and the same is true of data. Fail to control it, de-duplicate it, cleanse it or analyze it in context will only serve as the basis of bad decisions. What’s needed is data that’s deployed with a purpose, but do all those things and you have a platform for Data as a Service (DaaS).
If data is the new oil and the ‘as a service’ model is taking over not just technology but the Subscription Economy, where we rent rather than purchase goods and services, then combining those two phenomena in DaaS should really be something — and it is. DaaS is the ultimate in analytics, a constant spring of data that’s available on demand from multiple internal and external sources in the cloud.
DaaS is usually embodied in mash-ups and composite apps with metadata and policy management adding extra power and control. You might compare it to the movie Minority Report, where data is visualized in real time, bringing to life dashboards that benefit their owners by anticipating events rather than merely reacting to them after the event. This is the new wave of event-driven architectures where historical events automatically trigger actions based on known trends that help us forecast what is going to happen next.
But DaaS is also underwritten by access to multiple sources, both internal and external. Think of the example of utilities. A water company might receive information about a leakage from sentiment analysis of social media. Twitter complaints help it to identify where the leakage has occurred and, by triangulation, how extensive the problem is. The utility can then address the issue by using geofencing to establish which engineers are in the nearest location to stop the leakage.
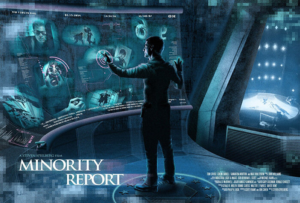
Real-time predictive mashups were a core feature of the 2002 film Minority Report (image courtesy Alternative Movie Posters)
To power DaaS, the way forward for companies must lie in building partner ecosystems to access a wide range of data and spark faster decision-making. An Indy 500 team will have a huge array of data from weather information and track simulations to hundreds of on-car sensors that support performance optimization while mitigating safety risks. A hotel chain will try for hyper personalization by understanding individual preferences but also tallying those with larger data sets of customers with similar demographics to best-guess associated preferences.
Companies naturally seek out useful data but governance and compliance guardrails are needed to avoid breaking data privacy rules or spooking customers. Data virtualization, where a modern data layer removes bottlenecks, will help to ensure that data can be accessed without impacting security by moving it elsewhere.
Another challenge is finding the data scientists and chief data officers to deploy DaaS. But the good news is that we are seeing more graduates emerge, more data mentoring, communities of practice, data mastering and established best practices and case studies to lean on.
By combining data and bringing it to people who need it on-demand and in close to real-time, we’re able to answer questions very quickly. When is the traffic worst near where I live? Where are COVID-19 cases worse and who is affected most – men or women, the young or the old? How much crime is there in my neighbourhood and what types of crimes are being committed?
Pretty much any question can be answered if you have the data. Data drives insights, those insights drive smart decisions, and those smart decisions build a platform for commercial and operational success. By leveraging APIs we can create the ‘monster mash-ups’ that we need, but in order to do that, we need smart workflows and we need to avoid data silos, where the statistics don’t talk to other statistics, or the information is dud or unreliable. DaaS drives revenue, profitability, strategy and efficiencies, so every company needs to pursue it, becoming data-infused, deploying the tools and data scientists who can interpret data and explain it in a way that’s compelling even for non-specialists.
About the author: Pedro Diaz Ochagavia is the vice president of TIBCO’s Central and West Americas regions. He works with numerous teams across the Americas, instilling a culture of customer excellence. With 20 years of experience in the software industry, Pedro is well respected for his views on the ever-changing SaaS and digital transformation markets. Prior to joining TIBCO, Pedro held senior positions at BMC in Europe before relocating to North America to run BMC’s Latin and West Americas regions. Pedro’s early career was honed at Hewlett-Packard in Europe. Pedro holds MS degrees in Information Security from Madrid University and Engineering in Information Technology from ICADE-ICAI University, as well as a BS in Computer Science from ICADE-ICAI University in Madrid.
Related Items:
Go Fast and Win: The Big Data Analytics of F1 Racing
Self-Service Analytics Seen Overtaking Data Scientists
How to Build a Data-Driven Culture