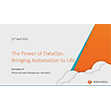
Google Enables Machine Learning for Gunshot Recognition in the Rainforest
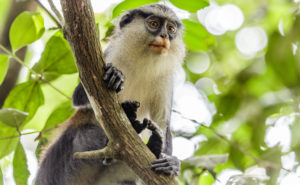
The World Wildlife Fund (WWF) estimates that poaching is the root of a $20 billion-a-year industry. This thriving, illegal practice is largely possible because enforcement of poaching laws is so difficult: personnel must monitor enormous swaths of land for a handful of rare animals and sneaky humans – and they must identify infractions quickly enough to make a meaningful difference. For the past three years, the Zoological Society of London (ZSL) has been partnered with Google Cloud to use machine learning to streamline these processes and protect endangered species. Now, ZSL and Google Cloud are highlighting a new tool on that front: acoustic data monitoring.
“The analysis of acoustic (sound) data to support wildlife conservation is one of the major lines of work at ZSL’s monitoring and technology programme,” wrote Omer Mahmood, a head of customer engineering for Google Cloud, UK and Ireland. “Compared to camera traps that are limited to detection at close range, acoustic sensors can detect events up to 1 kilometre (about half a mile) away. This has the potential to enable conservationists to track wildlife behaviour and threats over much greater areas.”
A few years ago, ZSL collected a month’s worth of acoustic data from 69 recording devices placed around the Dja Faunal Reserve in Cameroon. UNESCO describes the Dja Reserve as “one of the largest and best-protected rainforests in Africa,” with a whopping 90% of its area remaining undisturbed and exceptional biodiversity: the reserve is home to more than 100 mammal species, including forest elephants, African gray parrots, leopards and at least 14 primate species.
The resulting 350GB of audio data (cumulatively, 267 days of audio) proved overwhelming for ZSL. Luckily, machine learning was well-suited for ZSL’s primary point of interest: gunshots.
Google Cloud worked with ZSL to deploy a Google-developed machine learning model, YAMNet, that was trained on millions of YouTube videos to classify more than 500 types of audio events.
Within 15 minutes, YAMNet had combed the whole dataset, identifying 1,746 gunshots with confidence.
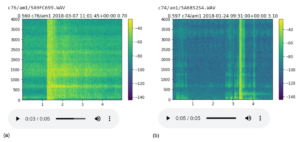
An example of a true positive versus a false positive. On the left, a gunshot; on the right, a hammer strike. Image courtesy of Google Cloud.
Using the original audio files, the possible gunshots, and the model’s confidence levels for each possible gunshot, the team built a visualization tool that allowed them to load each sample, visualize it, and listen to audio samples, sorting all the results by confidence level.
In the end, the team found three unique gunshots from the possible results – but more importantly, a task that would have taken the same researchers months took them just a few hours under the new workflow.
“In this short one-month study that only covered a portion of the reserve, the research team were able to contribute new insights to the human threats to species in the Dja Reserve,” Mahmood wrote. “Past data suggests gunshots are more likely to take place at night to evade ranger detection, but using ecoacoustics alone, ZSL provided evidence of illegal hunting occurring during the day.”
The team hopes that the findings can be used to create more powerful tools, like on-device classification, cheaper monitoring, and – eventually – real-time alerts.
This is far from the first time AI has been used in the quest to pinch poachers. Back in 2019, Peace Parks Foundation worked with Microsoft to use AI to comb through camera imagery.